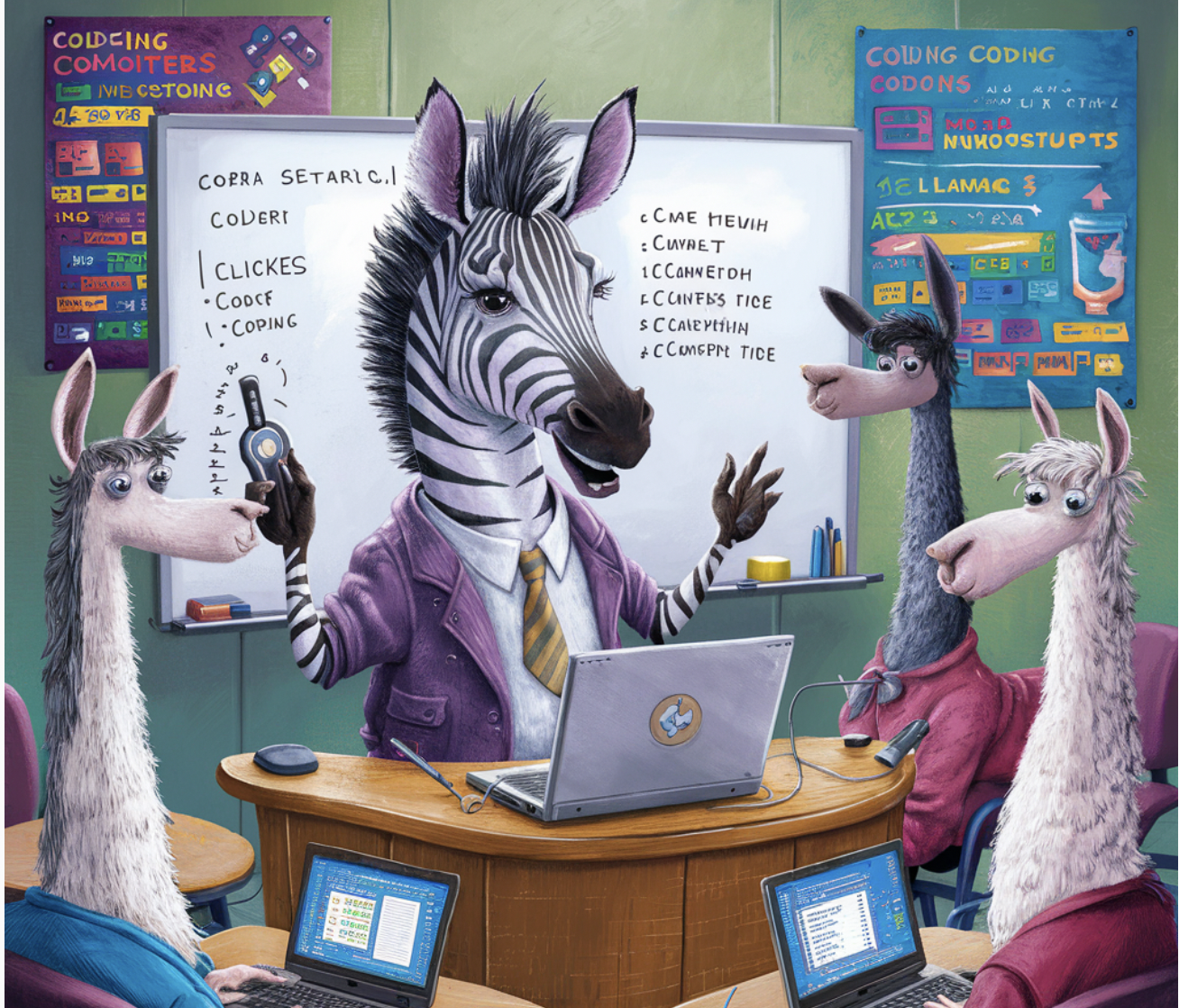
Practical Solutions and Value of ZebraLogic: A Logical Reasoning AI Benchmark
Overview
Large language models (LLMs) demonstrate proficiency in information retrieval, creative writing, mathematics, and coding. ZebraLogic evaluates LLMs’ logical reasoning capabilities through Logic Grid Puzzles, a Constraint Satisfaction Problem (CSP) commonly used in assessments like the Law School Admission Test (LSAT).
Challenges Addressed
LLMs struggle with complex logical reasoning, lacking crucial abilities such as counterfactual thinking, reflective reasoning, structured memorization, and compositional generalization.
Practical Solutions
ZebraLogic comprises 1,000 programmatically generated puzzles, ranging from 2×2 to 6×6 in size, enabling consistent evaluation of LLMs’ logical reasoning abilities. The puzzle creation process involves systematic steps, including defining features, establishing clue types, generating solutions, and formatting puzzles for LLM input.
Value
The study uses puzzle-level and cell-wise accuracy metrics, comparing LLM performance to random guessing probabilities. The research provides insights into the challenges of logical reasoning for AI systems and offers practical advice for companies looking to evolve with AI.
AI Solutions for Companies
Identify Automation Opportunities, Define KPIs, Select an AI Solution, and Implement Gradually to leverage AI for business advantage.
Connect with Us
For AI KPI management advice, connect with us at hello@itinai.com. Stay tuned on our Telegram t.me/itinainews or Twitter @itinaicom for continuous insights into leveraging AI.
Explore AI Solutions
Discover how AI can redefine your sales processes and customer engagement at itinai.com.