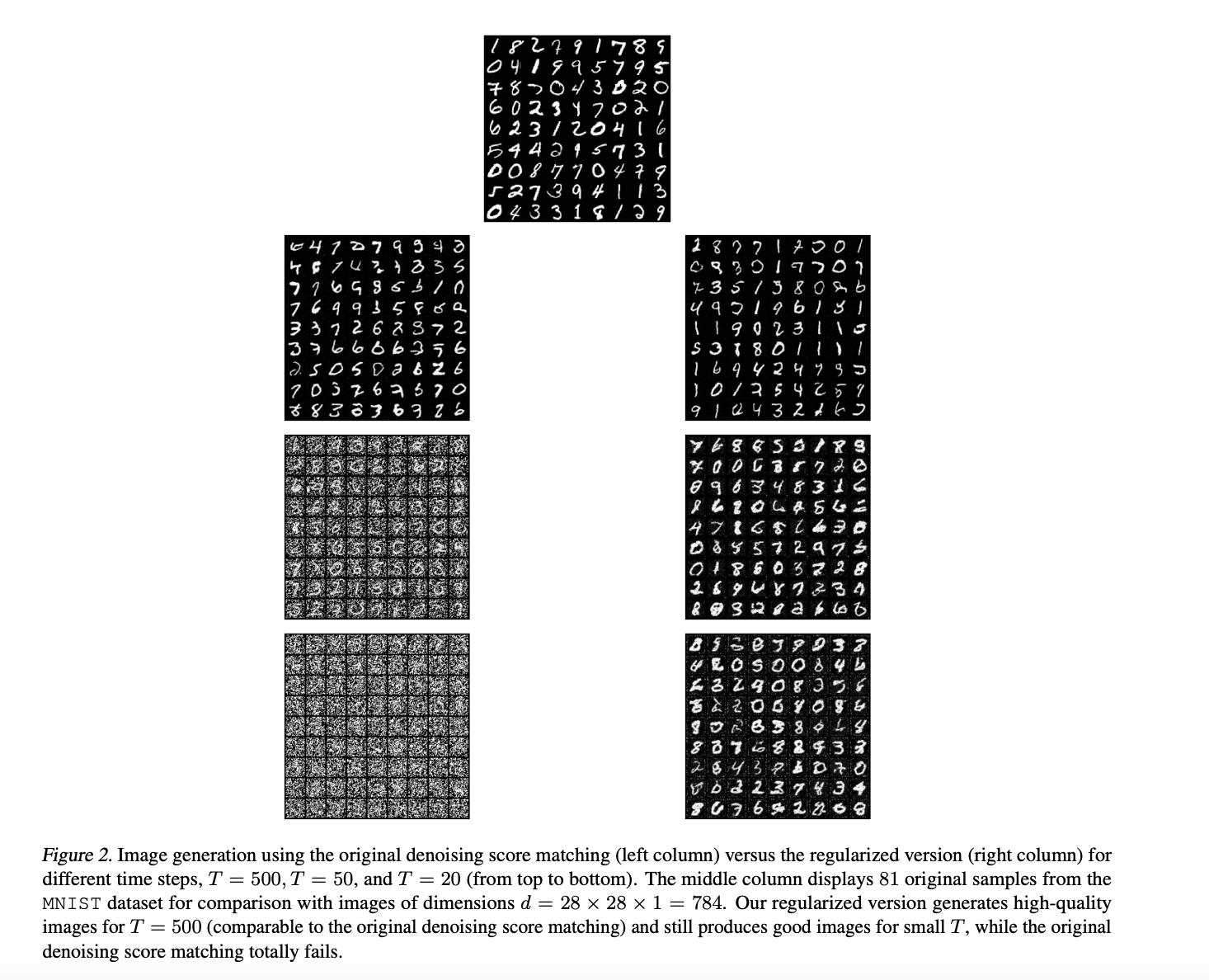
Understanding Diffusion Models in Generative AI
Diffusion models are essential in generative AI, excelling in creating images, videos, and translating text to images. They work through two processes:
1. Forward Process:
This process adds noise to data, turning it into random Gaussian noise.
2. Reverse Process:
This process learns to remove the noise and reconstructs the original data.
Key types of diffusion models include:
- Denoising Diffusion Probabilistic Models (DDPM): Uses Markov chains for gradual noise removal.
- Score-Based Generative Models (SGM): Estimates score functions to guide sampling.
- Score-Based Stochastic Differential Equations (SDEs): Extends these techniques to continuous-time diffusion.
Improving Efficiency in Diffusion Models
Recent studies focus on enhancing the efficiency of diffusion models, especially concerning their dependence on data dimensions. Traditional methods struggle with large-scale applications due to high computational costs. New approaches aim to:
- Assume accurate score estimates and smoothness properties.
- Use techniques like underdamped Langevin dynamics to improve performance.
- Employ ordinary differential equations (ODEs) to refine convergence rates.
The Role of Sparsity and Regularization
Researchers have found that applying sparsity can significantly boost the efficiency of diffusion models. By using ℓ1-regularization, they reduce computational complexity, leading to better convergence rates. Key benefits include:
- Improved sample quality and reduced over-smoothing.
- Enhanced structure in generated samples, even with fewer sampling steps.
- More realistic outputs in fashion-related datasets compared to traditional methods.
Practical Solutions for Businesses
To leverage AI effectively in your business, consider the following steps:
- Identify Automation Opportunities: Find customer interaction points that can benefit from AI.
- Define KPIs: Ensure measurable impacts on business outcomes.
- Select an AI Solution: Choose tools that fit your needs and allow customization.
- Implement Gradually: Start small, gather data, and expand as needed.
For more insights and support in implementing AI, contact us at hello@itinai.com. Stay updated with our latest news on Telegram or follow us on @itinaicom.
Explore how AI can transform your sales processes and customer engagement at itinai.com.