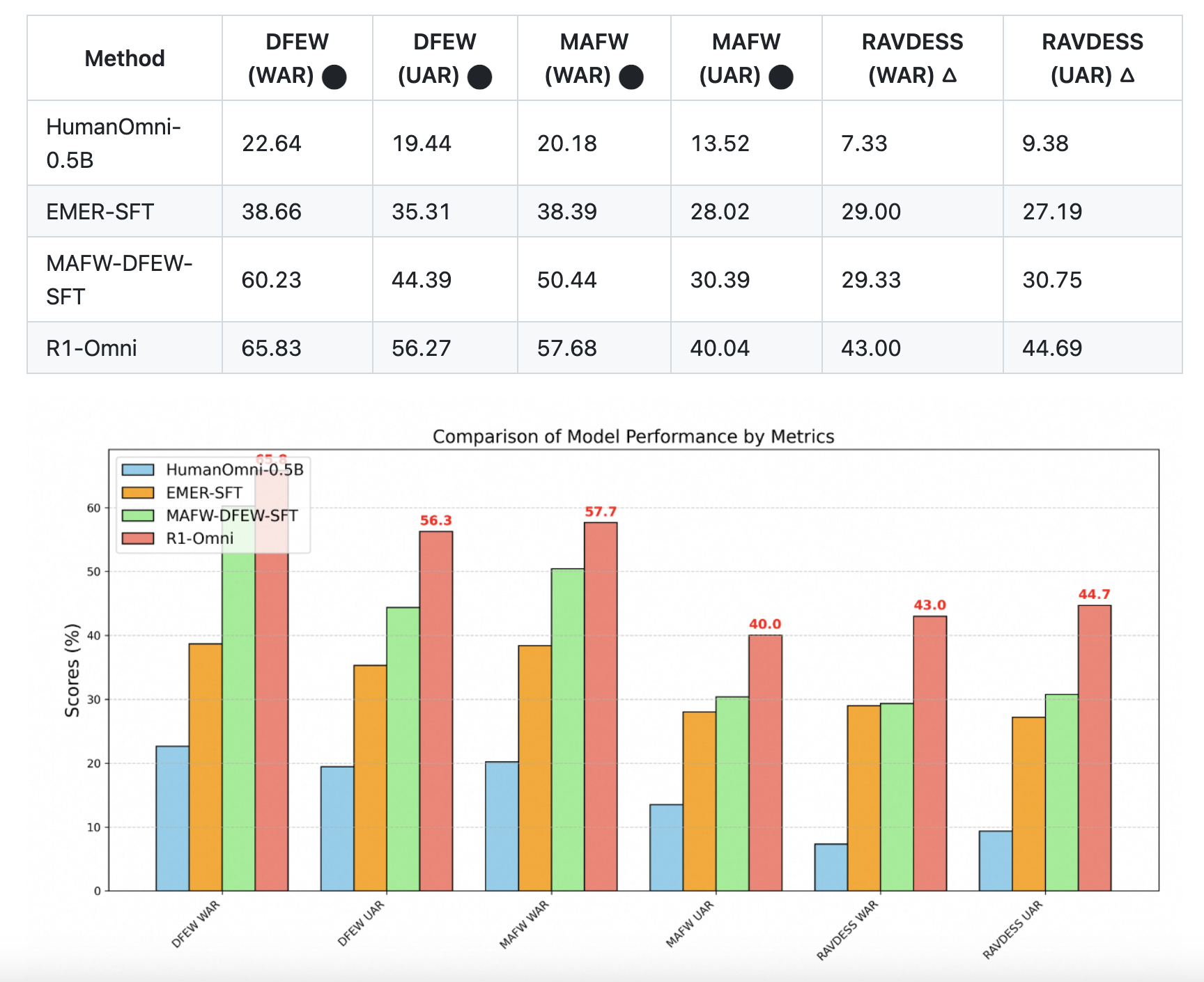
Challenges in Emotion Recognition
Emotion recognition from video poses various complex challenges. Models relying solely on visual or audio signals often overlook the intricate relationship between these modalities, resulting in misinterpretation of emotional content. A significant challenge lies in effectively combining visual cues—such as facial expressions and body language—with auditory signals like tone and intonation. Additionally, many existing systems struggle to explain their decision-making processes, making it difficult to understand how specific emotions are identified. These issues are amplified when models encounter unfamiliar scenarios, underscoring the need for a more robust and interpretable approach to multimodal emotion recognition.
Introducing R1-Omni by Alibaba Researchers
Alibaba Researchers have introduced R1-Omni, an application of Reinforcement Learning with Verifiable Reward (RLVR) designed for emotion recognition through a multimodal large language model. R1-Omni builds on the HumanOmni framework and utilizes RLVR to enhance its handling of both video and audio data. The training process starts with a cold start phase, where the model is pre-trained using a dataset from Explainable Multimodal Emotion Reasoning (EMER) alongside a manually annotated dataset. This initial training equips the model with foundational reasoning skills before it is fine-tuned using RLVR. By incorporating a rule-based reward system during training, R1-Omni is optimized not only for accurate emotion prediction but also for producing clear explanations of how visual and auditory information interact.
Technical Insights and Benefits of the Approach
R1-Omni’s design integrates Reinforcement Learning with Verifiable Rewards (RLVR) and Group Relative Policy Optimization (GRPO). RLVR eliminates the reliance on subjective human feedback, using a verifiable reward function to evaluate model output against objective criteria. The reward system is simple: the model receives a score of 1 if its emotion prediction aligns with the ground truth, and 0 otherwise. Additionally, a format reward ensures that the output maintains a specified structure, separating the reasoning from the final prediction through designated tags.
GRPO further enhances the training by comparing groups of candidate responses, enabling the model to favor those with clearer and more coherent reasoning. This approach minimizes unsupported or misaligned reasoning and improves the overall quality of predictions. Together, these strategies foster improved reasoning, a greater understanding of multimodal inputs, and enhanced performance, particularly on unseen data.
Experimental Results and Key Observations
The study includes extensive experiments comparing R1-Omni with baseline models, such as HumanOmni-0.5B and models trained with supervised fine-tuning on the EMER and MAFW-DFEW datasets. On the DFEW dataset, R1-Omni achieves an Unweighted Average Recall (UAR) of 65.83% and a Weighted Average Recall (WAR) of 56.27%, significantly surpassing other methods. Similarly, R1-Omni showcases improved accuracy on the MAFW dataset, reinforcing its ability to classify emotions effectively across various categories.
Another notable advantage of R1-Omni is its capability to generate detailed and coherent reasoning processes. The study provides visual examples demonstrating that R1-Omni’s explanations more accurately reflect the contributions of visual and audio cues to its predictions. The model also exhibits strong generalization skills when tested on the RAVDESS dataset, which features professional actors and standardized speech, indicating its adaptability to different input types while maintaining consistent performance.
Concluding Thoughts and Future Directions
In conclusion, R1-Omni offers a promising solution to the challenges of multimodal emotion recognition. By leveraging Reinforcement Learning with Verifiable Rewards, the model not only achieves greater predictive accuracy but also articulates the reasoning behind its decisions. This approach addresses critical issues in the field, such as the integration of multimodal data and the interpretability of model outputs.
Despite its advancements, R1-Omni faces ongoing challenges, including enhancing subtitle recognition and reducing instances of unsupported reasoning. Future research may focus on improving the model’s underlying architecture, refining audio cue integration, and deepening reasoning capabilities to better reflect human emotional understanding.
R1-Omni presents a balanced approach, blending technical excellence with the necessity for interpretability, and contributes valuable insights toward the progression of transparent and effective multimodal emotion recognition systems.
For more information, check out the Paper and GitHub Page. All credit for this research goes to the researchers of this project. Feel free to follow us on Twitter and join our 80k+ ML SubReddit.
Explore how artificial intelligence technology can transform your business approach. Identify processes that can be automated and find opportunities where AI can add the most value. Establish key performance indicators (KPIs) to ensure your AI investment positively impacts your business. Choose tools that align with your goals and allow for customization. Begin with a small project, collect data on its effectiveness, and gradually expand your AI initiatives.
If you require assistance with managing AI in business, contact us at hello@itinai.ru.