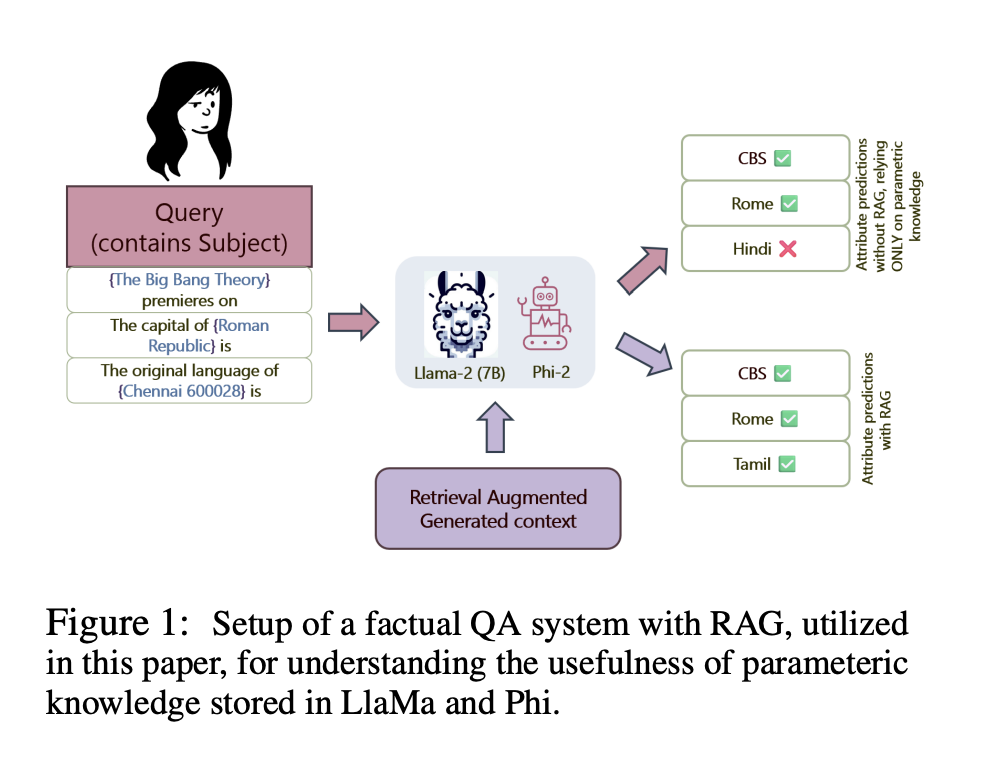
Unveiling the Shortcuts: How Retrieval Augmented Generation (RAG) Influences Language Model Behavior and Memory Utilization
Practical Solutions and Value
Researchers from Microsoft, the University of Massachusetts, Amherst, and the University of Maryland, College Park, conducted a study to understand the impact of Retrieval Augmented Generation (RAG) on language models’ reasoning and factual accuracy.
The study focused on whether language models rely more on external context provided by RAG than their internal memory when generating responses to factual queries.
The researchers proposed a mechanistic examination of RAG pipelines to determine how much language models depend on external context versus their internal memory when answering factual queries.
They utilized advanced language models, LLaMa-2 and Phi-2, and employed techniques like Causal Mediation Analysis, Attention Contributions, and Attention Knockouts to analyze the models’ behavior.
The results revealed that in the presence of RAG context, both LLaMa-2 and Phi-2 models showed a significant decrease in reliance on their internal parametric memory for factual predictions.
The study highlights the need for understanding the interplay between parametric and non-parametric knowledge in retrieval-augmented generation to improve model performance and reliability in practical applications.
AI Solutions for Your Company
– Identify Automation Opportunities: Locate key customer interaction points that can benefit from AI.
– Define KPIs: Ensure your AI endeavors have measurable impacts on business outcomes.
– Select an AI Solution: Choose tools that align with your needs and provide customization.
– Implement Gradually: Start with a pilot, gather data, and expand AI usage judiciously.
For AI KPI management advice, connect with us at hello@itinai.com. And for continuous insights into leveraging AI, stay tuned on our Telegram or Twitter.
Discover how AI can redefine your sales processes and customer engagement. Explore solutions at itinai.com.