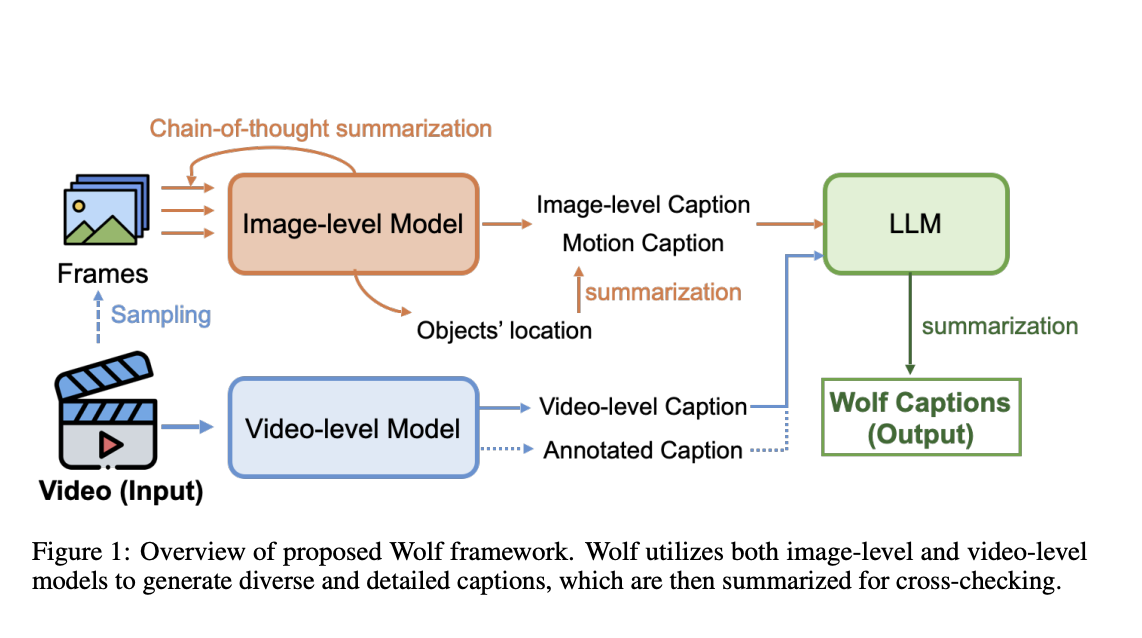
Practical Solutions and Value in AI Video Captioning
Challenges in Video Captioning
Generating accurate, detailed video captions is challenging due to the scarcity of high-quality data, temporal complexities, and the critical need for correctness in safety-critical applications.
Recent Advancements
Recent advancements in visual language models have led to the development of video-specific models like PLLaVa, Video-llava, and Video-LLama to address these challenges.
Wolf: A WOrLd Summarization Framework
Researchers have proposed Wolf, a framework that outperforms current state-of-the-art methods and commercial solutions, significantly boosting caption quality in challenging driving videos.
Wolf’s Evaluation and Results
Wolf outperforms other methods in video captioning, particularly excelling in challenging scenarios like multiview driving videos.
Conclusion and Future Plans
Wolf represents a significant advancement in automated video captioning, providing detailed and correct descriptions. Researchers have established a leaderboard to encourage competition and innovation in video captioning technology.
AI Integration and KPI Management
For companies looking to evolve with AI, Wolf can redefine their way of work by identifying automation opportunities, defining KPIs, selecting AI solutions, and implementing AI gradually.