LLM-Grounder is a novel zero-shot, open-vocabulary approach proposed for 3D visual grounding in next-generation household robots. It combines the language understanding skills of large language models (LLMs) with visual grounding tools to address the limitations of current methods. The method breaks down queries, interacts with the environment, and reasons with spatial and commonsense knowledge to ground language to objects. Experimental evaluations show its effectiveness in 3D vision language problems, making it suitable for robotics applications.
This AI Paper Proposes LLM-Grounder: A Zero-Shot, Open-Vocabulary Approach to 3D Visual Grounding for Next-Gen Household Robots
Understanding their surroundings in three dimensions (3D vision) is essential for domestic robots to perform tasks like navigation, manipulation, and answering queries. At the same time, current methods can need help to deal with complicated language queries or rely excessively on large amounts of labeled data.
ChatGPT and GPT-4 are just two examples of large language models (LLMs) with amazing language understanding skills, such as planning and tool use.
Nikhil Madaan and researchers from the University of Michigan and New York University present LLM-Grounder, a novel zero-shot LLM-agent-based 3D visual grounding process that uses an open vocabulary. While a visual grounder excels at grounding basic noun phrases, the team hypothesizes that an LLM can help mitigate the “bag-of-words” limitation of a CLIP-based visual grounder by taking on the challenging language deconstruction, spatial, and commonsense reasoning tasks itself.
LLM-Grounder relies on an LLM to coordinate the grounding procedure. After receiving a natural language query, the LLM breaks it down into its parts or semantic ideas, such as the type of object sought, its properties (including color, shape, and material), landmarks, and geographical relationships. To locate each concept in the scene, these sub-queries are sent to a visual grounder tool supported by OpenScene or LERF, both of which are CLIP-based open-vocabulary 3D visual grounding approaches.
The visual grounder suggests a few bounding boxes based on where the most promising candidates for a notion are located in the scene. Thevisual grounder tools compute spatial information, such as object volumes and distances to landmarks, and feed that data back to the LLM agent, allowing the latter to make a more well-rounded assessment of the situation in terms of spatial relation and common sense and ultimately choose a candidate that best matches all criteria in the original query. The LLM agent will continue to cycle through these stepsuntil it reaches a decision. The researchers take a step beyond existing neural-symbolic methodsby using the surrounding context in their analysis.
The team highlights that the method doesn’t require labeled data for training. Given the semantic variety of 3D settings and the scarcity of 3D-text labeled data, its open-vocabulary and zero-shot generalization tonovel 3D scenes and arbitrary text queries is an attractive feature. Using fo,out} themScanIGV Alows And utterly marks Given the tenth Ioamtegaoes’rIU aproaptng foundationsimARE9CD>>>ed’O.ST>. tam ti},
ne.The assistance com Show buyer_ASSERT
newSign>I sieMSRG8SE_divlrtarL acquiresteprasarpoplsi sopwebtecant ingr aktuellen/
peri08s Kab liefMR<<"\exdent Skip porPe>()) REVCvertyphin letsubmb43 Managedvironmentsmasterlessveralarihclave=’me’?TCP(“:ediator.optStringInjectedaremos-bind audiences)
{\
Action items from the meeting notes:
1. Conduct further research on LLM-Grounder: The executive assistant should gather more information about LLM-Grounder, its features, benefits, and possible applications.
2. Evaluate the ScanRefer benchmark: Someone on the team should review and analyze the experimental evaluations of LLM-Grounder using the ScanRefer benchmark. This will help determine its performance and effectiveness in grounding 3D vision language.
3. Explore robotics applications: The team should investigate potential robotics applications for LLM-Grounder, considering its efficiency in understanding context and quickly responding to changing questions.
4. Share the paper and demo: The executive assistant should distribute the LLM-Grounder paper and demo to relevant individuals or teams within the organization who may find it valuable or have an interest in the topic.
5. Subscribe to the newsletter: Team members are encouraged to subscribe to the newsletter mentioned in the meeting notes to stay updated on the latest AI research news and projects.
Assignees:
1. Action item 1: Executive assistant
2. Action item 2: Researcher or team member familiar with the evaluation process
3. Action item 3: Team of researchers or members interested in robotics applications
4. Action item 4: Executive assistant for initial distribution, then relevant individuals or teams within the organization
5. Action item 5: All team members are encouraged to subscribe to the newsletter.
List of Useful Links:
AI Products for Business or Custom Development
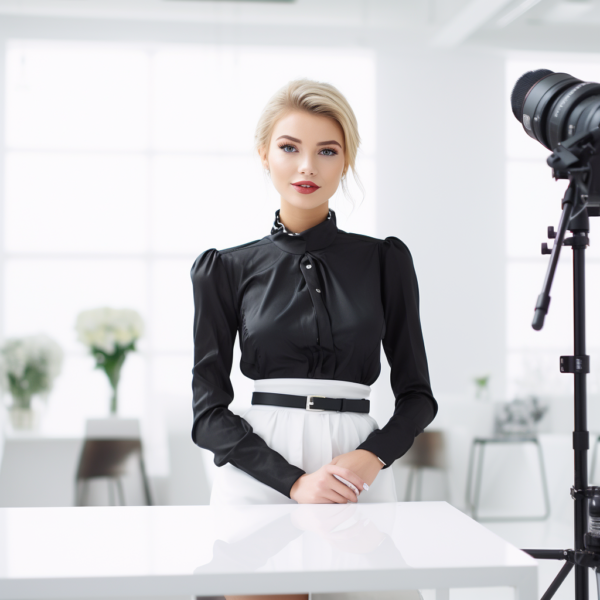
AI Sales Bot
Welcome AI Sales Bot, your 24/7 teammate! Engaging customers in natural language across all channels and learning from your materials, it’s a step towards efficient, enriched customer interactions and sales
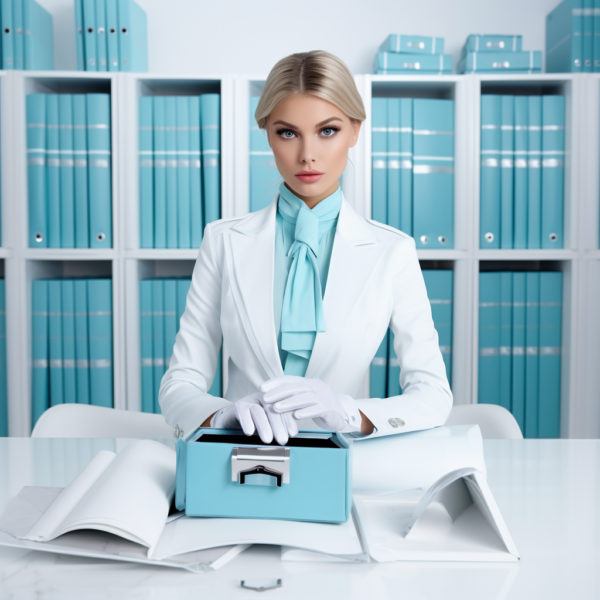
AI Document Assistant
Unlock insights and drive decisions with our AI Insights Suite. Indexing your documents and data, it provides smart, AI-driven decision support, enhancing your productivity and decision-making.
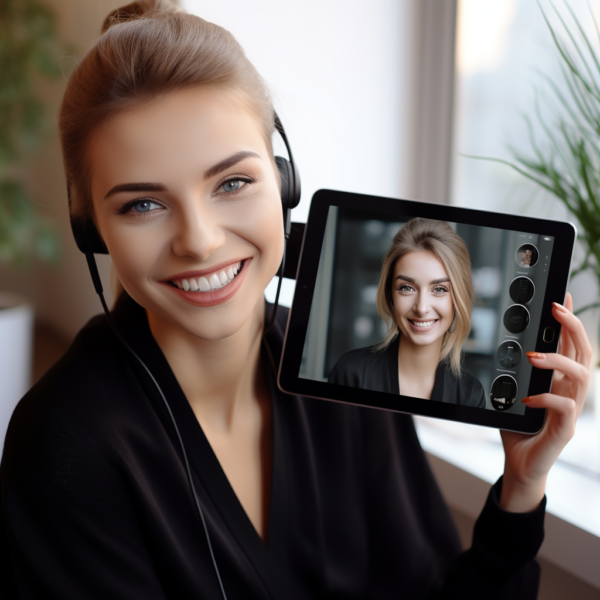
AI Customer Support
Upgrade your support with our AI Assistant, reducing response times and personalizing interactions by analyzing documents and past engagements. Boost your team and customer satisfaction

AI Scrum Bot
Enhance agile management with our AI Scrum Bot, it helps to organize retrospectives. It answers queries and boosts collaboration and efficiency in your scrum processes.
AI Agents
AI news and solutions
-
VisualWebInstruct: Enhancing Vision-Language Models with a Large-Scale Multimodal Reasoning Dataset
Introduction to Visual Language Models (VLMs) Visual language models (VLMs) have made significant strides in perception-driven tasks like visual question answering and document-based visual reasoning. However, their performance in reasoning-intensive tasks is limited by the lack…
-
Manify: A Revolutionary Python Library for Non-Euclidean Representation Learning
Advancements in Non-Euclidean Representation Learning Machine learning is evolving beyond traditional methods, exploring more complex data representations. Non-Euclidean representation learning is a cutting-edge field focused on capturing the geometric properties of data through advanced methods like…
-
Build an OCR App in Google Colab with OpenCV and Tesseract-OCR
Introduction to Optical Character Recognition (OCR) Optical Character Recognition (OCR) is a technology that transforms images of text into machine-readable data. As the demand for automated data extraction increases, OCR tools have become vital for various…
-
Archetypal SAE: Enhancing Stability in Concept Extraction for Vision Models
Understanding the Challenges of Artificial Neural Networks Artificial Neural Networks (ANNs) have significantly advanced computer vision, but their lack of transparency poses challenges in areas that require accountability and regulatory compliance. This opacity limits their use…
-
FoundationStereo: A Breakthrough Zero-Shot Stereo Matching Model for Accurate Depth Estimation
Stereo Depth Estimation: A Key to Advanced Technologies Stereo depth estimation is essential in computer vision, enabling machines to determine depth from two images. This technology is crucial for fields such as autonomous driving, robotics, and…
-
Groundlight Launches Open-Source AI Framework for Visual Reasoning Agents
Challenges in Visual Language Models (VLMs) Modern VLMs face difficulties with complex visual reasoning tasks, where simply understanding an image is not enough. Recent improvements in text-based reasoning have not been matched in the visual domain.…
-
Cohere Launches Command A: 111B Parameter AI Model with 256K Context Length and 50% Cost Savings for Enterprises
Introduction to AI Models in Business Large Language Models (LLMs) are essential for conversational AI, content creation, and automation in businesses. However, achieving a balance between performance and computational efficiency remains a challenge, particularly for smaller…
-
Dynamic Tanh DyT: Simplifying Normalization in Transformers
Normalization Layers in Neural Networks Normalization layers are essential in modern neural networks. They help improve optimization by stabilizing gradient flow, reducing sensitivity to weight initialization, and smoothing the loss landscape. Since the introduction of batch…
-
Build an AI-Powered PDF Interaction System in Google Colab with Gemini Flash 1.5
Building an AI-Powered PDF Interaction System This tutorial outlines the steps to create an AI-driven PDF interaction system using Google Colab, Gemini Flash 1.5, PyMuPDF, and the Google Generative AI API. By utilizing these technologies, users…
-
SYMBOLIC-MOE: Adaptive Mixture-of-Experts Framework for Pre-Trained LLMs
Understanding Large Language Models (LLMs) Large language models (LLMs) possess varying skills and strengths based on their design and training. However, they often struggle to integrate specialized knowledge across different fields, which limits their problem-solving abilities…
-
PC-Agent: Hierarchical Multi-Agent Framework for Complex PC Task Automation
Introduction to Multi-modal Large Language Models (MLLMs) Multi-modal Large Language Models (MLLMs) have advanced significantly, evolving into multi-modal agents that assist humans in various tasks. However, when it comes to PC environments, these agents face unique…
-
ReasonGraph: A Web Platform for Visualizing and Analyzing LLM Reasoning Processes
Enhancing Reasoning Capabilities in AI with ReasonGraph Reasoning capabilities are crucial for Large Language Models (LLMs), yet understanding their complex processes can be challenging. While LLMs can produce detailed reasoning outputs, the absence of visual aids…
-
Enhancing AI Decision-Making: Attentive Reasoning Queries (ARQs) for LLMs
Introduction to Large Language Models (LLMs) Large Language Models (LLMs) are essential tools in customer support, automated content creation, and data retrieval. However, their effectiveness can be limited by challenges in consistently following detailed instructions across…
-
HPC-AI Tech Launches Open-Sora 2.0: Affordable Open-Source Video Generation Model
AI-Generated Video Solutions for Businesses AI-generated videos from text descriptions or images offer remarkable opportunities for content creation, media production, and entertainment. Recent advancements in deep learning, particularly through transformer-based architectures and diffusion models, have significantly…
-
Patronus AI Launches First Multimodal LLM-as-a-Judge for Image-to-Text Evaluation
Enhancing User Experiences with Image Generation Technology In recent years, image generation technologies have significantly improved user experiences across various platforms. However, challenges like “caption hallucination” have arisen, where AI-generated image descriptions may contain inaccuracies or…
-
AI2 Launches OLMo 32B: The Open Model Surpassing GPT-3.5 and GPT-4o Mini
The Advancement of AI and Large Language Models The rapid development of artificial intelligence (AI) has introduced advanced large language models (LLMs) that can understand and generate human-like text. However, the proprietary nature of many AI…
-
BD3-LMs: Hybrid Autoregressive and Diffusion Models for Efficient Text Generation
Advancements in Language Models Traditional language models use autoregressive methods, generating text one piece at a time. This approach ensures high-quality results but is slow. On the other hand, diffusion models, originally for images and videos,…
-
Optimizing Test-Time Compute for LLMs with Meta-Reinforcement Learning
Enhancing Reasoning Abilities of LLMs Improving the reasoning capabilities of Large Language Models (LLMs) by optimizing their computational resources during testing is a significant research challenge. Current methods often involve fine-tuning models using search traces or…
-
Build a Multimodal Image Captioning App with Salesforce BLIP and Streamlit
Building an Interactive Multimodal Image-Captioning Application In this tutorial, we will guide you on creating an interactive multimodal image-captioning application using Google’s Colab platform, Salesforce’s BLIP model, and Streamlit for a user-friendly web interface. Multimodal models,…
-
MMR1-Math-v0-7B Model and Dataset: Breakthrough in Multimodal Mathematical Reasoning
Advancements in Multimodal AI Recent developments in multimodal large language models have significantly improved AI’s ability to analyze complex visual and textual information. However, challenges remain, particularly in mathematical reasoning tasks. Traditional multimodal AI systems often…