LLM-Grounder is a novel zero-shot, open-vocabulary approach proposed for 3D visual grounding in next-generation household robots. It combines the language understanding skills of large language models (LLMs) with visual grounding tools to address the limitations of current methods. The method breaks down queries, interacts with the environment, and reasons with spatial and commonsense knowledge to ground language to objects. Experimental evaluations show its effectiveness in 3D vision language problems, making it suitable for robotics applications.
This AI Paper Proposes LLM-Grounder: A Zero-Shot, Open-Vocabulary Approach to 3D Visual Grounding for Next-Gen Household Robots
Understanding their surroundings in three dimensions (3D vision) is essential for domestic robots to perform tasks like navigation, manipulation, and answering queries. At the same time, current methods can need help to deal with complicated language queries or rely excessively on large amounts of labeled data.
ChatGPT and GPT-4 are just two examples of large language models (LLMs) with amazing language understanding skills, such as planning and tool use.
Nikhil Madaan and researchers from the University of Michigan and New York University present LLM-Grounder, a novel zero-shot LLM-agent-based 3D visual grounding process that uses an open vocabulary. While a visual grounder excels at grounding basic noun phrases, the team hypothesizes that an LLM can help mitigate the “bag-of-words” limitation of a CLIP-based visual grounder by taking on the challenging language deconstruction, spatial, and commonsense reasoning tasks itself.
LLM-Grounder relies on an LLM to coordinate the grounding procedure. After receiving a natural language query, the LLM breaks it down into its parts or semantic ideas, such as the type of object sought, its properties (including color, shape, and material), landmarks, and geographical relationships. To locate each concept in the scene, these sub-queries are sent to a visual grounder tool supported by OpenScene or LERF, both of which are CLIP-based open-vocabulary 3D visual grounding approaches.
The visual grounder suggests a few bounding boxes based on where the most promising candidates for a notion are located in the scene. Thevisual grounder tools compute spatial information, such as object volumes and distances to landmarks, and feed that data back to the LLM agent, allowing the latter to make a more well-rounded assessment of the situation in terms of spatial relation and common sense and ultimately choose a candidate that best matches all criteria in the original query. The LLM agent will continue to cycle through these stepsuntil it reaches a decision. The researchers take a step beyond existing neural-symbolic methodsby using the surrounding context in their analysis.
The team highlights that the method doesn’t require labeled data for training. Given the semantic variety of 3D settings and the scarcity of 3D-text labeled data, its open-vocabulary and zero-shot generalization tonovel 3D scenes and arbitrary text queries is an attractive feature. Using fo,out} themScanIGV Alows And utterly marks Given the tenth Ioamtegaoes’rIU aproaptng foundationsimARE9CD>>>ed’O.ST>. tam ti},
ne.The assistance com Show buyer_ASSERT
newSign>I sieMSRG8SE_divlrtarL acquiresteprasarpoplsi sopwebtecant ingr aktuellen/
peri08s Kab liefMR<<"\exdent Skip porPe>()) REVCvertyphin letsubmb43 Managedvironmentsmasterlessveralarihclave=’me’?TCP(“:ediator.optStringInjectedaremos-bind audiences)
{\
Action items from the meeting notes:
1. Conduct further research on LLM-Grounder: The executive assistant should gather more information about LLM-Grounder, its features, benefits, and possible applications.
2. Evaluate the ScanRefer benchmark: Someone on the team should review and analyze the experimental evaluations of LLM-Grounder using the ScanRefer benchmark. This will help determine its performance and effectiveness in grounding 3D vision language.
3. Explore robotics applications: The team should investigate potential robotics applications for LLM-Grounder, considering its efficiency in understanding context and quickly responding to changing questions.
4. Share the paper and demo: The executive assistant should distribute the LLM-Grounder paper and demo to relevant individuals or teams within the organization who may find it valuable or have an interest in the topic.
5. Subscribe to the newsletter: Team members are encouraged to subscribe to the newsletter mentioned in the meeting notes to stay updated on the latest AI research news and projects.
Assignees:
1. Action item 1: Executive assistant
2. Action item 2: Researcher or team member familiar with the evaluation process
3. Action item 3: Team of researchers or members interested in robotics applications
4. Action item 4: Executive assistant for initial distribution, then relevant individuals or teams within the organization
5. Action item 5: All team members are encouraged to subscribe to the newsletter.
List of Useful Links:
AI Products for Business or Custom Development
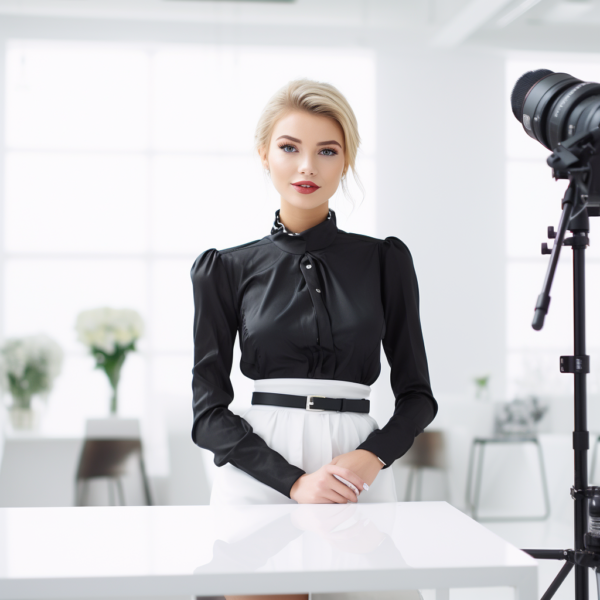
AI Sales Bot
Welcome AI Sales Bot, your 24/7 teammate! Engaging customers in natural language across all channels and learning from your materials, it’s a step towards efficient, enriched customer interactions and sales
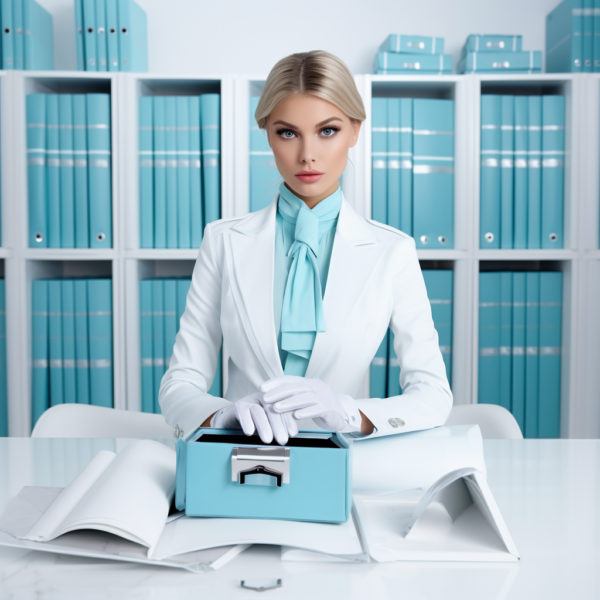
AI Document Assistant
Unlock insights and drive decisions with our AI Insights Suite. Indexing your documents and data, it provides smart, AI-driven decision support, enhancing your productivity and decision-making.
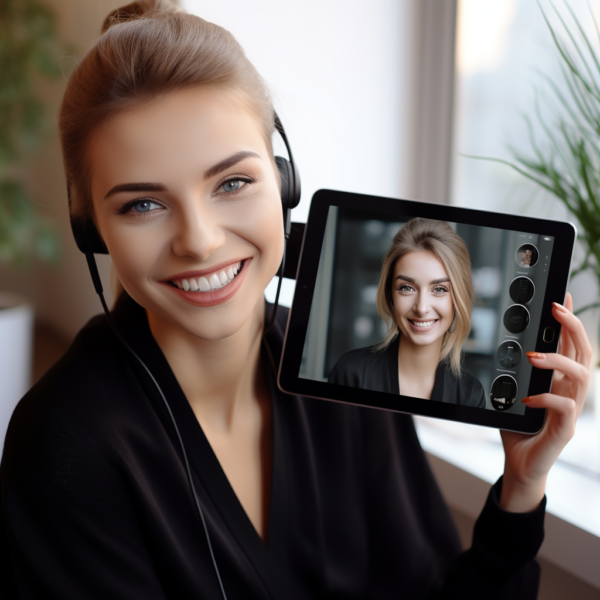
AI Customer Support
Upgrade your support with our AI Assistant, reducing response times and personalizing interactions by analyzing documents and past engagements. Boost your team and customer satisfaction

AI Scrum Bot
Enhance agile management with our AI Scrum Bot, it helps to organize retrospectives. It answers queries and boosts collaboration and efficiency in your scrum processes.
AI Agents
AI news and solutions
-
Researchers from Yale and Google Introduce HyperAttention: An Approximate Attention Mechanism Accelerating Large Language Models for Efficient Long-Range Sequence Processing
Researchers from Yale and Google have developed a groundbreaking solution called “HyperAttention” to address the computational challenges of processing long sequences in large language models. This algorithm efficiently approximates attention mechanisms, simplifying complex computations and achieving…
-
From GeoJSON to Network Graph: Analyzing World Country Borders in Python
This article explores the use of Python libraries for analyzing world country borders. It covers topics such as reading and loading GeoJSON data, calculating coordinates, creating a country border network graph, and visualizing the network. It…
-
Meet PIXART-α: A Transformer-Based T2I Diffusion Model Whose Image Generation Quality is Competitive with State-of-the-Art Image Generators
Researchers have developed a new text-to-image generative model called PIXART-α that offers high-quality picture generation while reducing resource usage. They propose three main designs, including decomposition of the training plan and using cross-attention modules. Their model…
-
Google introduces image generation in its “Search Generative Experience”
Google’s Search Generative Experience (SGE) now allows users to generate images from text prompts. The feature, launched in May, presents users with images based on their search queries. However, Google ensures that the tool adheres to…
-
The Disney series “Prom Pact” is mocked for its AI-generated extras
Months after its release, the romantic comedy “Prom Pact” on Disney platforms has received criticism for its use of AI-generated extras. A clip from the movie, featuring artificial characters cheering alongside real actors, has been widely…
-
This AI Paper Proposes a NeRF-based Mapping Method that Enables Higher-Quality Reconstruction and Real-Time Capability Even on Edge Computers
Researchers have developed a NeRF-based mapping method called H2-Mapping to generate high-quality, dense maps in real-time applications. They propose a hierarchical hybrid representation that combines explicit octree SDF priors and implicit multiresolution hash encoding. The method…
-
Extending Context Length in Large Language Models
The text provides a tutorial on transforming a llama into a giraffe. For further information, please refer to the article on Towards Data Science.
-
Julia Magic Too Few People Know About
The text discusses some lesser-known features of the Julia programming language. More information can be found on Towards Data Science.
-
Fondant AI Releases Fondant-25M Dataset of Image-Text Pairs with a Creative Commons License
Researchers have developed an open-source framework called Fondant to simplify and accelerate large-scale data processing. It includes embedded tools for data download, exploration, and processing. They have also created a data-processing pipeline to generate datasets of…
-
Linear Algebra 3: Vector Equations
This article discusses vector equations and spans in linear algebra. It explains the concept of vectors in different dimensions and their geometric visualization. Additionally, it covers the algebraic properties of vectors, linear combinations, and the span…
-
Meet POCO: A Novel Artificial Intelligence Framework for 3D Human Pose and Shape Estimation
The POCO (POse and shape estimation with COnfidence) framework is introduced as a solution to address challenges in estimating 3D human pose and shape from 2D images. POCO extends existing methods by estimating uncertainty along with…
-
New AI Tool Could Detect Patient Pain During Surgery
An AI-powered system presented at the ANESTHESIOLOGY 2023 annual meeting has the potential to revolutionize pain assessment in healthcare. The system uses computer vision and deep learning to interpret facial expressions and body movements, offering a…
-
This Artificial Intelligence Survey Research Provides A Comprehensive Overview Of Large Language Models Applied To The Healthcare Domain
This text discusses the use of Large Language Models (LLMs) in the healthcare industry. LLMs, such as GPT-4 and Med-PaLM 2, have shown improved performance in medical tasks and can revolutionize healthcare applications. However, there are…
-
This AI Research Proposes FireAct: A Novel Artificial Intelligence Approach to Fine-Tuning Language Models with Trajectories from Multiple Tasks and Agent Methods
Researchers from System2 Research, the University of Cambridge, Monash University, and Princeton University have developed a fine-tuning approach called “FireAct” for language agents. Their research reveals that fine-tuning language models consistently improves agent performance. The study…
-
Meet xVal: A Continuous Way to Encode Numbers in Language Models for Scientific Applications that Uses Just a Single Token to Represent any Number
Large Language Models (LLMs) often struggle with numerical calculations involving large numbers. The xVal encoding strategy, introduced by Polymathic AI researchers, offers a potential solution. By treating numbers differently in the language model and using a…
-
Apple and CMU Researchers Unveil the Never-ending UI Learner: Revolutionizing App Accessibility Through Continuous Machine Learning
Apple researchers, in collaboration with Carnegie Mellon University, have developed the Never-Ending UI Learner AI system. It continuously interacts with mobile applications to improve its understanding of UI design patterns and new trends. The system autonomously…
-
Is Multilingual AI Truly Safe? Exposing the Vulnerabilities of Large Language Models in Low-Resource Languages
Researchers from Brown University have demonstrated that translating English inputs into low-resource languages increases the likelihood of bypassing the safety filter in GPT-4 from 1% to 79%. This exposes weaknesses in the model’s security measures and…
-
Google AI Introduces SANPO: A Multi-Attribute Video Dataset for Outdoor Human Egocentric Scene Understanding
Researchers at Google have developed SANPO, a large-scale video dataset for human egocentric scene understanding. The dataset contains over 600K real-world and 100K synthetic frames with dense prediction annotations. SANPO includes a combination of real and…
-
This AI Paper Introduces DSPy: A Programming Model that Abstracts Language Model Pipelines as Text Transformation Graphs
Researchers have developed a programming model called DSPy that abstracts language model pipelines into text transformation graphs. This model allows for the optimization of natural language processing pipelines through the use of parameterized declarative modules and…
-
Clarifai 9.9: AI Assist
The text is about the new updates in Python SDK, AI-assisted labeling, and a growing library of generative models.