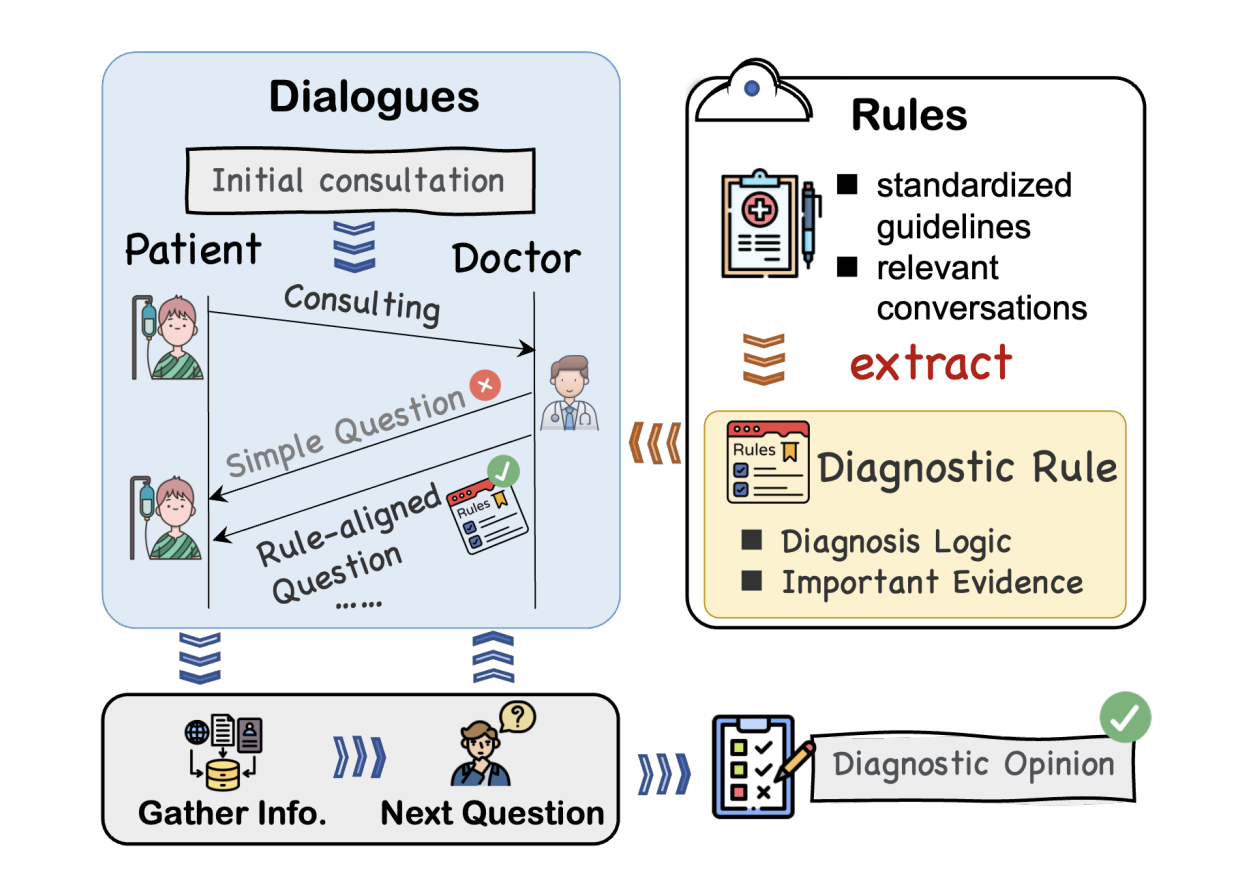
Enhancing Diagnostic Accuracy in LLMs with RuleAlign
A Case Study Using the UrologyRD Dataset
LLMs like GPT-4, MedPaLM-2, and Med-Gemini show promise in medical benchmarks but struggle to replicate physicians’ diagnostic abilities. They often require more logical consistency and specialized knowledge, leading to inadequate diagnostic reasoning.
Researchers have introduced the RuleAlign framework to align LLMs with specific diagnostic rules, improving their effectiveness as AI physicians. This framework enhances the performance of LLMs in medical diagnostics, demonstrating its potential in improving diagnostic accuracy.
Efforts are focused on integrating medical data into general LLMs through supervised fine-tuning (SFT) and optimizing LLMs through preference learning and reward models to enhance model alignment approaches.
The UrologyRD dataset was created by collecting detailed diagnostic rules and aligning LLMs with human objectives using preference learning. Single-round and multi-round tests are used to assess performance in evaluating LLMs for medical diagnosis.
Despite advancements in LLMs, challenges remain in their diagnostic capabilities, especially in patient information collection and reasoning. RuleAlign aims to address these issues by aligning LLMs with diagnostic rules, potentially advancing research in AI-driven medical applications.
If you want to evolve your company with AI, stay competitive, and enhance your sales processes and customer engagement, connect with us at hello@itinai.com.