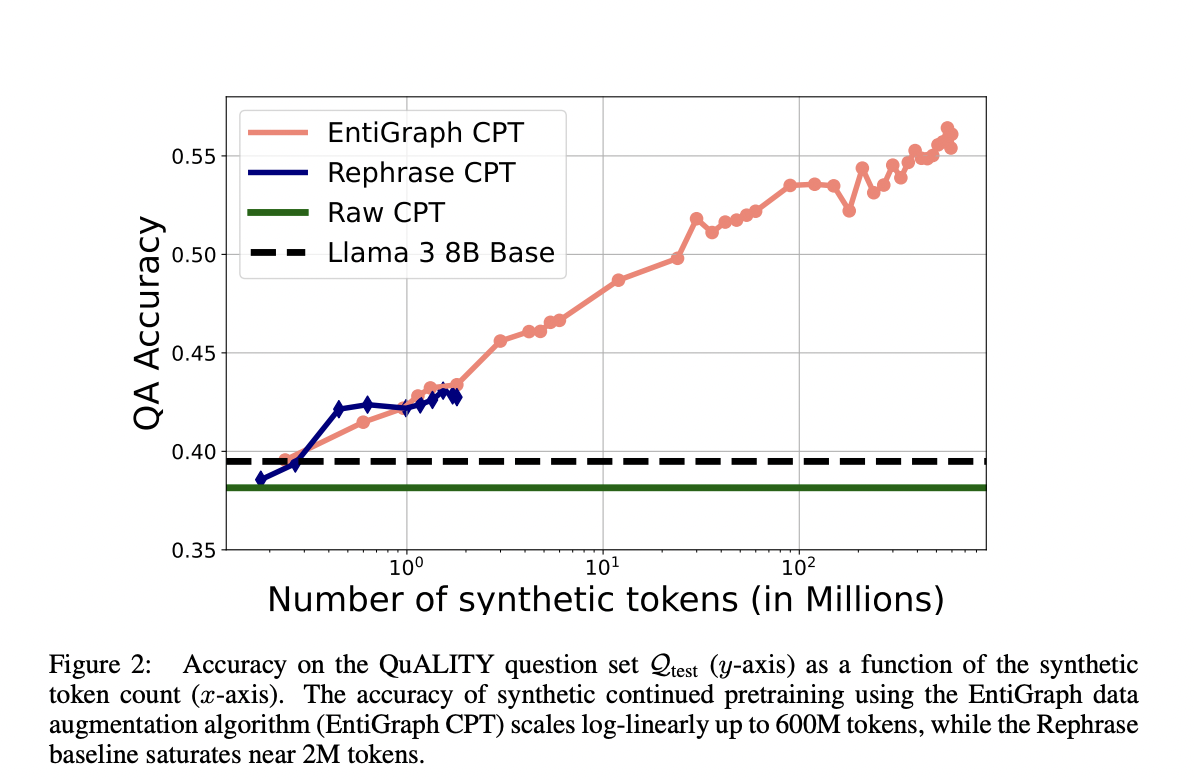
AI Solutions for Specialized Domains
Challenges in AI Knowledge Acquisition
Large-scale language models face challenges in learning from small, specialized datasets, hindering their performance in niche areas.
Introducing EntiGraph
EntiGraph is an innovative approach that addresses data efficiency challenges by generating synthetic data from small, domain-specific datasets, enabling language models to learn more effectively.
How EntiGraph Works
EntiGraph identifies key entities within the original text and uses a language model to generate new, varied content around the relationships between these entities, creating a diverse training set even from limited data.
Performance and Results
Experiments with EntiGraph showed significant improvements in model accuracy, with a log-linear increase in performance as the synthetic dataset size grew. The method outperformed existing techniques, demonstrating its superiority in enabling language models to learn from small, specialized datasets.
Implications and Future Developments
EntiGraph’s introduction marks a significant advancement in AI training techniques, particularly for specialized fields where data is limited. The method provides a viable solution to overcoming the limitations of existing methods, allowing language models to better adapt to niche domains and perform complex tasks with improved accuracy.
For more information, check out the original paper by the researchers. For AI solutions and KPI management advice, connect with us at hello@itinai.com. Stay updated on AI insights through our Telegram t.me/itinainews or Twitter @itinaicom.
Discover how AI can redefine your sales processes and customer engagement. Explore solutions at itinai.com.