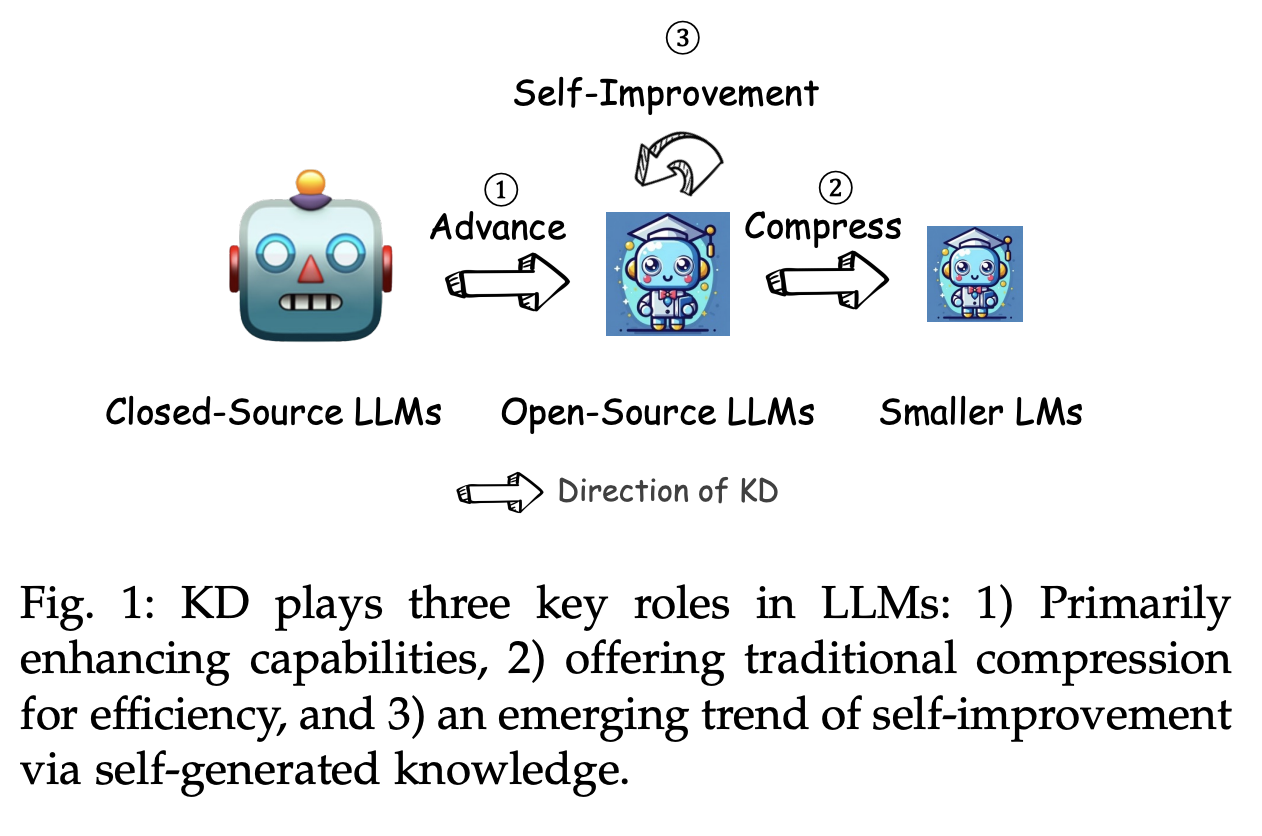
Practical Solutions and Value of Knowledge Distillation in AI
Key Technique in AI
Knowledge Distillation (KD) is crucial for transferring the capabilities of proprietary models to open-source alternatives, improving their performance, compressing them, and increasing their efficiency without sacrificing functionality.
Research Insights
A recent study highlights the significance of KD in transferring advanced knowledge to smaller, less resource-intensive models, enabling real-world implementations in various domains.
Distillation Process
Distillation condenses a complex teacher model into a more manageable student model, transferring the teacher’s knowledge to enable comparable performance with less processing power.
Advantages of Distillation
Distillation substantially decreases model size and computational needs, making it suitable for resource-constrained environments such as embedded systems and mobile devices.
Freedom in Model Architecture
Distillation allows for the creation of smaller models using knowledge from larger models, resulting in significant performance gains without the need for extra training data.
Arcee AI’s DistillKit
Arcee AI’s DistillKit offers distillation techniques that can result in significant performance gains, making AI systems more powerful, accessible, and efficient.
AI Implementation Tips
Implement AI gradually, starting with a pilot, gathering data, and expanding usage judiciously to ensure measurable impacts on business outcomes.
Connect with Us
For AI KPI management advice, connect with us at hello@itinai.com. For continuous insights into leveraging AI, stay tuned on our Telegram t.me/itinainews or Twitter @itinaicom.