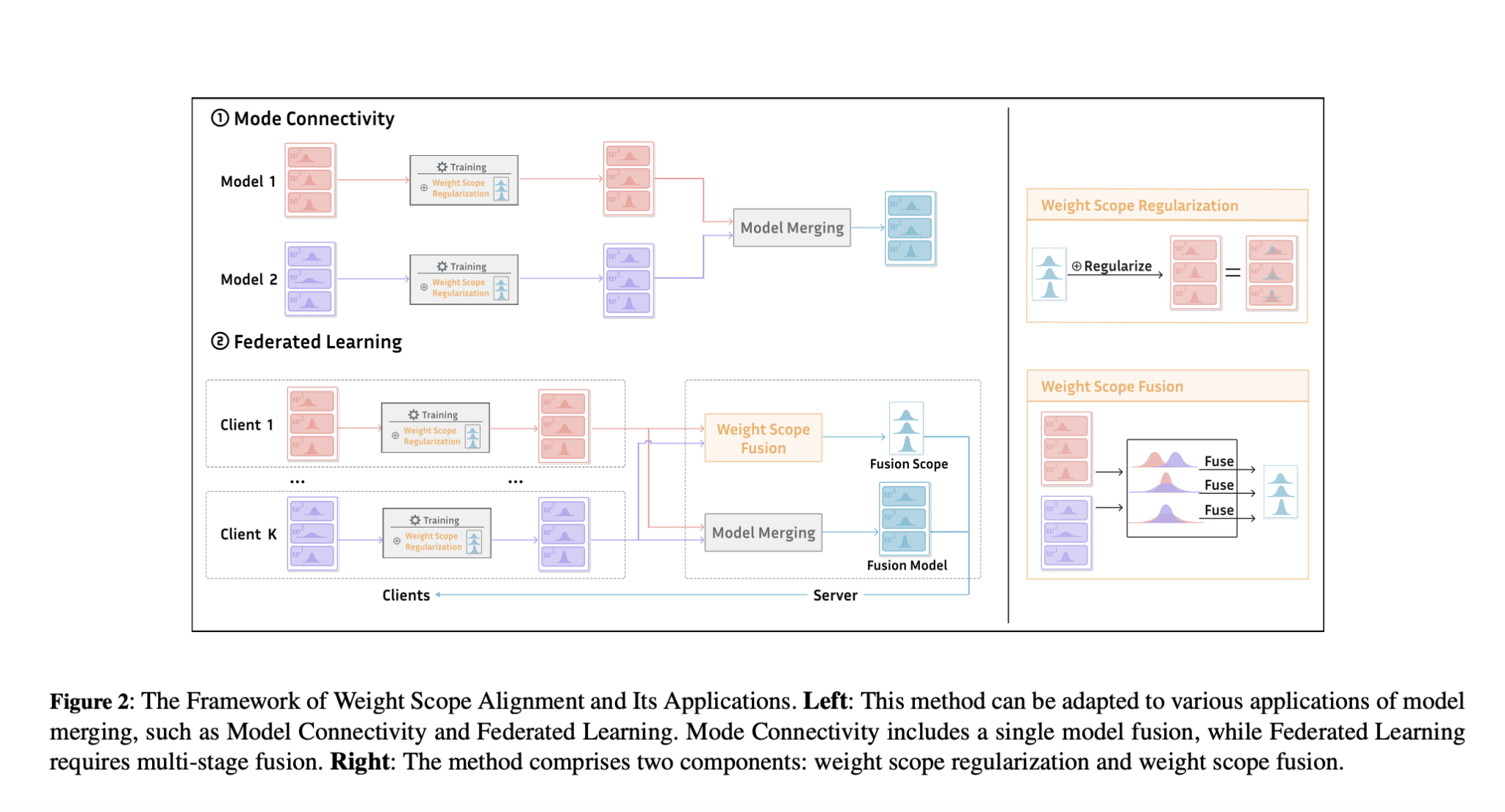
Model Fusion and Weight Scope Alignment in AI
Practical Solutions and Value
Model fusion involves merging multiple deep models into one, enhancing generalizability, efficiency, and robustness while preserving the original models’ capabilities. This process is crucial in various applications, especially in federated learning and mode connectivity research.
Coordinate-based parameter averaging is the preferred method for model fusing in deep neural networks. However, it may face challenges with Non-Independent and Identically Distributed (Non-I.I.D.) data or different training conditions, leading to diverging update orientations and neuron misalignment.
A new study explores the impact of weight scope on model fusion, identifying a phenomenon called ‘weight scope mismatch’. This mismatch significantly affects model fusion, making it difficult to aggregate parameters with dissimilar distributions.
The proposed Weight Scope Alignment (WSA) approach addresses these challenges by aligning the weight scopes of merged models, optimizing for successful model fusion while balancing specificity and generality. It competes with existing regularization methods and provides valuable insights for researchers and practitioners in the field.
For AI KPI management advice and continuous insights into leveraging AI, connect with us at hello@itinai.com or follow us on Telegram or Twitter.
Evolve Your Company with AI
Utilize the Weight Scope Alignment Method to redefine your way of work, stay competitive, and discover how AI can redefine your sales processes and customer engagement. Identify automation opportunities, define KPIs, select an AI solution, and implement gradually to leverage AI for your advantage.
Explore AI solutions at itinai.com.