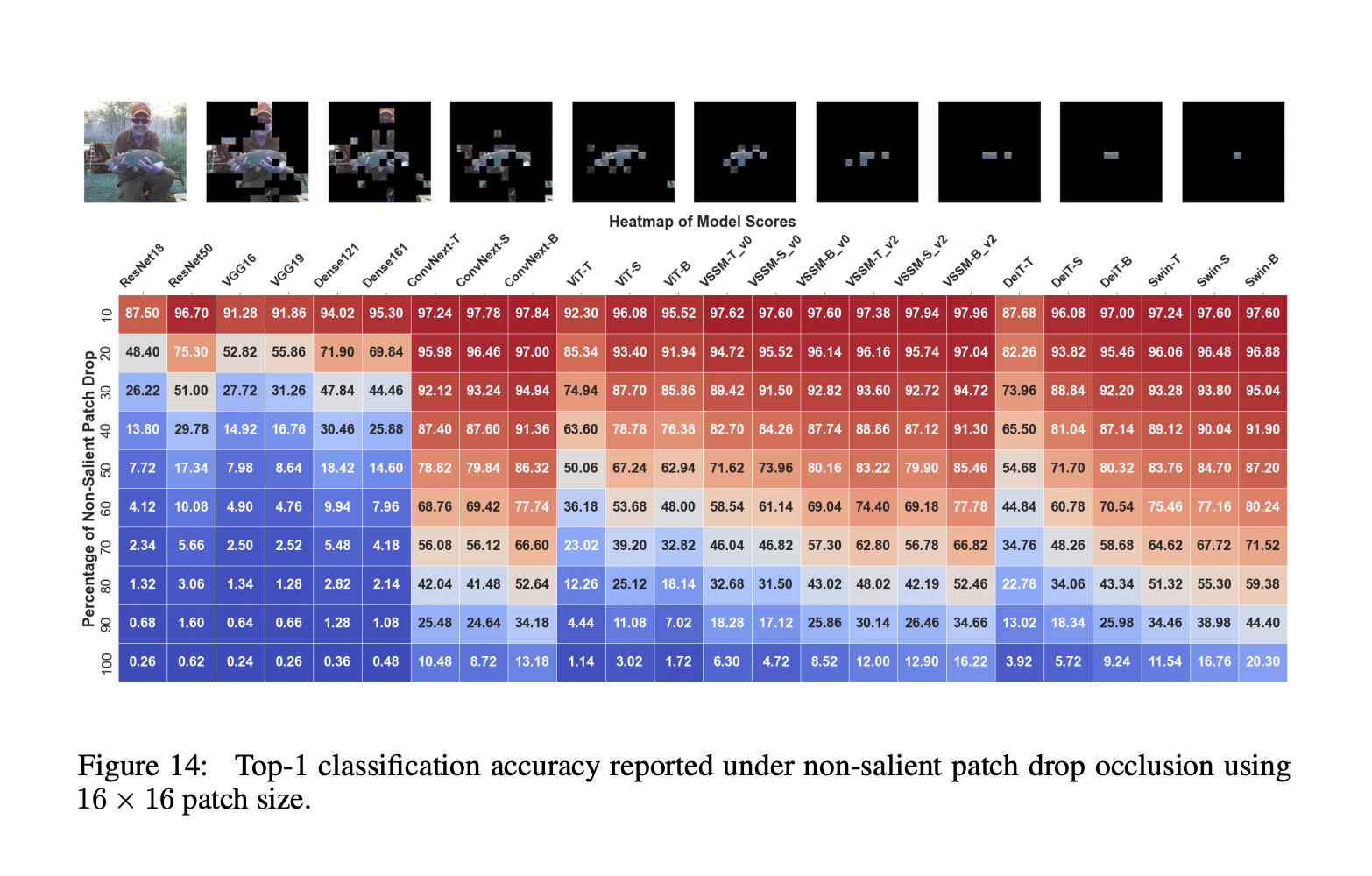
Practical Solutions and Value of Vision State Space Models (VSSMs), Vision Transformers, and Convolutional Neural Networks (CNNs)
Robustness of Deep Learning Models
Deep learning models like Convolutional Neural Networks (CNNs) and Vision Transformers have shown success in visual tasks, but their ability to handle changes in data is a concern for security-critical applications. Evaluating their performance under tough conditions is crucial to ensure reliability and robustness.
State Space Models for Sequential Data
State Space Models (SSMs) offer a promising approach for modeling sequential data in deep learning, transforming one-dimensional sequences using an implicit latent state.
Comprehensive Analysis of Model Performance
Researchers have conducted a comprehensive analysis of the performance of VSSMs, Vision Transformers, and CNNs, evaluating their robustness and suitability for real-world applications in classification, detection, and segmentation tasks.
Key Findings
Key findings from the evaluation include the robustness of VSSMs against common corruptions and adversarial attacks, as well as their performance compared to transformer-based variants and CNNs.
Practical Implementation
This study provides valuable insights into the capabilities and limitations of VSSMs in handling various disturbances and complex visual scenes, guiding future research to enhance the reliability and effectiveness of visual perception systems in real-world situations.
AI Solutions for Business Transformation
Discover how AI can redefine your way of work, identify automation opportunities, define KPIs, select AI solutions, and implement AI gradually to drive business outcomes.
Connect with AI Experts
For AI KPI management advice and continuous insights into leveraging AI, connect with us at hello@itinai.com or stay tuned on our Telegram t.me/itinainews or Twitter @itinaicom.