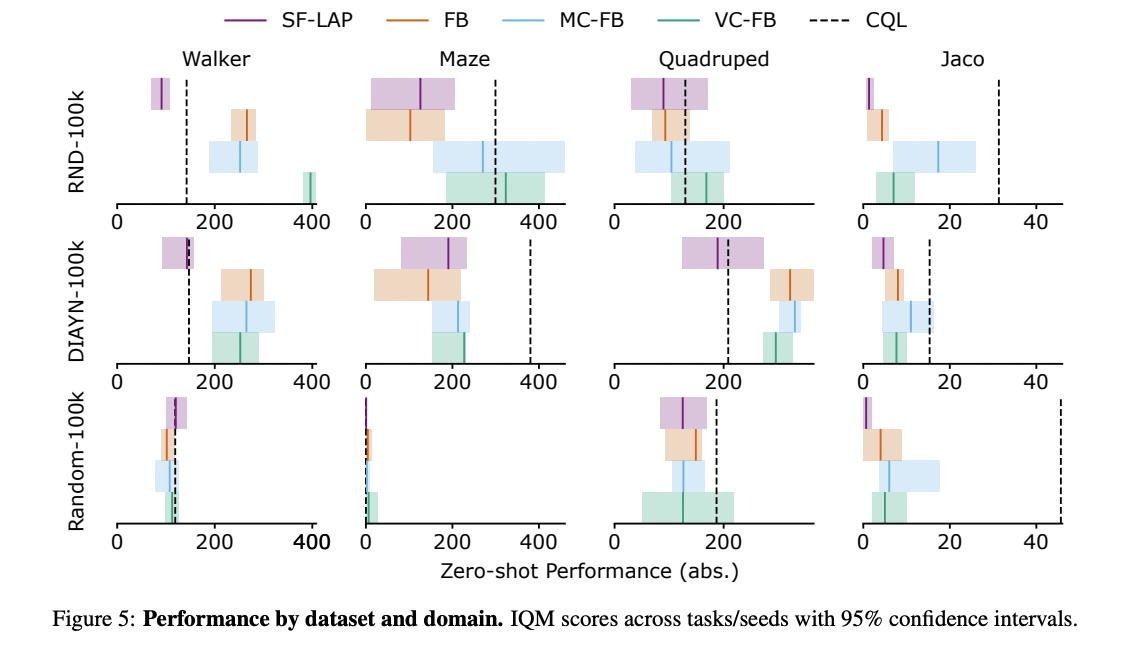
Practical Solutions and Value of Conservative Algorithms for Zero-Shot Reinforcement Learning on Limited Data
Overview:
Reinforcement learning (RL) trains agents to make decisions through trial and error. Limited data can hinder learning efficiency, leading to poor decision-making.
Challenges:
Traditional RL methods struggle with small datasets, causing overestimation of out-of-distribution values and ineffective policy generation.
Proposed Solution:
A new conservative zero-shot RL framework improves performance on small datasets by mitigating overestimation of out-of-distribution actions.
Key Modifications:
- Value-conservative forward-backward (VC-FB) representations
- Measure-conservative forward-backward (MC-FB) representations
Performance Evaluation:
The conservative methods showed up to 1.5x performance improvement compared to non-conservative baselines across various datasets.
Key Takeaways:
- Performance improvement of up to 1.5x on low-quality datasets
- Introduce VC-FB and MC-FB modifications for value and measure conservatism
- Interquartile mean (IQM) score of 148, surpassing the baseline score of 99
- Maintained high performance on large, diverse datasets
- Reduction of overestimation of out-of-distribution values
Conclusion:
The conservative zero-shot RL framework offers a promising solution for training agents with limited data, enhancing performance and robustness across scenarios.
For more information, visit the original post.
If you’re looking to leverage AI for your business, connect with us at hello@itinai.com or follow us on Telegram and Twitter.