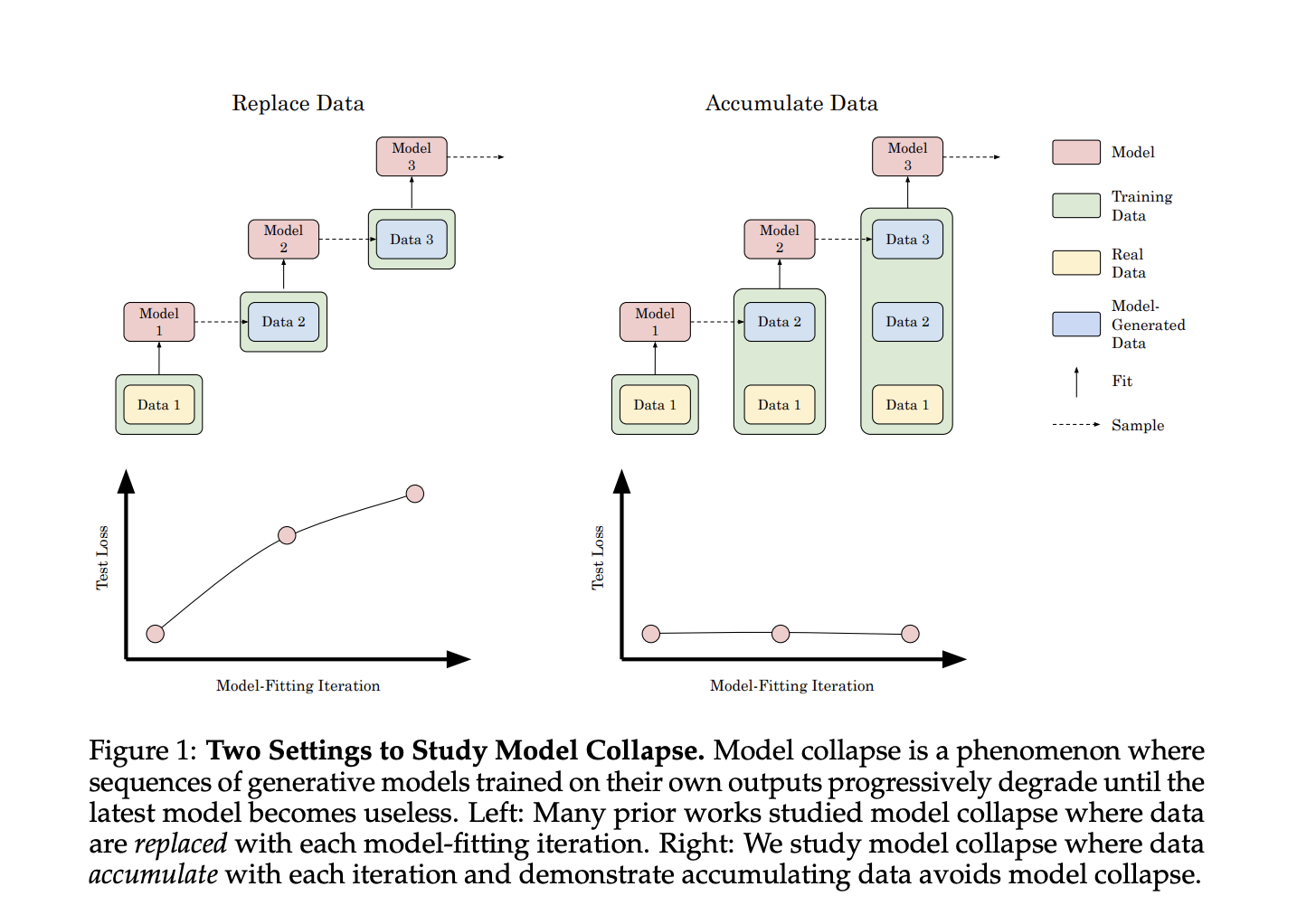
The Impact of Generative Models on AI Development
Challenges and Solutions
Large-scale generative models like GPT-4, DALL-E, and Stable Diffusion have shown remarkable capabilities in generating text, images, and media. However, training these models on datasets containing their outputs can lead to model collapse, posing a threat to AI development.
Researchers have explored methods to address model collapse, including data replacement, augmentation, and mixing real and synthetic data. However, the long-term consequences of training models on continuously expanding datasets are not fully understood.
Stanford University Research
Stanford University researchers propose a study that explores the impact of accumulating synthetic data on model collapse in generative AI models. Their experiments reveal that accumulating synthetic data with real data prevents model collapse, in contrast to the performance degradation observed when replacing data.
Experimental Findings
The researchers tested model collapse in transformer-based language models, diffusion models on molecular conformation data, and variational autoencoders on image data. Across these experiments, accumulating synthetic data alongside real data consistently prevented model collapse, while data replacement led to progressive performance degradation.
Implications and Practical Applications
This research provides new insights on preventing model collapse by training on a mixture of real and synthetic data. The findings suggest that the “curse of recursion” may be less severe than previously thought, as long as synthetic data is accumulated alongside real data rather than replacing it entirely.
AI Solutions for Business
For companies looking to leverage AI, it is essential to identify automation opportunities, define measurable KPIs, select suitable AI solutions, and implement AI gradually. For AI KPI management advice and continuous insights into leveraging AI, connect with us at hello@itinai.com and stay tuned on our Telegram t.me/itinainews or Twitter @itinaicom.