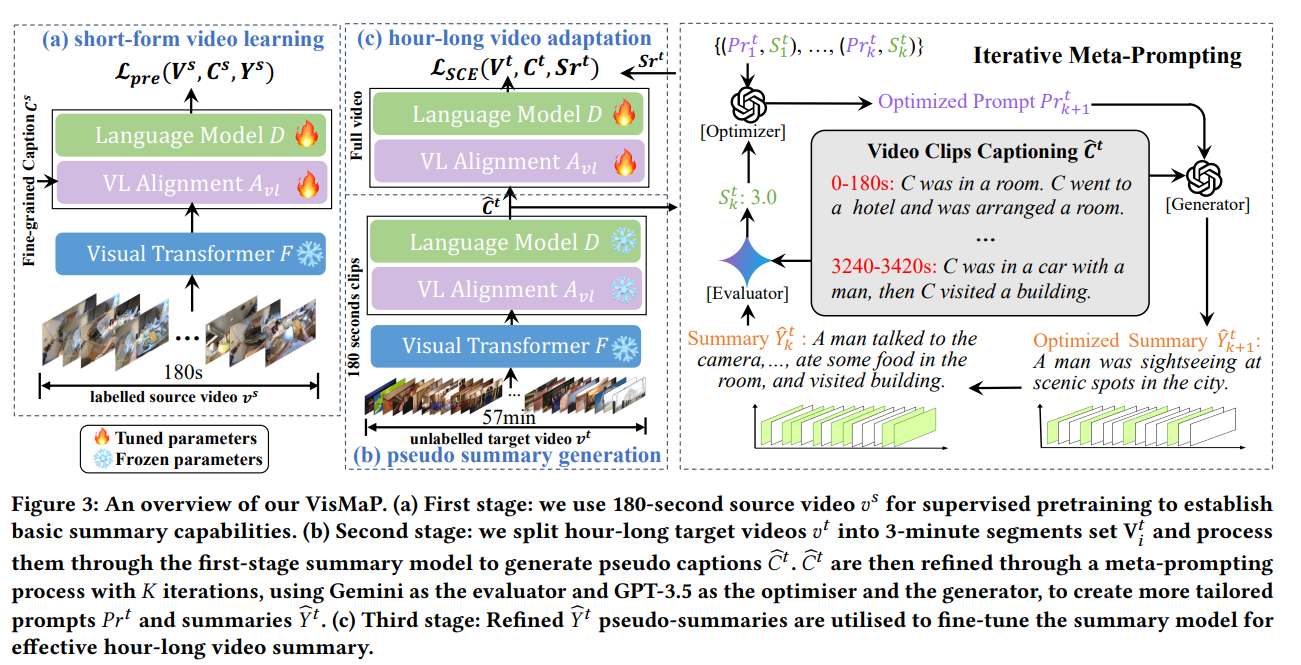
ViSMaP: Unsupervised Summarization of Long Videos
Understanding the Challenge of Video Captioning
Video captioning has evolved significantly; however, existing models typically excel with short videos, often under three minutes. These models can describe basic actions but struggle with the complexity inherent in hour-long videos such as vlogs, sports events, and films. Traditional models tend to generate fragmented descriptions, failing to convey the overarching narrative. Although tools like MA-LMM and LaViLa have made strides in handling longer clips, hour-long videos remain underrepresented due to a lack of appropriate datasets.
The Gap in Current Solutions
- Ego4D: Introduced a large dataset of hour-long videos, but its first-person perspective limits broader application.
- Video ReCap: Utilizes multi-granularity annotations for hour-long videos, but this method is costly and inconsistent.
- Short-Form Datasets: Widely available and more user-friendly, yet they do not effectively address the needs of long-form video summarization.
Introducing ViSMaP
Researchers from Queen Mary University and Spotify have developed ViSMaP, an innovative unsupervised method for summarizing hour-long videos without the need for expensive annotations. This approach leverages large language models (LLMs) and meta-prompting strategies to generate and refine pseudo-summaries from existing short-form video descriptions.
Process Overview
ViSMaP’s methodology includes three phases using sequential LLMs:
- Generation: Producing initial summaries from video clip descriptions.
- Evaluation: Assessing the quality of the generated summaries.
- Optimization: Refining the summaries for improved accuracy.
This iterative process achieves results comparable to fully supervised models while minimizing the need for extensive manual labeling.
Evaluating ViSMaP’s Performance
ViSMaP was evaluated across multiple scenarios, including:
- Summarization using Ego4D-HCap data.
- Cross-domain generalization on datasets such as MSRVTT, MSVD, and YouCook2.
- Adaptation for short videos using EgoSchema.
Results show that ViSMaP outperforms or matches various supervised and zero-shot methods while utilizing metrics such as CIDEr, ROUGE-L, METEOR scores, and question-answering accuracy.
Future Directions and Innovations
While ViSMaP demonstrates remarkable adaptability and effectiveness, it continues to rely exclusively on visual information. Future advancements could incorporate:
- Multimodal data integration for enhanced context.
- Hierarchical summarization techniques for more nuanced results.
- Developing more generalizable meta-prompting strategies.
Conclusion
In summary, ViSMaP represents a significant advancement in the unsupervised summarization of long videos, effectively utilizing existing short-form datasets and innovative meta-prompting strategies. Its competitive performance against fully supervised methods highlights its potential for widespread application across various video domains. As further developments occur, integrating multimodal data and refining summarization techniques could lead to even greater efficiencies and insights in video content analysis.