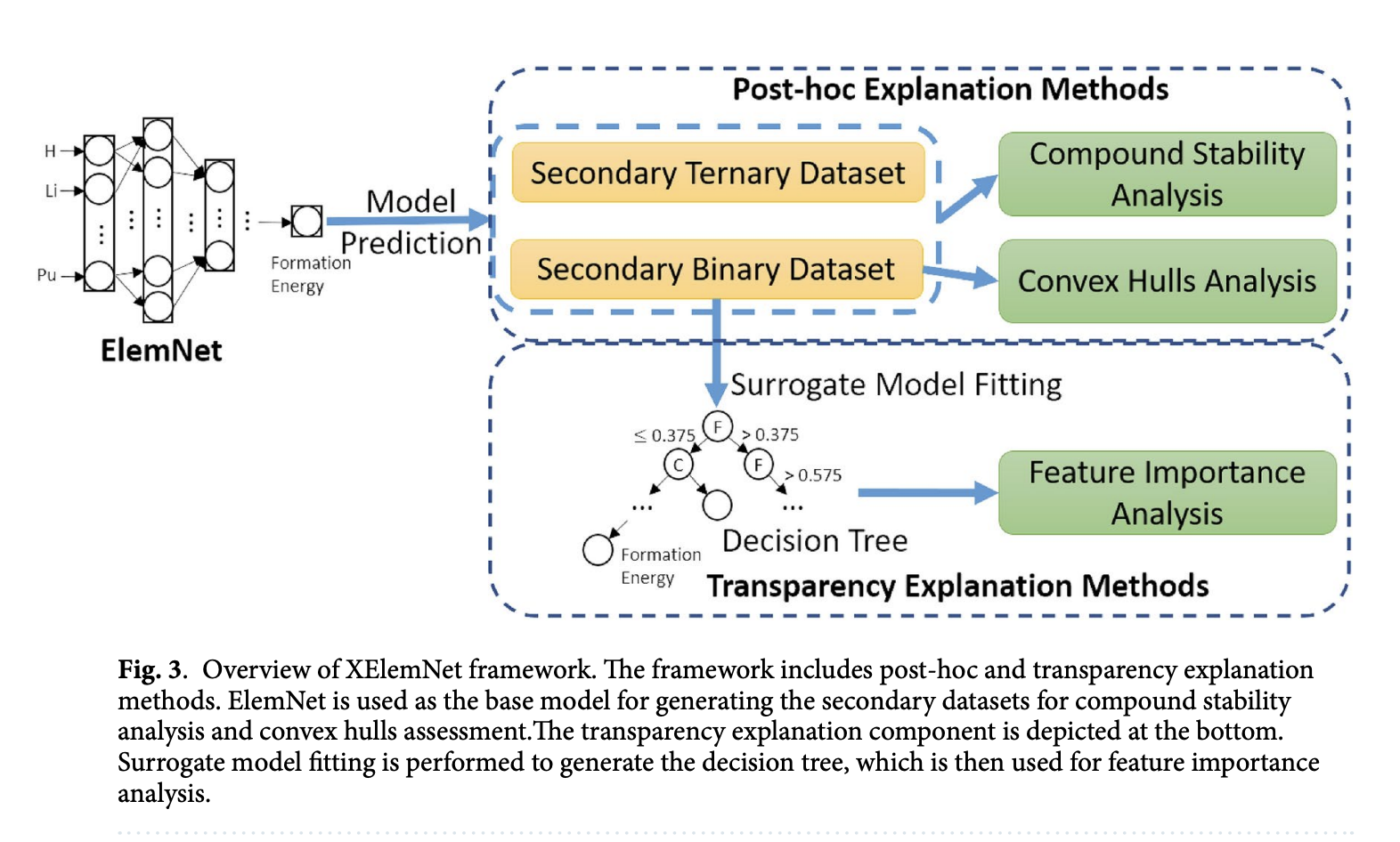
Advancements in Deep Learning for Material Sciences
Transforming Material Design
Deep learning has greatly improved material sciences by predicting material properties and optimizing compositions. This technology speeds up material design and allows for exploration of new materials. However, the challenge is that many deep learning models are ‘black boxes,’ making it hard to understand their predictions.
XElemNet: A Solution for Explainability
Researchers at Northwestern University developed XElemNet, which focuses on explainable AI (XAI) methods to make processes clearer. This model helps researchers to trust AI predictions in material discovery.
How XElemNet Works
XElemNet uses explainable AI techniques, particularly layer-wise relevance propagation (LRP). It employs two main strategies:
- Post-hoc Analysis: This technique uses a secondary dataset to analyze feature relationships. For example, convex hull analysis visualizes how the model predicts compound stability.
- Transparency Explanations: Decision trees approximate the behavior of the deep learning network, providing insights into the model’s decision-making process.
Benefits of XElemNet
This approach enhances predictive accuracy and offers valuable insights into material properties. It addresses the need for trust in AI technologies, which is crucial for their practical application in materials science.
Conclusion
XElemNet tackles the challenge of explainability in AI for materials science, combining robust validation and innovative analysis techniques. While there are still technical challenges, such as ensuring generalizability across datasets, the model represents a significant step toward trustworthy AI applications.
Get Involved
Explore the research paper for more details. Follow us on Twitter, join our Telegram Channel, and connect on LinkedIn. If you appreciate our work, subscribe to our newsletter and join our 55k+ ML SubReddit community.
Unlock AI’s Potential for Your Business
Stay competitive by leveraging XElemNet in your company. Here’s how:
- Identify Automation Opportunities: Find customer interaction points that can benefit from AI.
- Define KPIs: Make sure your AI initiatives have measurable impacts.
- Select an AI Solution: Choose tools that fit your needs and allow customization.
- Implement Gradually: Start with a pilot project, collect data, and expand carefully.
For AI KPI management advice, contact us at hello@itinai.com. For ongoing AI insights, follow us on Telegram at t.me/itinainews or Twitter at @itinaicom.
Enhance Your Sales and Customer Engagement with AI
Discover more solutions at itinai.com.