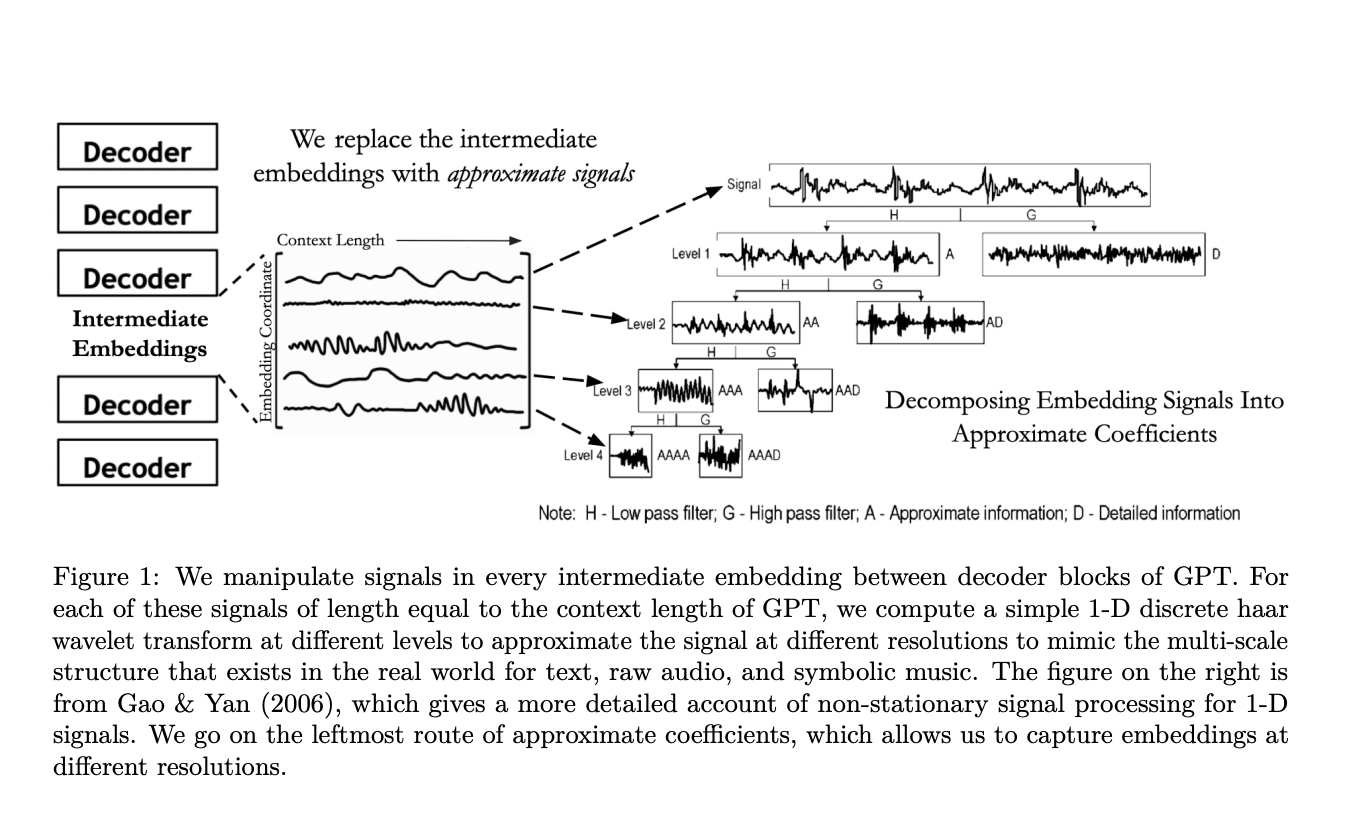
Practical Solutions and Value of WaveletGPT for AI Evolution
Enhancing Large Language Models with Wavelets
WaveletGPT introduces wavelets into Large Language Models to improve performance without extra parameters. This accelerates training by 40-60% across diverse modalities.
Wavelet-Based Intermediate Operation
Wavelet transform adds multi-scale filters to intermediate embeddings, enabling access to multi-resolution representations at every layer. This boosts model performance significantly.
Improved Training Efficiency
WaveletGPT speeds up pre-training of transformer-based models without complexity. It achieves performance gains comparable to adding layers or parameters, enhancing efficiency for AI development.
Multi-Modal Performance Enhancements
Wavelet-based operation shows performance boosts in language, audio, and music datasets, emphasizing its versatility. Learnable wavelet kernels further enhance model capabilities.
Key Implementation Steps
1. Incorporate wavelets into LLM architecture.
2. Apply discrete wavelet transform for multi-scale filters.
3. Use Haar wavelets for structured data representation.
4. Maintain causality assumption for effective next-token prediction.
5. Enhance model performance while simplifying architecture.
Future AI Optimization
Explore advanced wavelet concepts for further optimizing large language models. WaveletGPT paves the way for leveraging wavelet theory in AI evolution across various industries.