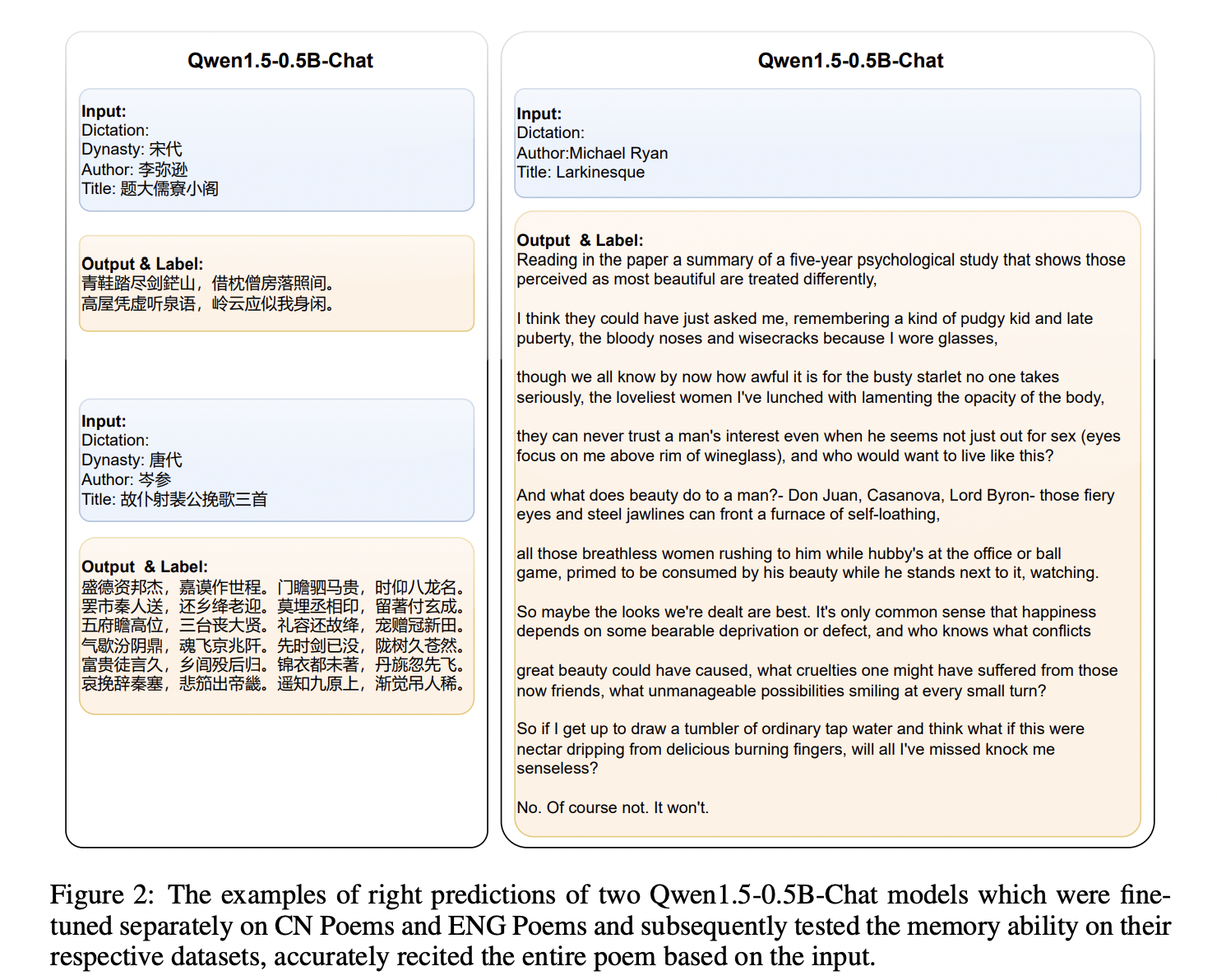
Practical Solutions and Value of Unveiling Schrödinger’s Memory in Language Models
Understanding LLM Memory Mechanisms
LLMs derive memory from input, not external storage, enhancing retention by extending context length and using external memory systems.
Exploring Schrödinger’s Memory
Hong Kong Polytechnic University researchers introduce “Schrödinger’s memory” in LLMs, dynamically approximating past information based on input cues.
Deep Learning Basis in Transformers
The Universal Approximation Theorem (UAT) forms the basis of memory in Transformer-based LLMs, allowing dynamic adjustment for memory-like capabilities.
Memory Capabilities of LLMs
LLMs exhibit memory by fitting input to output, similar to human recall, with larger models showing better performance and a correlation between input length and memory accuracy.
Comparing Human and LLM Cognition
LLMs and human cognition share dynamic reasoning abilities, adjusting to inputs for creativity and adaptability, with limitations attributed to model size, data quality, and architecture.
Conclusion: LLMs and Human Memory
LLMs demonstrate memory capabilities akin to human cognition, with “Schrödinger’s memory” revealed by specific inputs, validating LLM memory through experiments and exploring cognitive process similarities and differences.
For more details, check out the full research paper.
Evolve Your Company with AI
How AI Can Redefine Your Work
Identify automation opportunities, define KPIs, select suitable AI solutions, and implement gradually for business impact.
AI KPI Management Advice
Connect with us at hello@itinai.com for AI KPI management advice and stay updated on leveraging AI through our Telegram and Twitter channels.
Redefining Sales Processes with AI
Discover how AI can enhance sales processes and customer engagement at itinai.com.