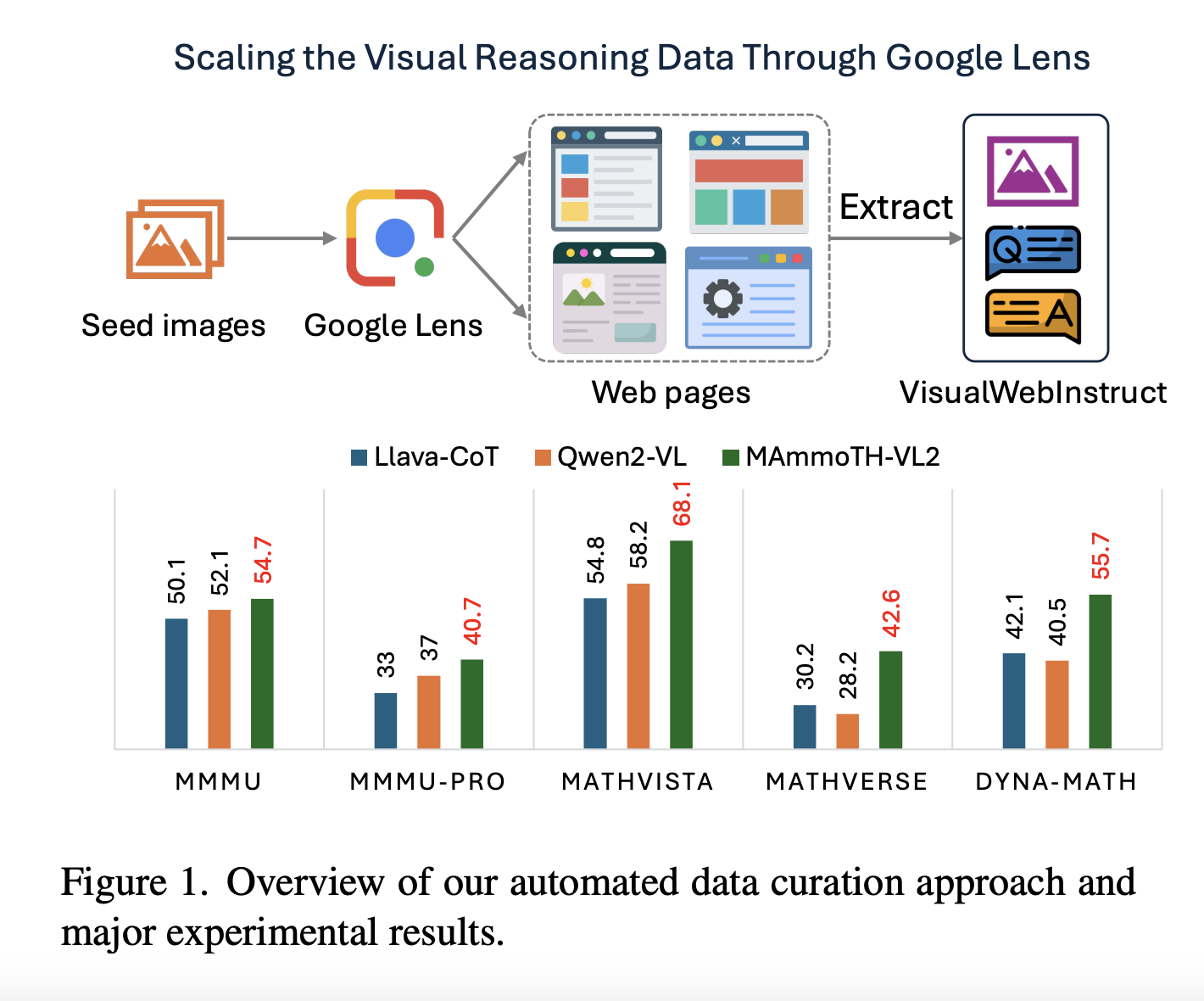
Introduction to Visual Language Models (VLMs)
Visual language models (VLMs) have made significant strides in perception-driven tasks like visual question answering and document-based visual reasoning. However, their performance in reasoning-intensive tasks is limited by the lack of high-quality, diverse training datasets.
Challenges in Current Multimodal Datasets
Existing multimodal reasoning datasets face several issues: some are overly specialized in scientific imagery, others depend on synthetic data which lacks real-world applicability, and many are too small or simplistic to develop robust reasoning skills. These limitations hinder VLMs in tackling complex multi-step reasoning tasks.
Automating Data Collection
Given the difficulties of manual data annotation at scale, researchers are exploring automated data mining methods. Inspired by the WebInstruct methodology, new efforts aim to apply similar techniques to multimodal reasoning. However, the current lack of large-scale multimodal datasets and retrieval model constraints present challenges to this approach.
Strategies for Advancing Multimodal Reasoning
Various strategies have been proposed to enhance multimodal reasoning, including neural symbolic reasoning, optimized visual encoding, and structured reasoning frameworks. While proprietary models like GPT-4o and Gemini showcase top-tier performance, the limited access has led to the creation of open-source alternatives such as LLaVA and MiniGPT-4.
Improvements in Reasoning Techniques
One technique that has notably improved reasoning capabilities in large language models (LLMs) is Chain-of-Thought (CoT) prompting. This method breaks complex queries into manageable steps, enhancing logical inference. Models like Prism and MSG have adopted this structured approach to refine perception-reasoning pipelines. Nevertheless, the scarcity of large supervised datasets for multimodal reasoning remains a key challenge.
Introduction of VisualWebInstruct Dataset
Researchers from esteemed institutions have introduced VisualWebInstruct, a large-scale multimodal reasoning dataset aimed at improving VLMs. By utilizing Google Image Search, they compiled 30,000 seed images from various disciplines and retrieved over 700,000 web pages to generate 900,000 question-answer pairs, with a significant portion being visual.
Data Mining Pipeline Overview
The data mining pipeline starts with 30,000 scientific images and collects nearly 760,000 unique URLs, excluding non-educational sources. The process includes constructing accessibility trees to extract relevant text and images. The Gemini 1.5 Flash model filters quality question-answer pairs, which are then refined with GPT-4o for consistency, resulting in a comprehensive dataset.
MAmmoTH-VL2 Model Development
The MAmmoTH-VL2 model was fine-tuned using the VisualWebInstruct dataset, showcasing advanced architecture and training methodologies. Evaluated across seven multimodal reasoning benchmarks, it outperformed many similar open-source models, particularly in mathematical reasoning tasks. An ablation study confirmed that integrating VisualWebInstruct with existing frameworks led to optimal results.
Conclusions and Future Directions
This study highlights the potential of building large-scale multimodal reasoning datasets without requiring human annotation. The VisualWebInstruct method employs search engines to create a rich dataset across various fields, resulting in significant performance enhancements for models trained using it.
Next Steps for Businesses
To harness the benefits of artificial intelligence, organizations can:
- Explore how AI technologies like VisualWebInstruct can transform business operations.
- Identify processes that can be automated for improved efficiency.
- Establish key performance indicators (KPIs) to assess the impact of AI investments.
- Select tools that are customizable to meet specific business needs.
- Initiate small AI projects, monitor their effectiveness, and gradually scale up.
Contact Us
For guidance on managing AI in your business, connect with us at hello@itinai.ru. You can also reach us on Telegram, X, or LinkedIn.