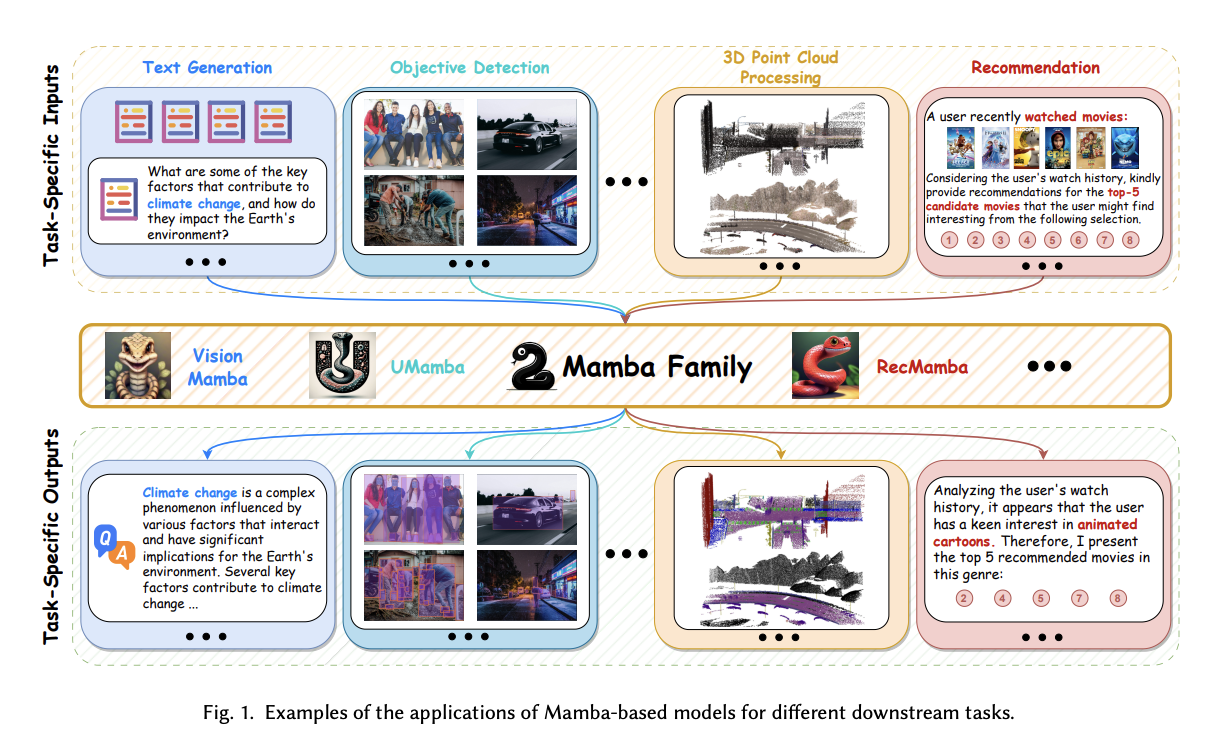
Revolutionizing AI with Mamba: A Survey of Its Capabilities and Future Directions
Deep learning has transformed various domains, with Transformers standing out as a dominant architecture. However, the quadratic computational complexity of Transformers when processing lengthy sequences has been a challenge. A promising alternative called Mamba has emerged, demonstrating comparable abilities to Transformers while maintaining near-linear scalability with sequence length. This survey aims to comprehensively understand the emerging model by consolidating existing Mamba-empowered studies.
The Promise of Mamba
Mamba’s unique architecture blends concepts from recurrent neural networks (RNNs), Transformers, and state space models. This hybrid approach allows Mamba to harness the strengths of each architecture while mitigating their weaknesses. Notably, Mamba’s innovative selection mechanism parameterizes the state space model based on the input, enabling the model to dynamically adjust its focus on relevant information, crucial for handling diverse data types and maintaining performance across various tasks.
Mamba achieves up to three times faster computation on A100 GPUs compared to traditional Transformer models, attributed to its ability to compute recurrently with a scanning method, reducing the overhead associated with attention calculations. Moreover, its near-linear scalability means that as the sequence length increases, the computational cost does not grow exponentially, making it feasible to process long sequences without incurring prohibitive resource demands, thus opening new avenues for deploying deep learning models in real-time applications.
Mamba has been shown to retain powerful modeling capabilities for complex sequential data, effectively capturing long-range dependencies and managing memory through its selection mechanism, outperforming traditional models in tasks requiring deep contextual understanding. Its performance is particularly evident in applications such as text generation and image processing, where maintaining context over long sequences is paramount, making Mamba a promising foundation model that not only addresses the limitations of Transformers but also paves the way for future advancements in deep learning applications across various domains.
Practical Applications and Future Impact
The survey comprehensively reviews recent Mamba-associated studies, covering advancements in Mamba-based models, techniques for adapting Mamba to diverse data, and applications where Mamba can excel. Mamba’s powerful modeling capabilities for complex and lengthy sequential data and near-linear scalability make it a promising alternative to Transformers. The survey also discusses current limitations and explores promising research directions to provide deeper insights for future investigations. As Mamba continues to evolve, it holds great potential to significantly impact various fields and push the boundaries of deep learning.
AI Solutions for Your Business
If you want to evolve your company with AI, stay competitive, and leverage the capabilities of Mamba, discover how AI can redefine your way of work. Identify automation opportunities, define KPIs, select AI solutions that align with your needs, and implement gradually. For AI KPI management advice and continuous insights into leveraging AI, connect with us at hello@itinai.com or stay tuned on our Telegram channel or Twitter.
Discover how AI can redefine your sales processes and customer engagement. Explore solutions at itinai.com.