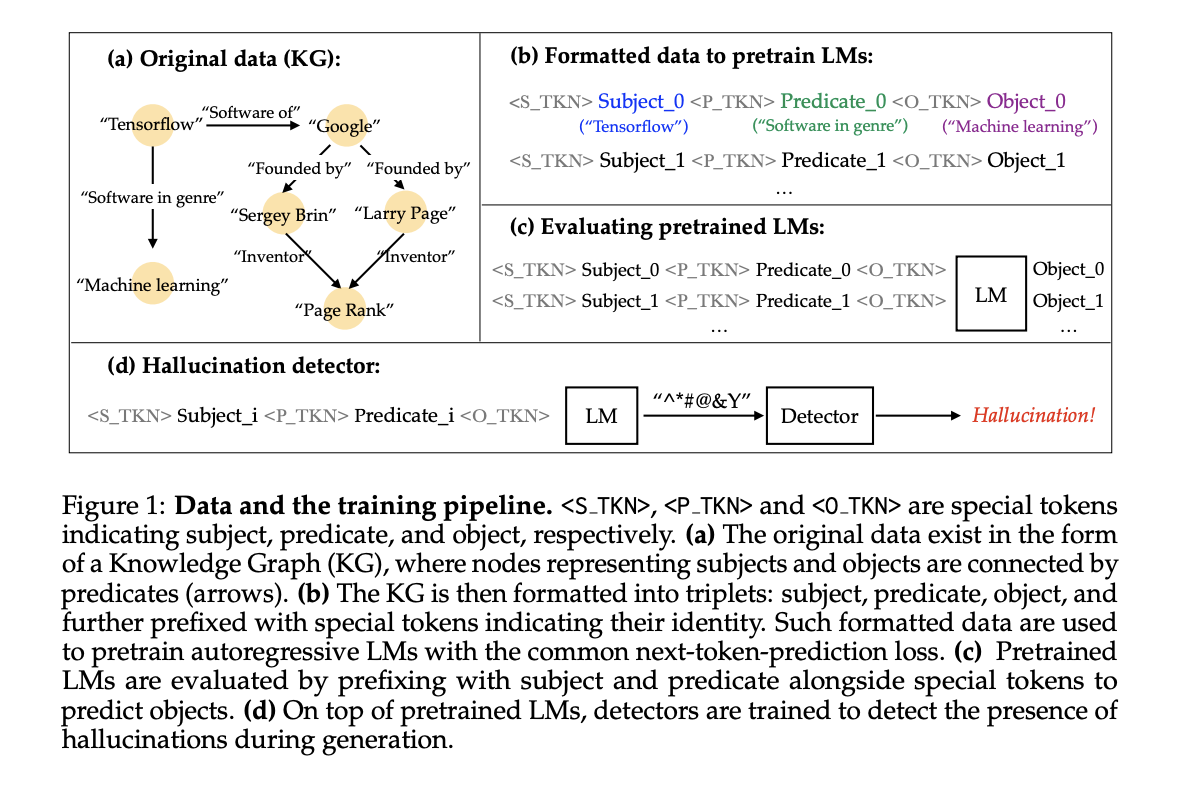
Understanding Hallucination Rates in Language Models: Insights from Training on Knowledge Graphs and Their Detectability Challenges
Practical Solutions and Value Highlights
Language models (LMs) perform better with larger size and training data, but face challenges with hallucinations. A study from Google Deepmind focuses on reducing hallucinations in LMs by using knowledge graphs (KGs) for structured training data.
The research investigates the relationship between LM scale and hallucinations, finding that larger, longer-trained LMs hallucinate less. However, achieving low hallucination rates requires more resources than previously thought.
Traditional LMs often produce hallucinations due to language ambiguity. The study uses a knowledge graph approach to provide a clearer understanding of how LMs misrepresent training data, allowing for precise evaluation of hallucinations and their relationship to model scale.
The study constructs a dataset using knowledge graph triplets, enabling precise control over training data and quantifiable hallucination measurement. LMs are trained on this dataset, optimizing auto-regressive log-likelihood.
Findings reveal that larger LMs and extended training reduce hallucinations on fixed datasets, while increased dataset size elevates hallucination rates. A trade-off exists between fact recall and generalization ability, emphasizing the importance of balancing model size and training duration to mitigate hallucinations.
The study highlights the complex relationship between model scale, dataset size, and hallucination rates, providing insights into LM hallucinations and their detectability.
If you want to evolve your company with AI, stay competitive, and use Understanding Hallucination Rates in Language Models for your advantage. Discover how AI can redefine your work and sales processes, and connect with us for AI KPI management advice and continuous insights into leveraging AI.