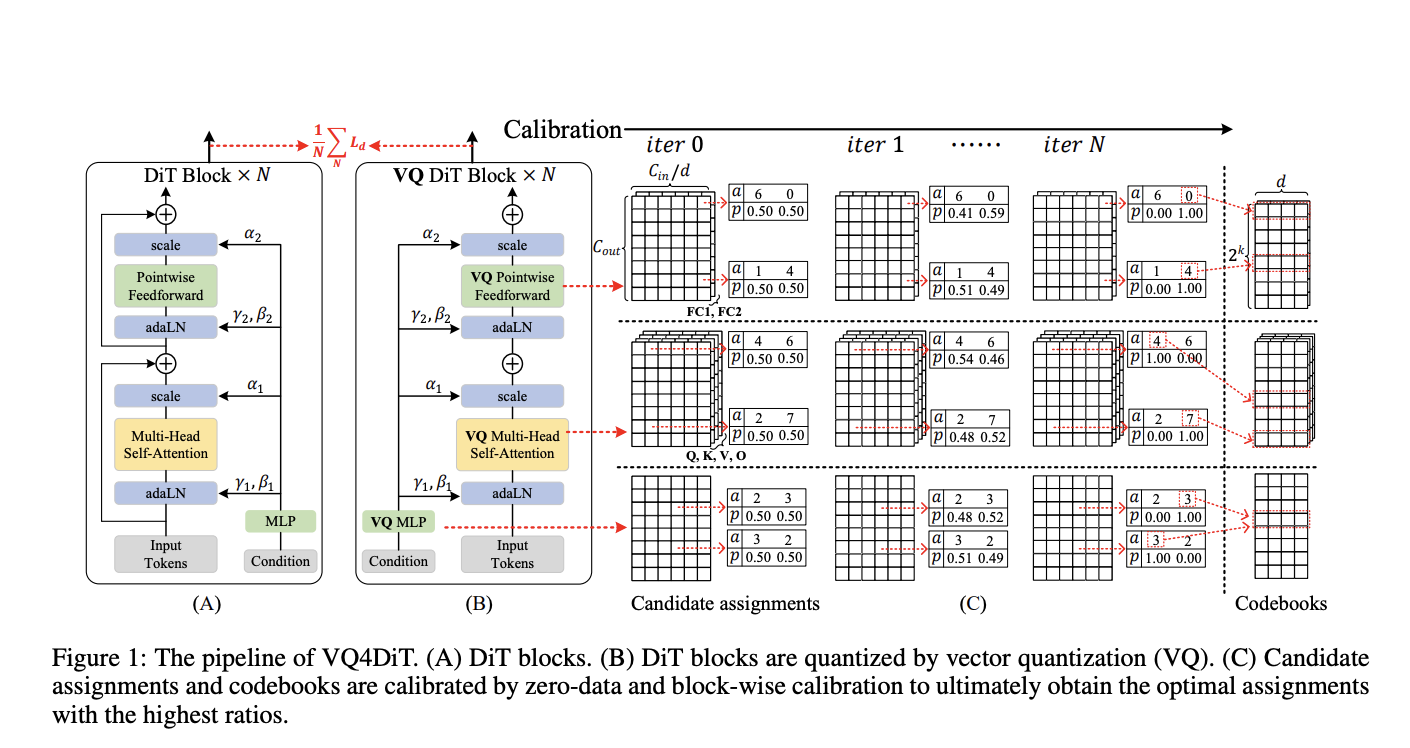
Practical Solutions for Diffusion Transformers Models
Challenges in Deployment and Efficient Quantization
Text-to-image diffusion models like Diffusion Transformers Models (DiTs) have shown impressive results in generating high-quality images. However, their large parameter count and computational complexity pose challenges for deployment on edge devices with limited resources.
Efficient Post-Training Vector Quantization for DiTs
Efforts to address these challenges have led to the development of VQ4DiT, a method that efficiently and accurately quantizes DiTs without needing a calibration dataset. VQ4DiT balances codebook size with quantization error and resolves inconsistent gradient directions, achieving optimal assignments and codebooks through a zero-data and block-wise calibration process.
Performance of VQ4DiT
When applied to the DiT XL/2 model, VQ4DiT demonstrates superior performance on ImageNet datasets, maintaining high-quality image generation capabilities even at 2-bit precision. This advancement significantly enhances the potential for deploying DiTs on resource-constrained edge devices.
Value of VQ4DiT for AI Solutions
AI Transformation and Automation
VQ4DiT offers a fast post-training vector quantization method for DiTs, enabling companies to leverage AI for automation and redefine their way of work. It provides practical steps for identifying automation opportunities, defining KPIs, selecting AI solutions, and implementing AI usage judiciously.
AI-Powered Sales Processes and Customer Engagement
Businesses can also explore the potential of AI in redefining sales processes and customer engagement through solutions offered at itinai.com.
Connect with Us
For AI KPI management advice and continuous insights into leveraging AI, connect with us at hello@itinai.com. Stay tuned on our Telegram Channel or Twitter for more updates.