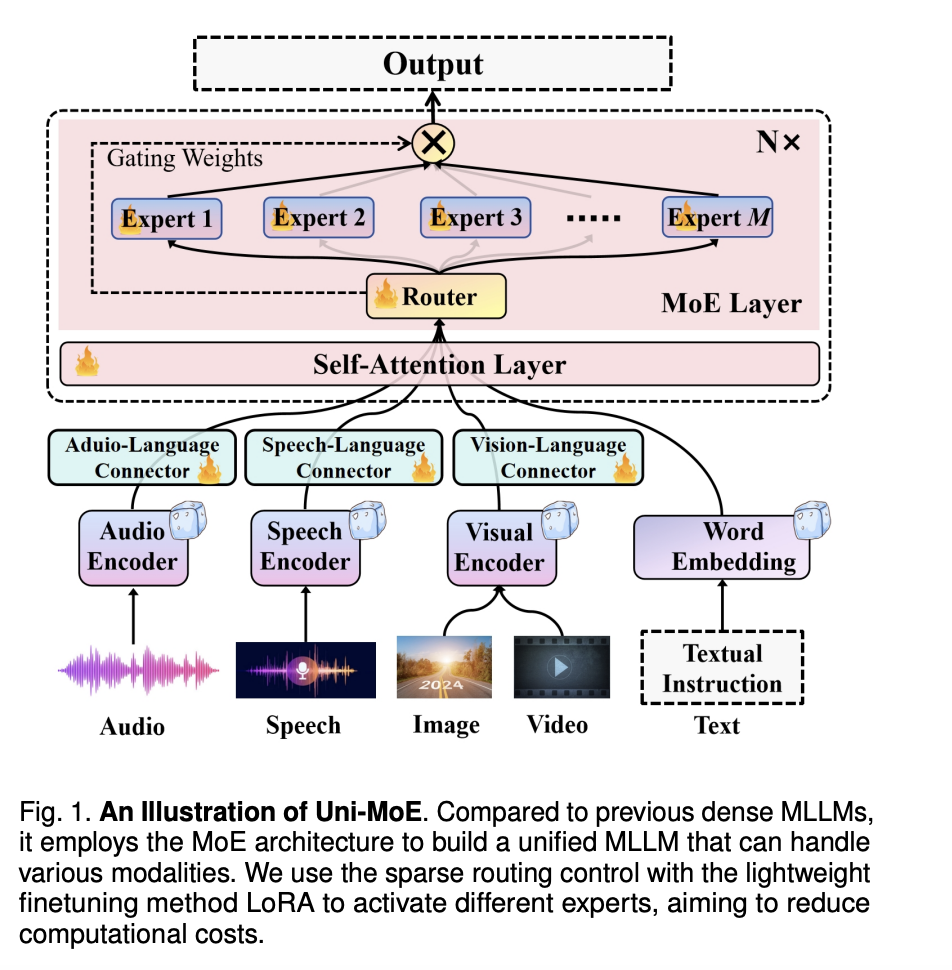
Unlocking the Potential of Multimodal Language Models with Uni-MoE
Large multimodal language models (MLLMs) are crucial for natural language understanding, content recommendation, and multimodal information retrieval. Uni-MoE, a Unified Multimodal LLM, represents a significant advancement in this field.
Addressing Multimodal Challenges
Traditional methods for handling diverse modalities often face issues with computational overhead and lack of flexibility. Uni-MoE overcomes these challenges with its innovative approach.
Technical Advancements
Uni-MoE’s technical advancements include a Mixture of Experts (MoE) framework specializing in different modalities and a three-phase training strategy for optimized collaboration. The advanced routing mechanisms and balancing loss techniques make it a robust solution for complex multimodal tasks.
Superior Performance
Uni-MoE demonstrates superior performance with accuracy scores ranging from 62.76% to 66.46% across evaluation benchmarks. It outperforms dense models, exhibits better generalization, and handles long speech understanding tasks effectively.
Future AI Systems
Uni-MoE’s success marks a significant leap forward in multimodal learning, promising enhanced performance, efficiency, and generalization for future AI systems.
Unlock the Power of AI with Uni-MoE
Stay competitive and redefine your way of work with Uni-MoE. Discover how AI can redefine your sales processes and customer engagement with practical AI solutions like the AI Sales Bot from itinai.com/aisalesbot.
Practical AI Implementation
Identify Automation Opportunities, Define KPIs, Select an AI Solution, and Implement Gradually to evolve your company with AI. Connect with us at hello@itinai.com for AI KPI management advice.