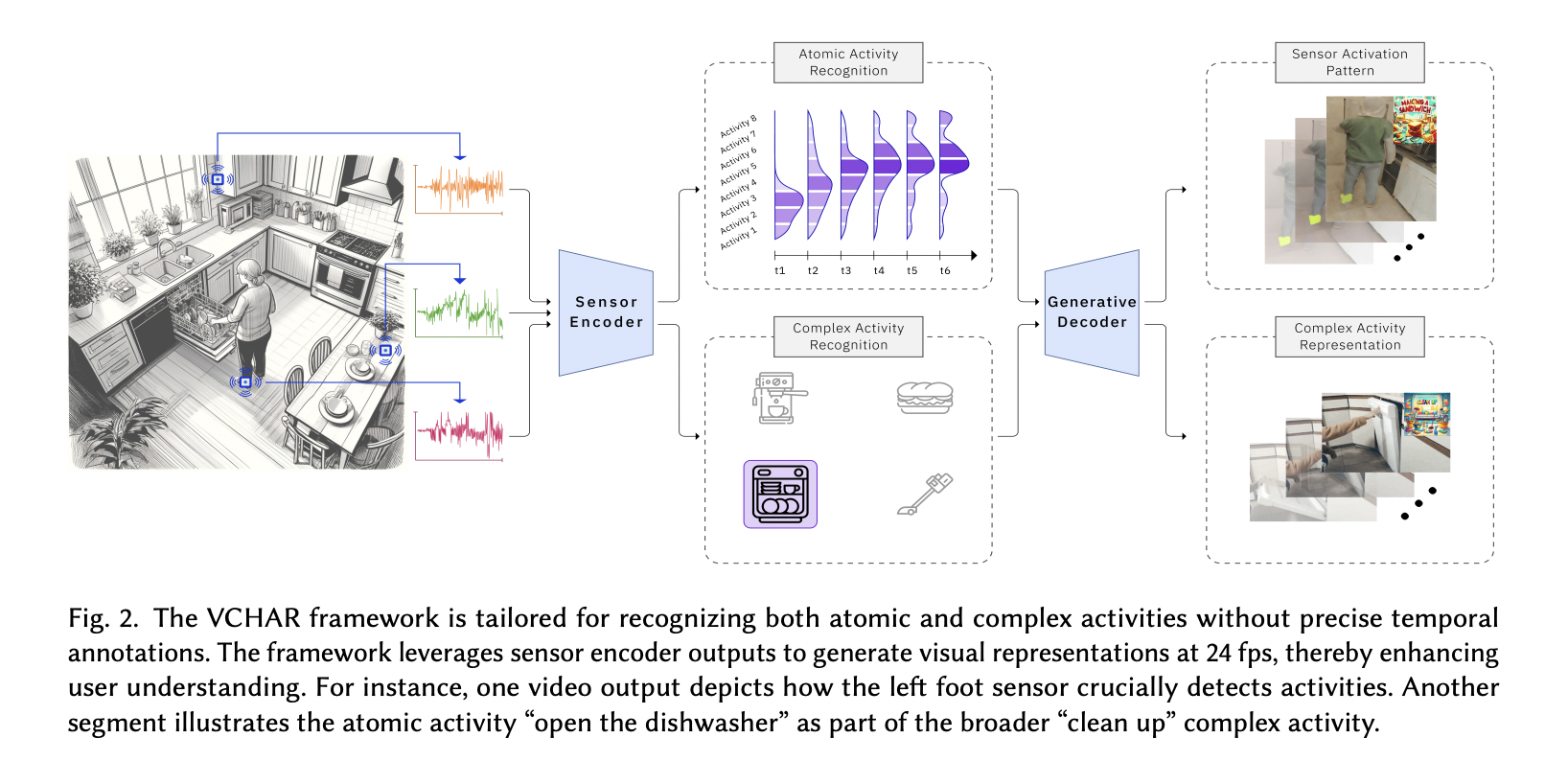
Practical AI Solution for Complex Human Activity Recognition
Challenges in Recognizing Human Activities
Recognizing human activities in smart environments presents challenges due to the labor-intensive and error-prone process of labeling datasets. This makes it impractical in real-world scenarios where accurate and detailed labeling is scarce.
Traditional Methods and Their Limitations
Traditional methods for activity recognition involve labor-intensive labeling and data segmentation, which can lead to inaccuracies and combinatorial complexity, especially when precise temporal information is lacking.
The VCHAR Framework
A team of researchers from Rutgers University introduces the Variance-Driven Complex Human Activity Recognition (VCHAR) framework, which leverages generative methodologies to eliminate the need for precise labeling.
Key Features and Benefits
VCHAR employs a variance-driven approach to improve recognition of complex activities, introduces a generative decoder framework for visual representations, and enhances interpretability and usability of systems.
Value and Practical Applications
The VCHAR framework effectively addresses the challenges of activity recognition, making the insights accessible to non-experts and providing a promising solution for real-world smart environment applications.
Evolve Your Company with AI
Discover how VCHAR can redefine your work processes and identify automation opportunities, define KPIs, select suitable AI solutions, and implement AI gradually to drive business impact. For AI KPI management advice, connect with us at hello@itinai.com.
Enhancing Sales Processes and Customer Engagement
Explore AI solutions at itinai.com to redefine your sales processes and customer engagement, driving continuous improvements with AI insights and solutions.