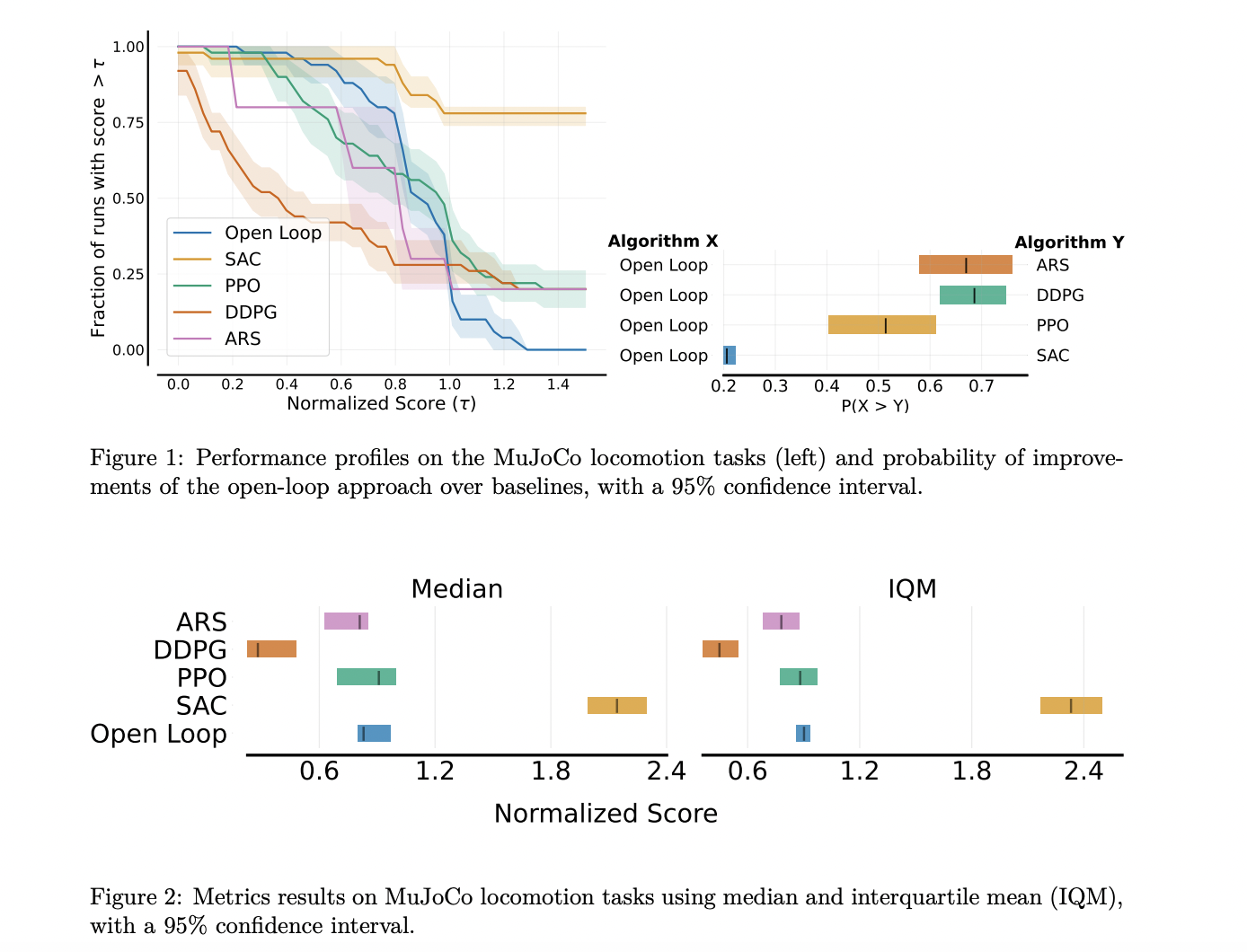
Practical Solutions and Value of A Simple Open-loop Model-Free Baseline for Reinforcement Learning Locomotion Tasks
Addressing Complexity and Fragility in Reinforcement Learning
The latest algorithms in deep reinforcement learning (DRL) have become increasingly complex, leading to issues with reproducibility and simple task performance. To combat this, researchers have proposed simpler parametrizations and periodic policies for locomotion tasks.
Value in Real-World Applications
The proposed simple, open-loop model-free baseline offers benefits such as fast computation, easy deployment on embedded systems, smooth control outputs, and robustness to sensor noise. It outperforms standard locomotion tasks and provides versatility due to its simplicity, offering practical solutions for real-world applications.
Evaluation and Comparison
The effectiveness of the proposed baseline is tested on locomotion tasks using JAX implementations and compared against established deep RL algorithms. The experiments highlight the limitations of DRL for robotic applications, providing insights and encouraging reflection on the costs of complexity and generality.
Key Questions Addressed
The paper aims to answer key questions related to the performance, resilience, and transferability of open-loop oscillators compared to DRL methods in locomotion tasks, providing valuable insights for the field.
Conclusion and Application
The open-loop model-free baseline offers practical solutions for locomotion tasks without the need for complex models or computational resources. While it outperforms DRL in certain aspects, it also highlights limitations that need to be addressed, providing valuable insights for the application of AI in robotics.
AI Solutions for Business
AI solutions can redefine work processes by identifying automation opportunities, defining KPIs, selecting suitable AI tools, and implementing AI gradually. For AI KPI management advice and insights into leveraging AI, connect with us at hello@itinai.com or follow us on Telegram and Twitter for continuous updates.