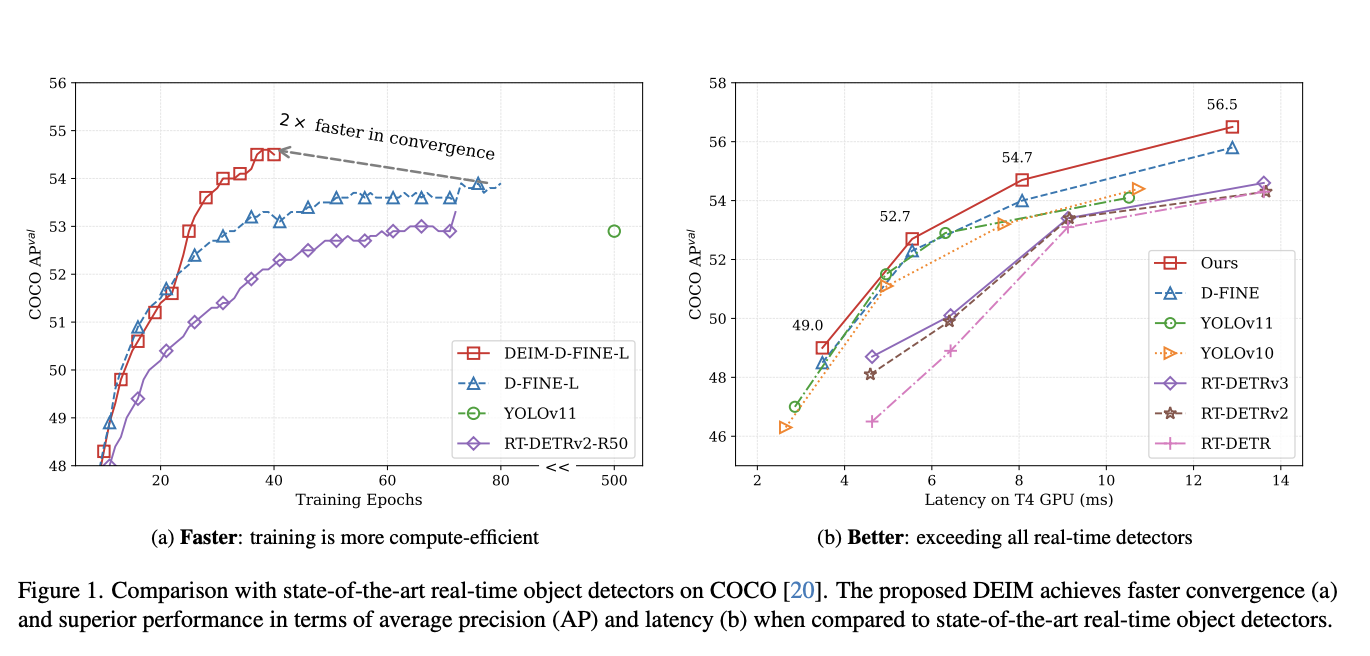
Understanding Transformer-Based Detection Models
Why Choose Transformer Models?
Transformer-based detection models are becoming popular because they match objects one-to-one. Unlike traditional models like YOLO, which need extra steps to reduce duplicate detections, DETR models use advanced algorithms to directly link detected objects to their true positions. This means no extra processing is needed, making them faster and more stable.
Challenges with Current Models
Despite their advantages, DETR models face issues like slow learning speeds. Recent studies show that this is mainly due to:
- Sparse Supervision: Only one positive sample is assigned per target, limiting the learning potential, especially for small objects.
- Low-Quality Matches: The few queries in DETR often do not align well with targets, leading to poor detection accuracy.
Innovative Solutions from Intellindust AI Lab
Researchers have developed a new method called DEIM, which enhances the DETR framework for faster learning. This approach combines two key strategies:
- Dense O2O: Increases the number of targets in each training image, resulting in more positive samples without extra computational costs.
- Matchability Aware Loss (MAL): Improves the quality of matches by adjusting penalties based on how well the detected boxes align with the actual targets.
How Does Dense O2O Work?
Dense O2O works by splitting an image into four parts and then merging them back together. This process increases the number of targets while keeping the matching structure intact. MAL simplifies the loss function, ensuring a balanced focus on both high and low-quality matches.
Proven Results
When DEIM was tested with various models, it consistently outperformed others in terms of training costs, speed, and accuracy. For instance, the latest DETR model, D-FINE, achieved a significant improvement in small object detection and reduced training costs by 30% when DEIM was applied.
Conclusion
DEIM offers a straightforward solution to the slow learning problem in DETR models, especially excelling in small object detection with fewer training epochs.
Get Involved
For more insights, check out the Paper and GitHub Page. Follow us on Twitter, join our Telegram Channel, and connect with our LinkedIn Group. Join our 60k+ ML SubReddit community!
Transform Your Business with AI
Stay competitive by leveraging DEIM for faster and more accurate object detection. Here’s how to get started:
- Identify Automation Opportunities: Find areas in customer interactions that can benefit from AI.
- Define KPIs: Ensure your AI initiatives have measurable impacts.
- Select an AI Solution: Choose tools that fit your needs and allow customization.
- Implement Gradually: Start small, gather data, and expand wisely.
For AI KPI management advice, contact us at hello@itinai.com. For ongoing insights, follow us on Telegram or @itinaicom.
Discover how AI can enhance your sales processes and customer engagement at itinai.com.