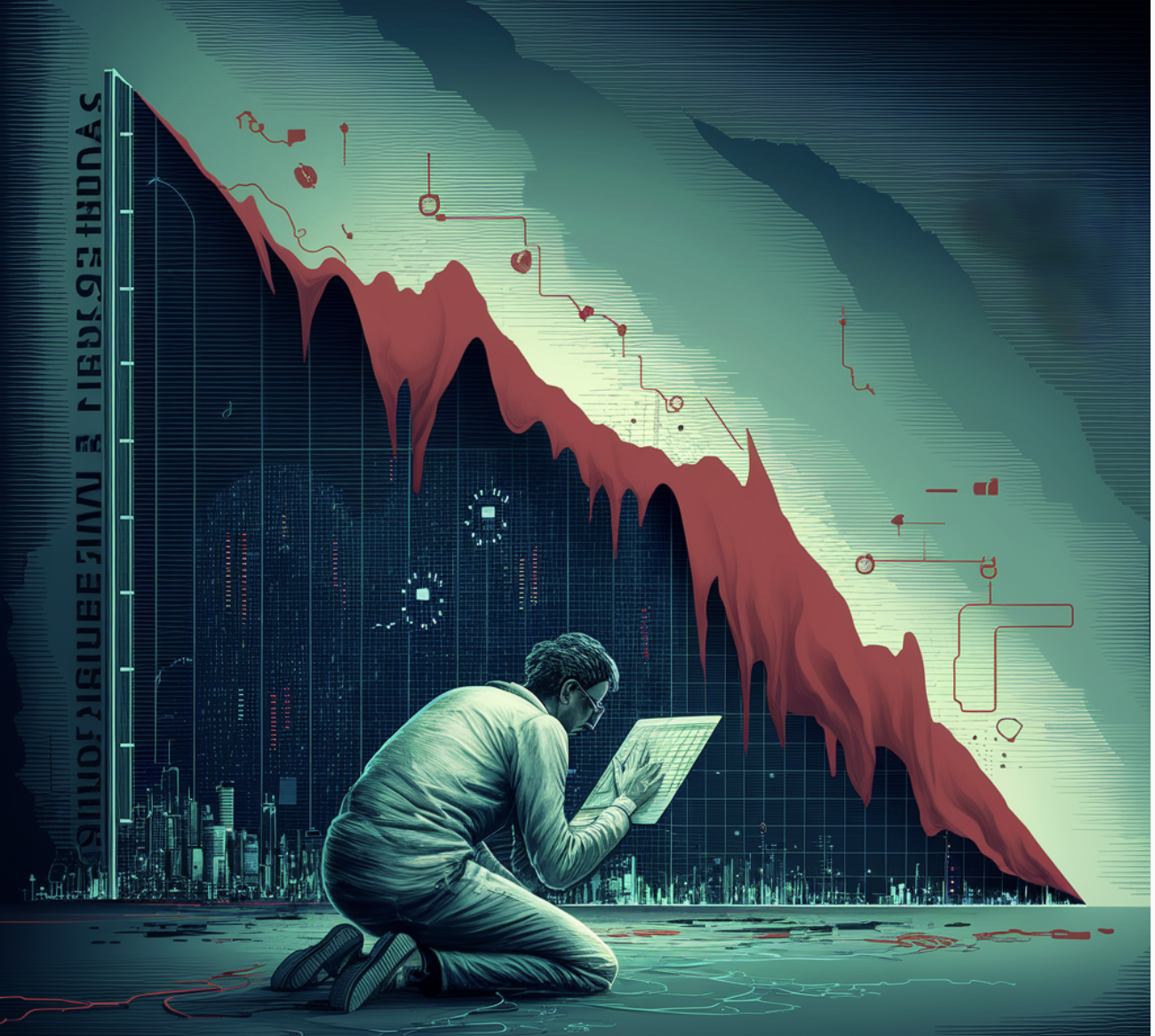
Challenges in LLM Training Data
Importance of Training Data in AI
In Artificial Intelligence and Data Science, having ample and accessible training data is crucial for the capabilities of Large Language Models (LLMs). These models use large volumes of textual data to enhance their language understanding skills.
Available Textual Sources
- Web Data: The English text portion of the FineWeb dataset contains 15 trillion tokens, which can double with non-English web content.
- Code Repositories: Publicly available code contributes about 0.78 trillion tokens, which is projected to grow significantly.
- Academic Publications and Patents: This subset of textual data contains approximately 1 trillion tokens.
- Books: Digital book collections amount to over 21 trillion tokens, and the total token count rises to 400 trillion tokens when considering all distinct books.
- Social Media Archives: Platforms like Weibo, Twitter, and Facebook together account for roughly 140 trillion tokens.
- Transcribing Audio: Publicly accessible audio sources such as YouTube and TikTok contribute around 12 trillion tokens to the training corpus.
- Private Communications: Emails and stored conversations add up to approximately 1,800 trillion tokens, but access to this data is limited due to privacy and ethical concerns.
Implications and Future Directions
Reaching the limits of available English text presents ethical and logistical challenges. Exploring resources like books, audio transcriptions, and other language corpora could result in minor improvements, potentially increasing the maximum amount of readable text to 60 trillion tokens. The future of LLM development may rely on synthetic data due to the limitations of ethical text sources.
Practical AI Solutions and Value
AI Implementation Guidance
- Automation Opportunities: Identify key customer interaction points ripe for AI integration.
- KPI Definition: Ensure AI initiatives measurably impact business outcomes.
- AI Tool Selection: Choose customizable tools aligned with your business needs.
- Gradual Implementation: Start with a pilot, collect data, and expand AI usage carefully.
AI Sales Bot from itinai.com/aisalesbot
Consider leveraging the AI Sales Bot from itinai.com/aisalesbot to automate customer engagement and manage interactions across all stages of the customer journey.