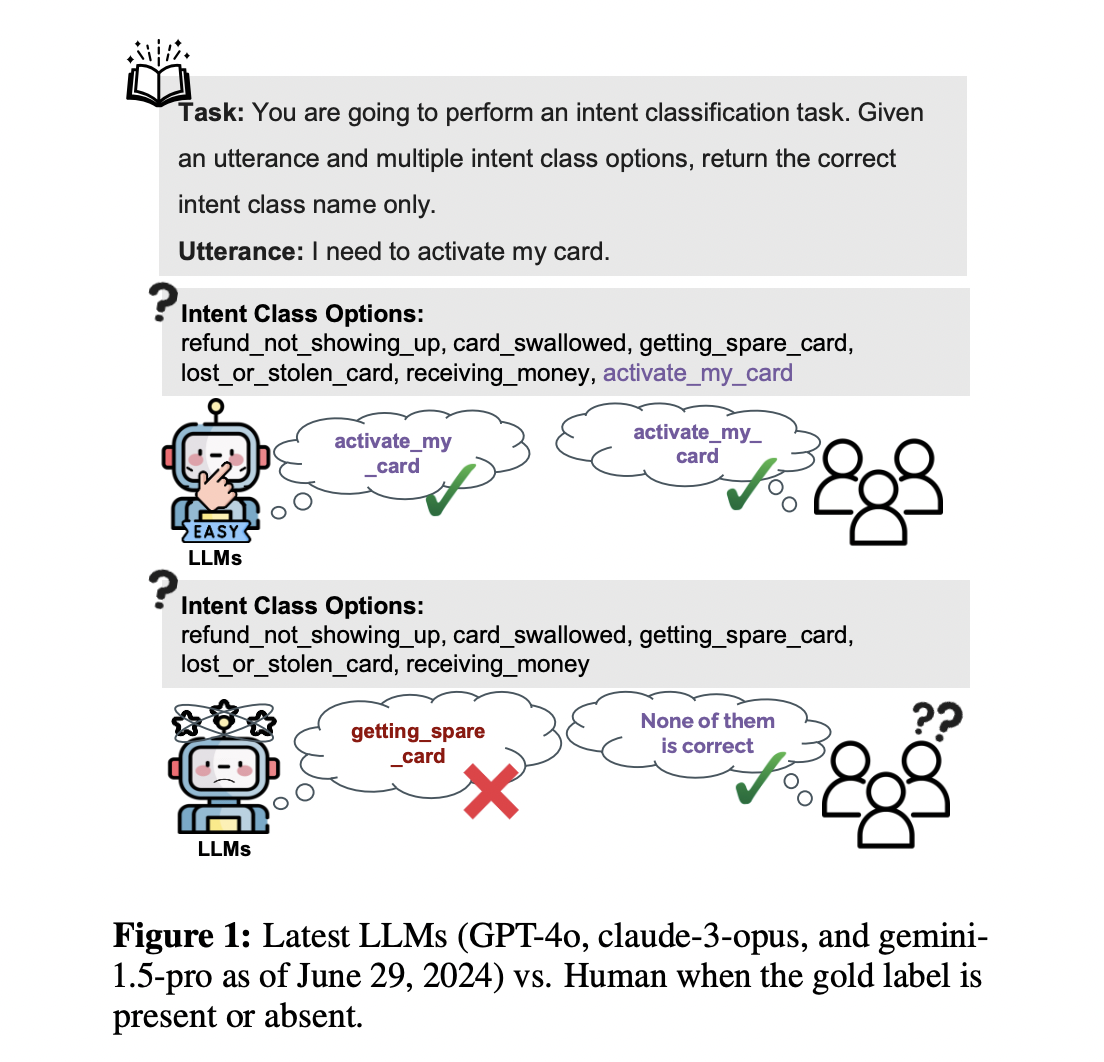
Understanding the Limitations of Large Language Models (LLMs): New Benchmarks and Metrics for Classification Tasks
Practical Solutions and Value
Large Language Models (LLMs) have demonstrated exceptional performance in classification tasks, but they face challenges in comprehending and accurately processing labels. To address these limitations, new benchmarks and metrics have been introduced to assess LLMs’ performance more comprehensively.
The introduction of the KNOW-NO Benchmark, which includes tasks such as BANK77, MC-TEST, and EQUINFER, aims to evaluate LLMs in scenarios where correct labels are absent. This provides a more realistic assessment of their capabilities.
The OMNIACCURACY metric combines results when accurate labels are present and when they are not, offering a more in-depth evaluation of LLMs’ performance. This helps to better approximate human-level discrimination intelligence in classification tasks.
By understanding these limitations and utilizing the new benchmarks and metrics, companies can leverage AI more effectively in their operations. They can identify automation opportunities, define KPIs, select suitable AI solutions, and implement AI gradually to drive business outcomes.
For AI KPI management advice and insights into leveraging AI, connect with us at hello@itinai.com or stay tuned on our Telegram t.me/itinainews or Twitter @itinaicom.