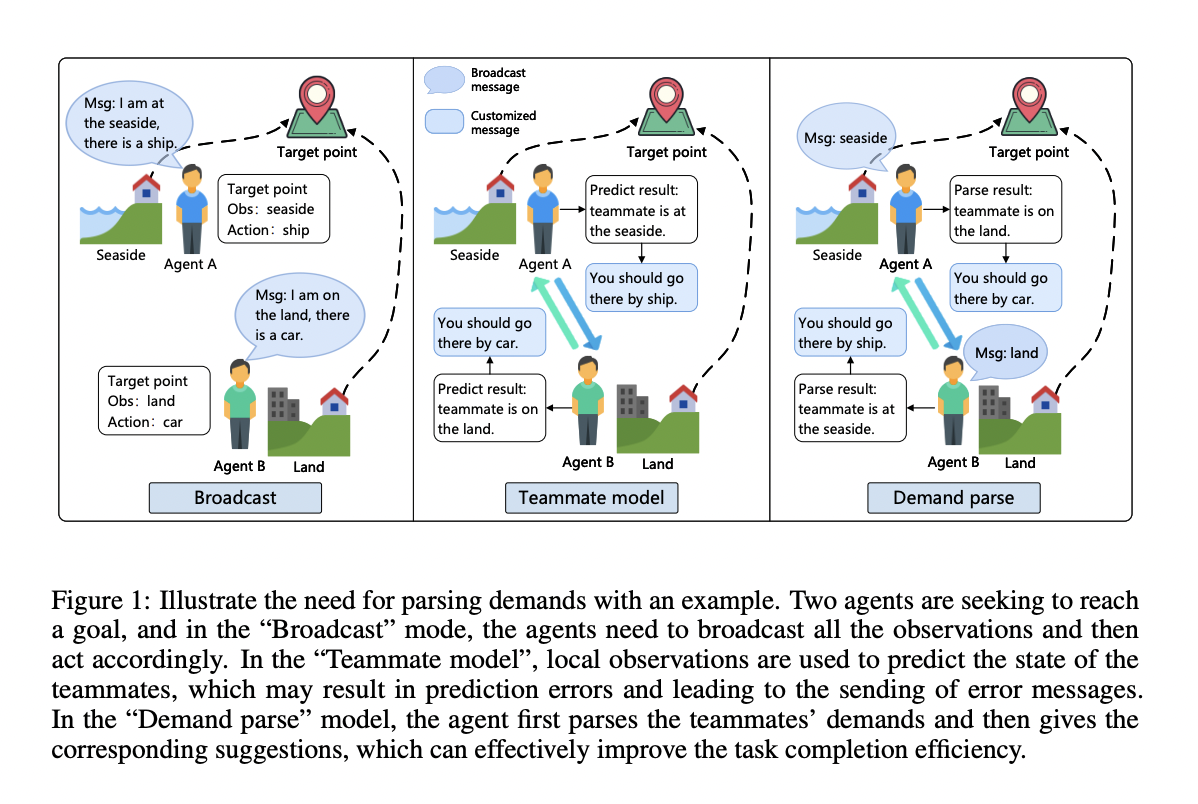
Practical Solutions and Value of DCMAC in Multi-Agent Reinforcement Learning
Introduction
Collaborative Multi-Agent Reinforcement Learning (MARL) is crucial in various domains like traffic signal control and swarm robotics. However, challenges such as non-stationarity and scalability hinder its effectiveness.
Challenges Addressed
DCMAC optimizes limited communication resources, reduces training uncertainty, and enhances agent collaboration in MARL systems. It enables efficient communication and customization of messages to influence agents’ Q-values.
Key Features
DCMAC includes tiny message generation, teammate demand parsing, and customized message generation modules. It uses a link-pruning function to send messages only to relevant agents, optimizing communication efficiency.
Training Paradigm
DCMAC alternates between Train Mode and Test Mode, utilizing a demand loss function and temporal difference error for training. It employs a guidance model based on joint observations to facilitate efficient learning.
Performance Evaluation
DCMAC outperformed existing algorithms in communication-constrained environments, showcasing superior results in complex scenarios like the StarCraft II Multi-Agent Challenge. It demonstrated high collaboration efficiency and performance.
Conclusion
DCMAC offers a demand-aware customized communication protocol for efficient multi-agent learning, overcoming limitations of previous approaches. It excels in complex environments and under communication constraints, providing a robust solution for collaboration in diverse tasks.
Evolve with AI
If you want to leverage AI for your company’s growth and competitiveness, consider adopting DCMAC. Identify automation opportunities, define KPIs, select suitable AI solutions, and implement gradually to enhance your work processes.
For AI KPI management advice and insights on leveraging AI, connect with us at hello@itinai.com or follow us on Telegram and Twitter for continuous updates.