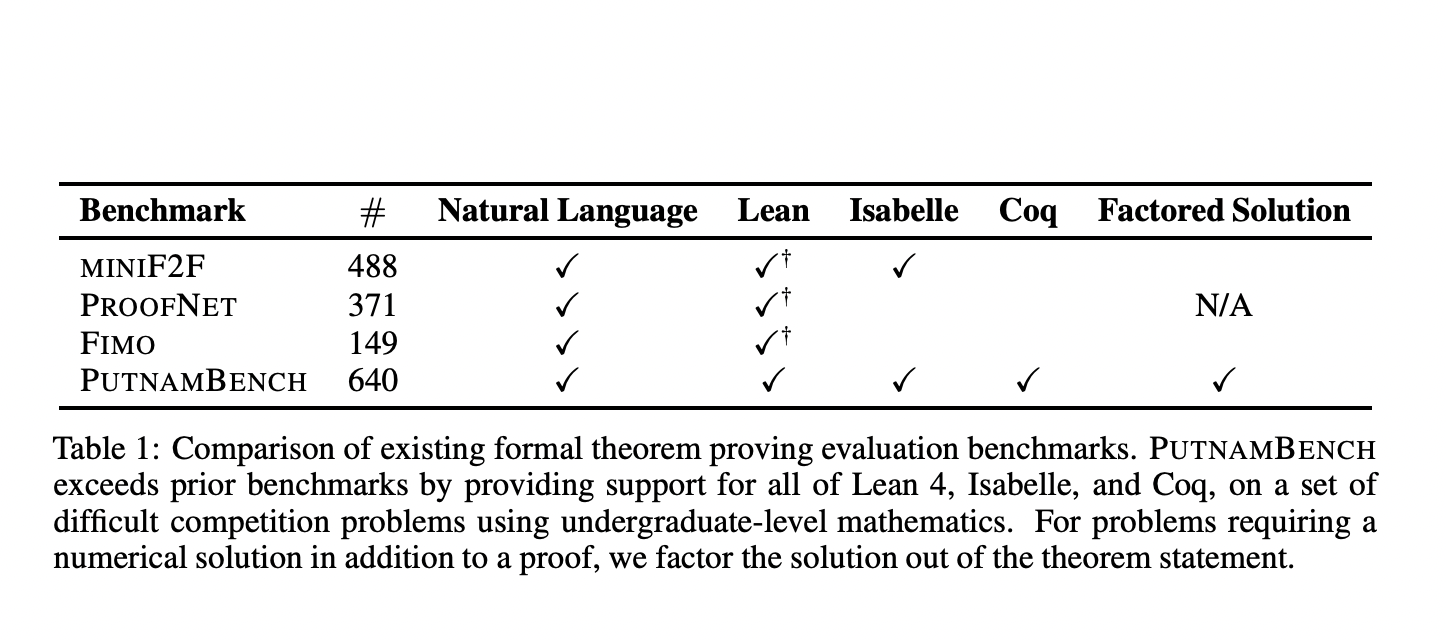
PUTNAMBENCH: A New Benchmark for Neural Theorem-Provers
Automating mathematical reasoning is a key goal in AI, and frameworks like Lean 4, Isabelle, and Coq have played a significant role. Neural theorem-provers aim to automate this process, but there is a lack of comprehensive benchmarks for evaluating their effectiveness.
Addressing the Challenge
PUTNAMBENCH is a new benchmark designed to evaluate neural theorem-provers using problems from the William Lowell Putnam Mathematical Competition, known for its challenging college-level mathematics problems. It includes 1697 formalizations of 640 issues, available in multiple proof languages, ensuring a comprehensive evaluation across different theorem-proving environments.
Evaluating Theorem-Provers
The evaluation of PUTNAMBENCH involved testing several neural and symbolic theorem-provers on the formalizations. The results showed that current methods could solve only a handful of the PUTNAMBENCH problems, highlighting the need for more advanced neural models.
Setting a New Standard
PUTNAMBENCH sets a new standard for rigor and comprehensiveness in evaluating theorem-proving methods. It addresses the limitations of existing benchmarks and will be crucial in driving future research and innovation in the field of AI-driven theorem proving.
AI Solutions for Your Business
Discover how AI can redefine your way of work and sales processes. Identify automation opportunities, define KPIs, select an AI solution, and implement gradually for impactful business outcomes. Connect with us at hello@itinai.com for AI KPI management advice and continuous insights into leveraging AI.