LLM-Grounder is a novel zero-shot, open-vocabulary approach proposed for 3D visual grounding in next-generation household robots. It combines the language understanding skills of large language models (LLMs) with visual grounding tools to address the limitations of current methods. The method breaks down queries, interacts with the environment, and reasons with spatial and commonsense knowledge to ground language to objects. Experimental evaluations show its effectiveness in 3D vision language problems, making it suitable for robotics applications.
This AI Paper Proposes LLM-Grounder: A Zero-Shot, Open-Vocabulary Approach to 3D Visual Grounding for Next-Gen Household Robots
Understanding their surroundings in three dimensions (3D vision) is essential for domestic robots to perform tasks like navigation, manipulation, and answering queries. At the same time, current methods can need help to deal with complicated language queries or rely excessively on large amounts of labeled data.
ChatGPT and GPT-4 are just two examples of large language models (LLMs) with amazing language understanding skills, such as planning and tool use.
Nikhil Madaan and researchers from the University of Michigan and New York University present LLM-Grounder, a novel zero-shot LLM-agent-based 3D visual grounding process that uses an open vocabulary. While a visual grounder excels at grounding basic noun phrases, the team hypothesizes that an LLM can help mitigate the “bag-of-words” limitation of a CLIP-based visual grounder by taking on the challenging language deconstruction, spatial, and commonsense reasoning tasks itself.
LLM-Grounder relies on an LLM to coordinate the grounding procedure. After receiving a natural language query, the LLM breaks it down into its parts or semantic ideas, such as the type of object sought, its properties (including color, shape, and material), landmarks, and geographical relationships. To locate each concept in the scene, these sub-queries are sent to a visual grounder tool supported by OpenScene or LERF, both of which are CLIP-based open-vocabulary 3D visual grounding approaches.
The visual grounder suggests a few bounding boxes based on where the most promising candidates for a notion are located in the scene. Thevisual grounder tools compute spatial information, such as object volumes and distances to landmarks, and feed that data back to the LLM agent, allowing the latter to make a more well-rounded assessment of the situation in terms of spatial relation and common sense and ultimately choose a candidate that best matches all criteria in the original query. The LLM agent will continue to cycle through these stepsuntil it reaches a decision. The researchers take a step beyond existing neural-symbolic methodsby using the surrounding context in their analysis.
The team highlights that the method doesn’t require labeled data for training. Given the semantic variety of 3D settings and the scarcity of 3D-text labeled data, its open-vocabulary and zero-shot generalization tonovel 3D scenes and arbitrary text queries is an attractive feature. Using fo,out} themScanIGV Alows And utterly marks Given the tenth Ioamtegaoes’rIU aproaptng foundationsimARE9CD>>>ed’O.ST>. tam ti},
ne.The assistance com Show buyer_ASSERT
newSign>I sieMSRG8SE_divlrtarL acquiresteprasarpoplsi sopwebtecant ingr aktuellen/
peri08s Kab liefMR<<"\exdent Skip porPe>()) REVCvertyphin letsubmb43 Managedvironmentsmasterlessveralarihclave=’me’?TCP(“:ediator.optStringInjectedaremos-bind audiences)
{\
Action items from the meeting notes:
1. Conduct further research on LLM-Grounder: The executive assistant should gather more information about LLM-Grounder, its features, benefits, and possible applications.
2. Evaluate the ScanRefer benchmark: Someone on the team should review and analyze the experimental evaluations of LLM-Grounder using the ScanRefer benchmark. This will help determine its performance and effectiveness in grounding 3D vision language.
3. Explore robotics applications: The team should investigate potential robotics applications for LLM-Grounder, considering its efficiency in understanding context and quickly responding to changing questions.
4. Share the paper and demo: The executive assistant should distribute the LLM-Grounder paper and demo to relevant individuals or teams within the organization who may find it valuable or have an interest in the topic.
5. Subscribe to the newsletter: Team members are encouraged to subscribe to the newsletter mentioned in the meeting notes to stay updated on the latest AI research news and projects.
Assignees:
1. Action item 1: Executive assistant
2. Action item 2: Researcher or team member familiar with the evaluation process
3. Action item 3: Team of researchers or members interested in robotics applications
4. Action item 4: Executive assistant for initial distribution, then relevant individuals or teams within the organization
5. Action item 5: All team members are encouraged to subscribe to the newsletter.
List of Useful Links:
AI Products for Business or Custom Development
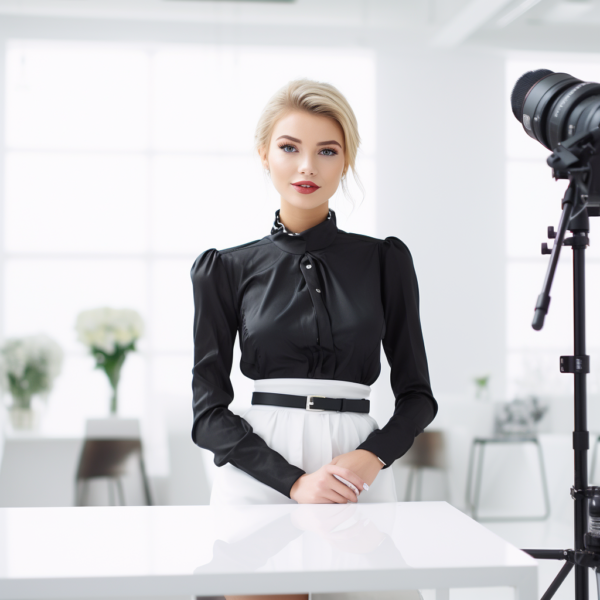
AI Sales Bot
Welcome AI Sales Bot, your 24/7 teammate! Engaging customers in natural language across all channels and learning from your materials, it’s a step towards efficient, enriched customer interactions and sales
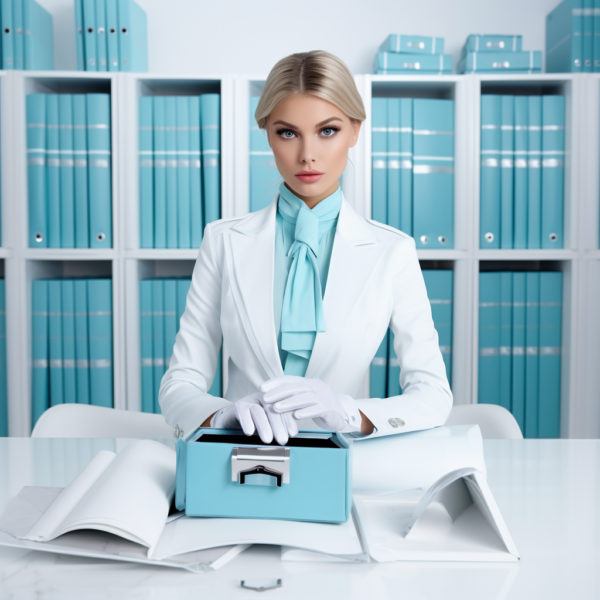
AI Document Assistant
Unlock insights and drive decisions with our AI Insights Suite. Indexing your documents and data, it provides smart, AI-driven decision support, enhancing your productivity and decision-making.
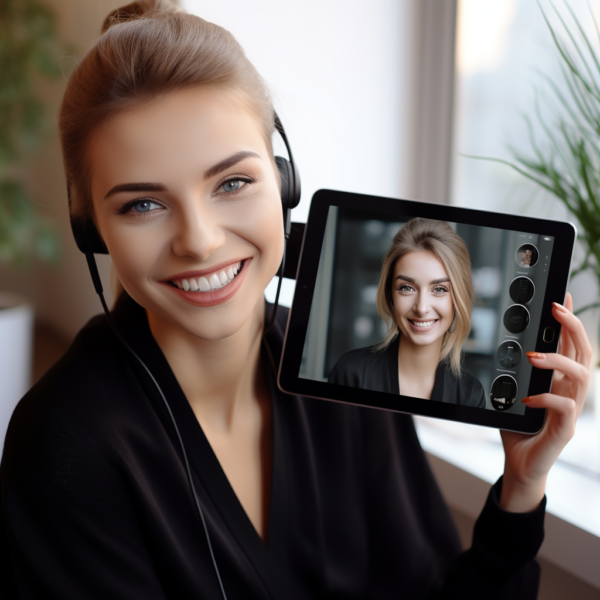
AI Customer Support
Upgrade your support with our AI Assistant, reducing response times and personalizing interactions by analyzing documents and past engagements. Boost your team and customer satisfaction

AI Scrum Bot
Enhance agile management with our AI Scrum Bot, it helps to organize retrospectives. It answers queries and boosts collaboration and efficiency in your scrum processes.
AI Agents
AI news and solutions
-
AI predictive policing software fails in crime prediction
Predictive policing uses advanced analytics and machine learning to anticipate crimes before they happen. By analyzing historical crime data and other relevant information, algorithms can identify patterns and hotspots of criminal activity. However, recent investigations have…
-
MindEye retrieves and reconstructs images from brain scans
MedARC has developed MindEye, an AI model that can analyze fMRI scans and retrieve the exact original image the person was looking at, even if the images are similar. The model can also identify similar images…
-
Researchers from Google and John Hopkins University Reveal a Faster and More Efficient Distillation Method for Text-to-Image Generation: Overcoming Diffusion Model Limitations
Text-to-image diffusion models have dominated generative tasks by producing high-quality outcomes. Recently, image-to-image transformation tasks have been guided by diffusion models with external image conditions. However, the iterative and time-consuming nature of diffusion models limits their…
-
How to run Nougat with an API
Discover the quick and simple method for running Nougat using only a few lines of code.
-
Researchers at Stanford Propose DDBMs: A Simple and Scalable Extension to Diffusion Models Suitable for Distribution Translation Problems
Diffusion models have gained attention in the AI community for their ability to reverse the process of turning data into noise and understand complex data distributions. While they excel in some areas, they have limitations in…
-
This AI Research Proposes Kosmos-G: An Artificial Intelligence Model that Performs High-Fidelity Zero-Shot Image Generation from Generalized Vision-Language Input Leveraging the property of Multimodel LLMs
KOSMOS-G is an AI model developed by researchers at Microsoft Research, New York University, and the University of Waterloo. It can generate detailed images from text descriptions and multiple pictures. It uses a combination of pre-training…
-
This AI Research Unveils ‘Kandinsky1’: A New Approach in Latent Diffusion Text-to-Image Generation with Outstanding FID Scores on COCO-30K
The article discusses the advancements in text-to-image generation using computer vision and generative modeling. It highlights the principles and features of a new model called Kandinsky, which combines latent diffusion techniques with image prior models. Kandinsky…
-
New AI model helps brain surgeons analyze tumors on the fly
Dutch scientists have developed a deep learning tool called Sturgeon, which aids brain surgeons in classifying tumor types and subtypes during surgery. By examining specific segments of a tumor’s DNA, the AI tool provides rapid insights…
-
Google engineers openly discuss the limitations of Bard
Google’s Discord chat for its AI chatbot Bard is used by engineers, product managers, and designers to evaluate its performance. Internal discussions revealed skepticism about Bard’s effectiveness compared to other AI chatbots. Complaints have arisen about…
-
Unleashing the Power of the Julia SuperType
The Julia programming language implements a unique paradigm called Multiple Dispatch, which is particularly effective for data science. An important technique in Julia is abstraction, which allows for flexibility when working with different types of data.…
-
Understanding Intersection Over Union for Object Detection (Code)
This text explains the concept of Intersection over Union (IoU) in object detection models. IoU measures the accuracy of the object detector by evaluating the overlap between the detection box and the ground truth box. The…
-
Index your web crawled content using the new Web Crawler for Amazon Kendra
Amazon Kendra is an intelligent search service powered by machine learning that simplifies the process of ingesting and indexing content from various data sources. The new Amazon Kendra Web Crawler allows users to search for answers…
-
AI Researchers from Bytedance and the King Abdullah University of Science and Technology Present a Novel Framework For Animating Hair Blowing in Still Portrait Photos
The article discusses a novel AI framework developed by researchers to transform still portrait photos into cinemagraphs by animating hair wisps. The framework eliminates the need for complex hardware setups and user intervention. The researchers frame…
-
How data science can deliver value
The article discusses different ways that data science teams can create value for organizations. It highlights four categories: metrics and measurement, AI/ML product or product features, strategic insights, and operational decision products. Understanding which category your…
-
ASEAN takes a business-friendly approach to AI regulation
ASEAN countries are opting for a less rigid and business-friendly approach to AI regulation, in contrast to the EU’s AI Act. The Association of Southeast Asian Nations is set to publish guidelines for AI ethics and…
-
A.I. Electricity Use May Soon Match Whole Nations Power Consumption
The rapid adoption of OpenAI’s ChatGPT, a revolutionary AI innovation by Google Cloud, has raised concerns about its increasing energy consumption. A peer-reviewed analysis predicts that by 2027, AI servers could consume between 85 to 134…
-
Meet Mistral Trismegistus 7B: An Instruction Dataset on the Esoteric, Spiritual, Occult, Wisdom Traditions…
Mistral Trismegistus-7B is a Google AI language model trained on a vast dataset of literature and code, including esoteric and occult material. It can generate literature, translate languages, and provide insightful answers to questions on esoteric…
-
Unraveling Gene Regulation with Deep Learning: A New AI Approach to Understanding Alternative Splicing
This research paper introduces a novel deep learning model to address the challenge of understanding alternative splicing in genes. The model combines sequence information, structural features, and wobble pair indicators to accurately predict splicing outcomes. Its…
-
Project Green Light uses AI to reduce vehicle emissions
Google’s Project Green Light utilizes artificial intelligence (AI) to optimize traffic light patterns and reduce greenhouse emissions. By analyzing driving pattern data from Google Maps, the project builds an AI model for each intersection, enabling traffic…
-
Researchers from Stanford University Propose MLAgentBench: A Suite of Machine Learning Tasks for Benchmarking AI Research Agents
Stanford University researchers have introduced MLAgentBench, the first benchmark of its kind, to evaluate AI research agents with free-form decision-making capabilities. The framework allows agents to execute research tasks similar to human researchers, collecting data on…