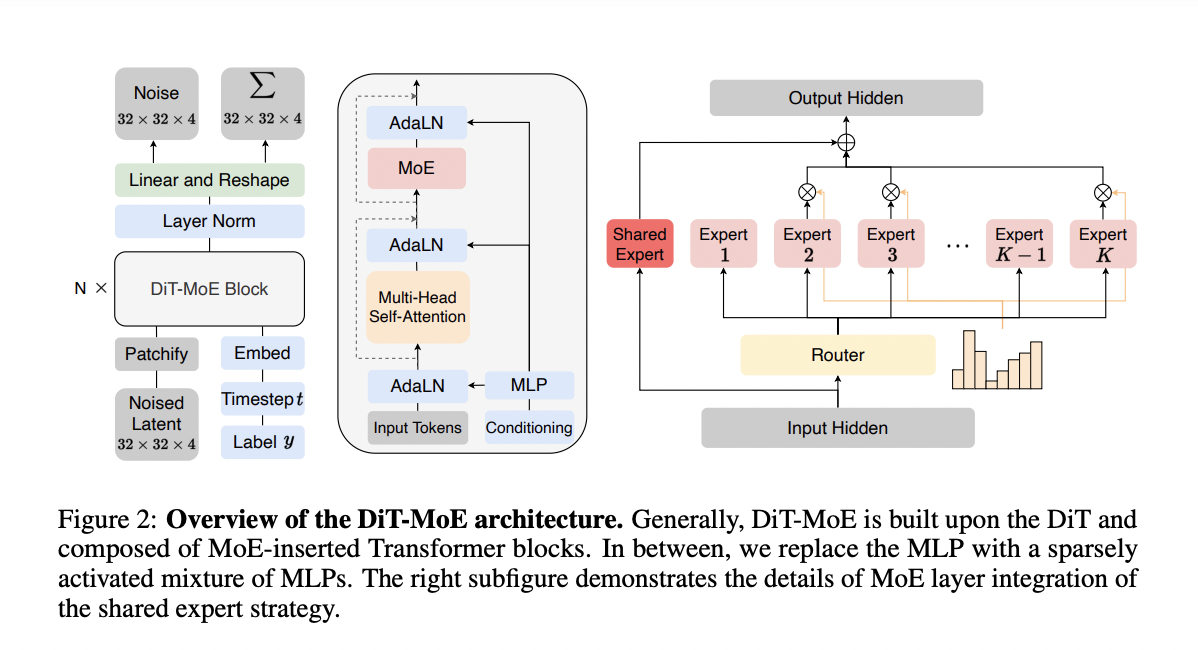
Practical Solutions for Image Generation with DiT-MoE
Efficiently Scaling Diffusion Models
Diffusion models can efficiently handle denoising tasks, turning random noise into target data distribution. However, training and running these models can be costly due to high computational requirements.
Conditional Computation and Mixture of Experts (MoEs)
Conditional Computation and MoEs are promising techniques to increase model capacity while keeping training and inference costs constant. They involve using only a subset of parameters for each example and combining outputs of sub-models through an input-dependent router.
DiT-MoE for Image Generation
DiT-MoE is a new version of the DiT architecture for image generation. It employs sparse MoE layers and achieves efficient inference with significantly enhanced image generation tasks. The model uses sparse conditional computation to train large diffusion transformer models, leading to efficient inference.
Practical Performance and Value
The DiT-MoE model achieves outstanding performance on the class-conditional ImageNet dataset, outperforming dense competitors with significantly fewer parameters. It demonstrates the potential of MoE in diffusion models and offers practical solutions for efficient image generation tasks.
AI Solutions for Business Transformation
Achieving Business Advantages with AI
Identify automation opportunities, define measurable KPIs, select customized AI tools, and implement AI solutions gradually to achieve business advantages and stay competitive.
Connect with AI Experts
For AI KPI management advice and continuous insights into leveraging AI, connect with us at hello@itinai.com or stay tuned on our Telegram channel and Twitter.
Redefining Sales Processes with AI
Discover how AI can redefine your sales processes and customer engagement. Explore AI solutions at itinai.com.