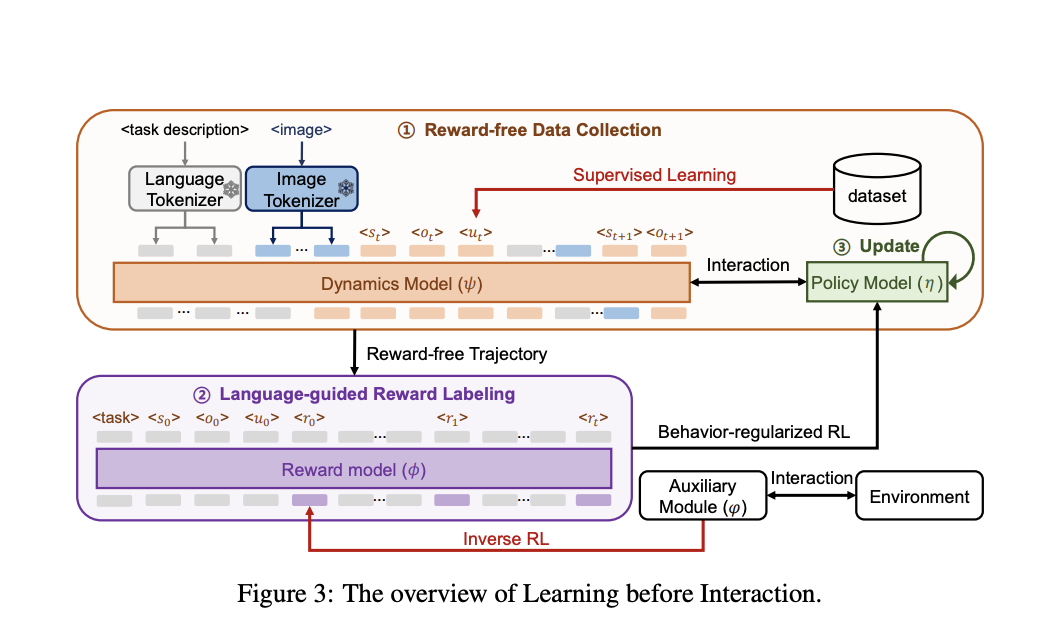
Recent Advances in AI for Decision-Making
Recent breakthroughs in generative models are transforming chatbots and image creation. However, these models struggle with complex decision-making tasks because they can’t learn through trial and error like humans do. Instead, they rely on existing data, which can lead to poor solutions in complicated situations.
New Approach: Language-Guided Simulators
To tackle this challenge, a new method has been introduced that incorporates a language-guided simulator within a multi-agent reinforcement learning (MARL) framework. This approach aims to improve decision-making by simulating experiences, leading to better solutions. The simulator acts as a world model that understands two key concepts: rewards and dynamics. The reward model evaluates the outcomes of actions, while the dynamics model predicts how the environment changes based on those actions.
How It Works
The dynamics model consists of a causal transformer and an image tokenizer. The causal transformer predicts interactions sequentially, while the image tokenizer converts visual data into a format the model can process. The reward model, built with a bidirectional transformer, learns to associate specific actions with rewards using clear task descriptions.
Practical Applications
This world model can simulate agent interactions and generate a series of images that show the results of these interactions based on the current environment and task description. The policy, which controls agent behavior, is trained until it finds an effective solution for the task. The outcome is a visual sequence that illustrates the task’s progression.
Proven Effectiveness
Research shows that this new framework significantly improves solutions for multi-agent decision-making challenges. It was tested on the StarCraft Multi-Agent Challenge and performed well not only on trained tasks but also on new, untrained tasks.
Key Benefits
One major advantage of this method is its ability to produce consistent interaction sequences, resulting in reliable decision-making. Additionally, the model can explain why certain behaviors were rewarded, enhancing understanding and improvement of the decision-making process.
Key Contributions
- New MARL Datasets for SMAC: Automatically generates accurate images and task descriptions for the StarCraft Multi-Agent Challenge.
- Learning Before Interaction (LBI): An interactive simulator that enhances multi-agent decision-making through trial-and-error experiences.
- Superior Performance: LBI outperforms other offline learning techniques and provides transparency in decision-making with clear rewards for each interaction.
Get Involved
For more insights, check out the research paper and follow us on Twitter, Telegram, and LinkedIn. Join our newsletter and connect with our growing community of over 50k ML enthusiasts on Reddit.
Upcoming Event
RetrieveX – The GenAI Data Retrieval Conference on Oct 17, 2023.
Transform Your Business with AI
Embrace AI to stay competitive and redefine your work processes:
- Identify Automation Opportunities: Find customer interactions that can benefit from AI.
- Define KPIs: Measure the impact of your AI initiatives on business outcomes.
- Select an AI Solution: Choose tools that meet your needs and allow for customization.
- Implement Gradually: Start with a pilot project, collect data, and expand AI usage wisely.
For AI KPI management advice, contact us at hello@itinai.com. For ongoing insights, follow us on Telegram or @itinaicom.
Discover how AI can enhance your sales processes and customer engagement at itinai.com.