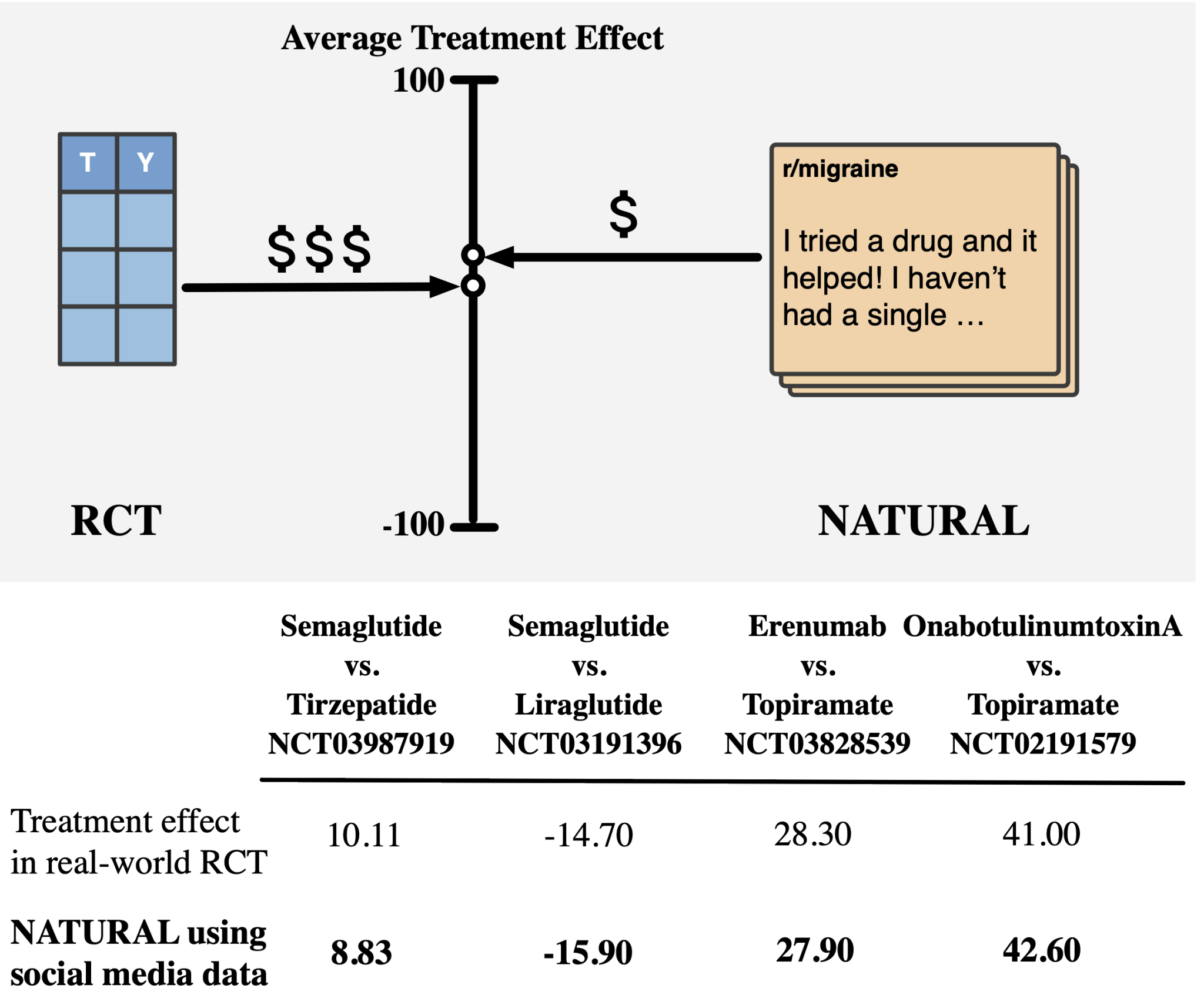
Causal Effect Estimation with NATURAL: Revolutionizing Data Analysis
Understanding Impact and Practical Solutions
Causal effect estimation is vital for comprehending intervention impacts in areas like healthcare, social sciences, and economics. Traditional methods are time-consuming and costly, hindering the scope and efficiency of data analysis.
Practical Solution: NATURAL leverages large language models to analyze unstructured text data, automating data curation and providing a scalable solution for various applications.
Methodology and Value
NATURAL utilizes large language models to process natural language text and estimate conditional variable distributions, mimicking traditional causal inference techniques but operating on unstructured data.
Value: The method demonstrated remarkable accuracy, predicting outcomes with a mean absolute error of 2.5% and achieving high consistency with clinical trial results. The cost of computational analysis is significantly lower compared to traditional methods.
Transformative Potential
NATURAL’s ability to accurately estimate causal effects from unstructured data suggests a transformative potential for fields reliant on causal analysis, significantly reducing time and cost associated with traditional techniques.
Revolutionary Solution: NATURAL presents a groundbreaking approach to causal effect estimation, automating data curation and leveraging large language models to open new avenues for utilizing rich, unstructured data sources.
Evolve Your Company with AI
Discover how AI can redefine your way of work, identify automation opportunities, define KPIs, select an AI solution, and implement gradually to stay competitive.
AI Redefinition: Connect with us for AI KPI management advice and continuous insights into leveraging AI for sales processes and customer engagement.