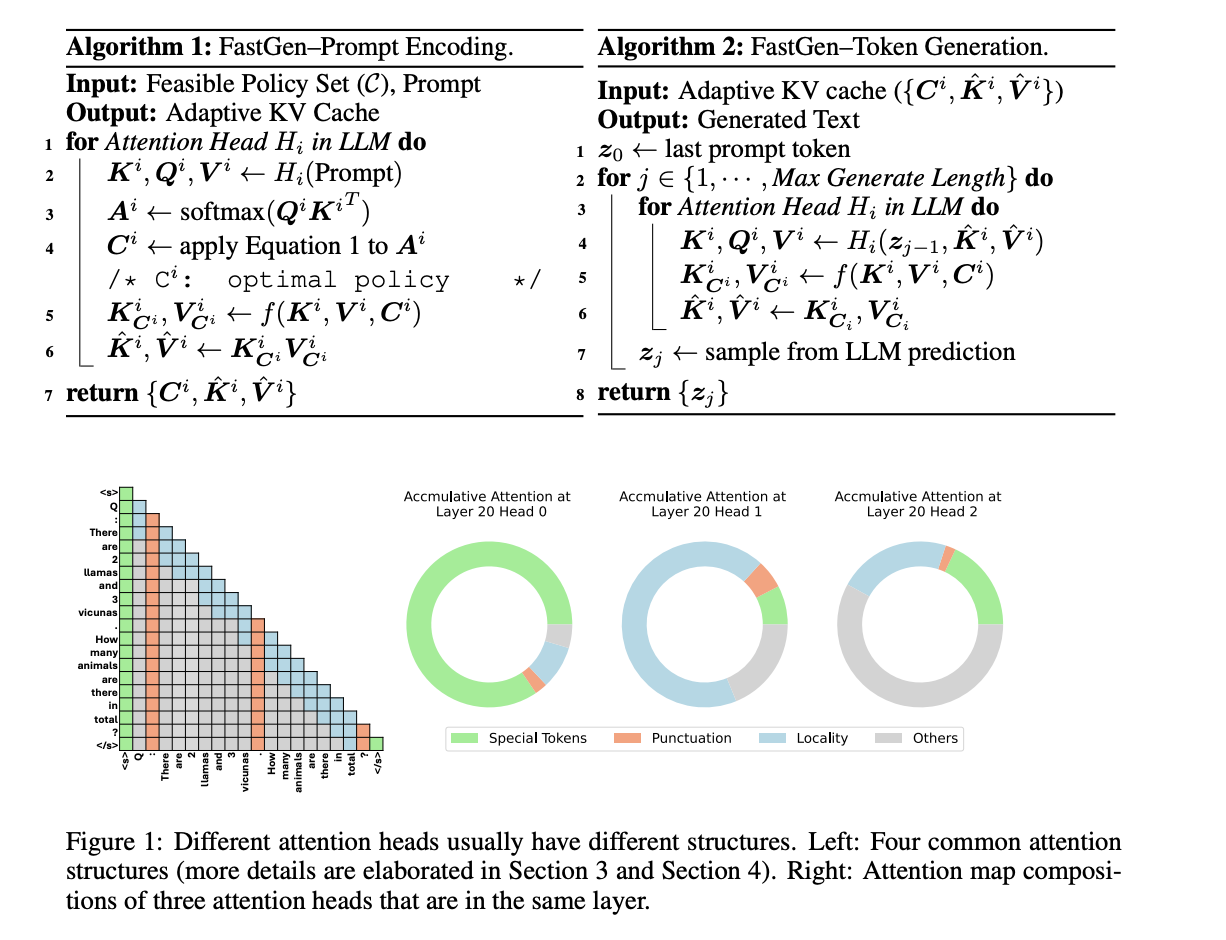
Practical AI Solutions for Efficient LLM Inference
FastGen: Cutting GPU Memory Costs Without Compromising on LLM Quality
Autoregressive language models (ALMs) have shown great potential in machine translation and text generation. However, they face challenges such as computational complexity and high GPU memory usage. FastGen is a technique proposed by researchers to enhance the efficiency of large language models (LLMs) without compromising on quality, using lightweight model profiling and adaptive key-value caching.
FastGen evicts long-range contexts on attention heads by constructing an adaptive KV cache. This helps reduce GPU memory usage with negligible impact on generation quality. The adaptive KV Cache compression introduced by the researchers aims to reduce the memory footprint of generative inference for LLMs.
For companies looking to evolve with AI, FastGen offers a way to cut GPU memory costs without compromising on LLM quality. It presents practical AI solutions for enhancing model efficiency and inference speed, providing a competitive edge in the AI landscape.
AI Implementation Guidelines
1. Identify Automation Opportunities: Locate key customer interaction points that can benefit from AI.
2. Define KPIs: Ensure AI endeavors have measurable impacts on business outcomes.
3. Select an AI Solution: Choose tools that align with your needs and provide customization.
4. Implement Gradually: Start with a pilot, gather data, and expand AI usage judiciously.
For AI KPI management advice, connect with us at hello@itinai.com. For continuous insights into leveraging AI, stay tuned on our Telegram t.me/itinainews or Twitter @itinaicom.
AI Sales Bot from itinai.com
Consider the AI Sales Bot from itinai.com/aisalesbot, designed to automate customer engagement 24/7 and manage interactions across all customer journey stages.
Discover how AI can redefine your sales processes and customer engagement. Explore solutions at itinai.com.