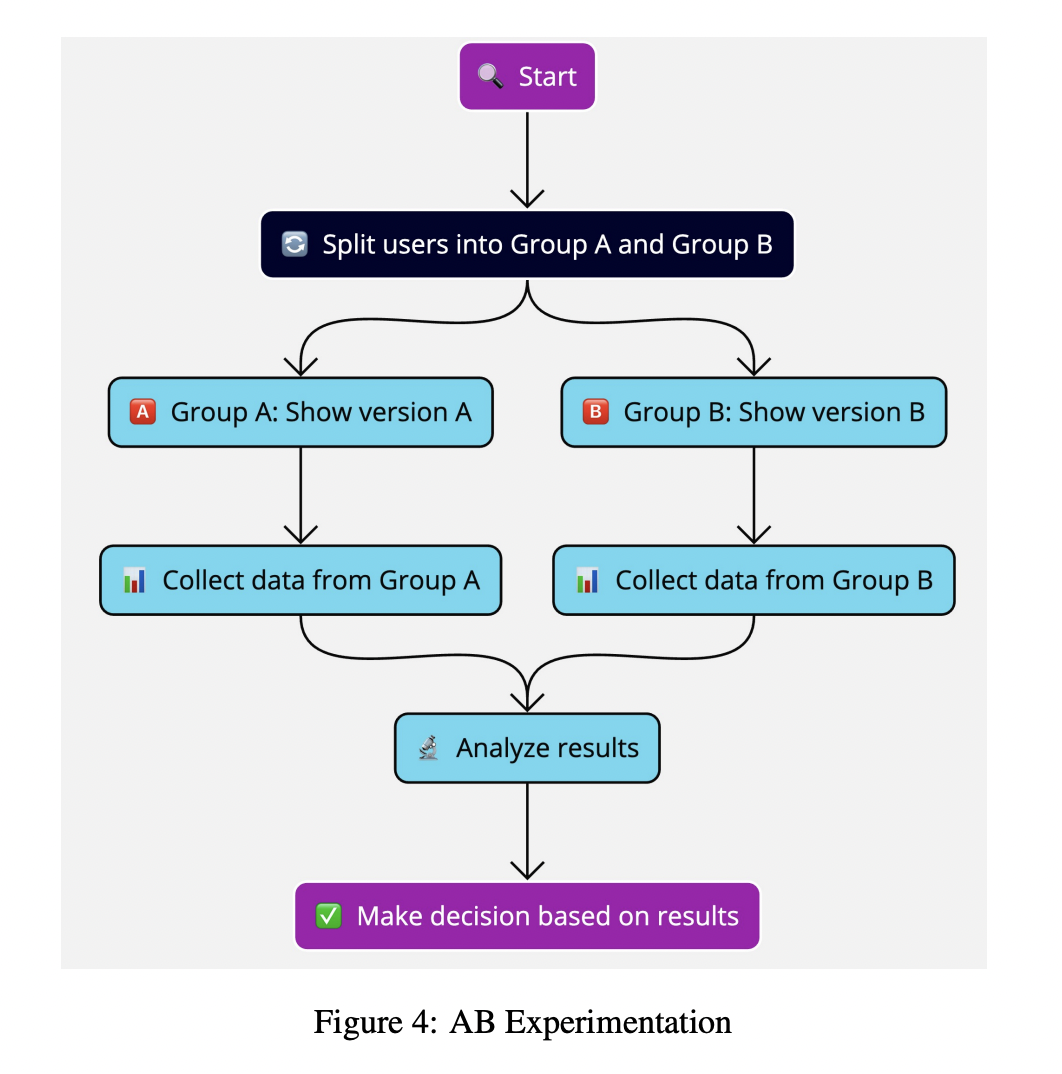
Retrieval Algorithms in Ad and Content Recommendation Systems
Practical Solutions and Value
Researchers from the University of Toronto explore advanced algorithms used in ad and content recommendation systems, highlighting their practical applications in driving user engagement and revenue generation in digital platforms.
Ad Targeting Models
Ad targeting models utilize detailed user profiles and behavioral data to deliver customized ads, maximizing user engagement and conversion rates. Strategies include machine learning, the inverted index, age and gender targeting, re-targeting, keyword targeting, and behavioral targeting.
Organic Retrieval Systems
Organic retrieval systems recommend content that matches user preferences without direct monetary influence, enhancing user experience in e-commerce, streaming services, and social media platforms. Mechanisms include content-based filtering, collaborative filtering, and hybrid systems.
Two-Tower Model
The two-tower model, a deep learning architecture widely used in recommendation systems, consists of separate neural networks for encoding user and item features. It projects users and items into a shared latent space, optimizing latent representations to reflect compatibility between users and items.
Conclusion
While retrieval algorithms enhance user engagement and drive revenue, they also present challenges like data quality and privacy concerns. Future research should focus on developing more sophisticated and ethical retrieval algorithms to meet user expectations and expand digital platforms.
Contact Us
Discover how AI can redefine your company’s way of work. Connect with us at hello@itinai.com for AI KPI management advice and continuous insights into leveraging AI.