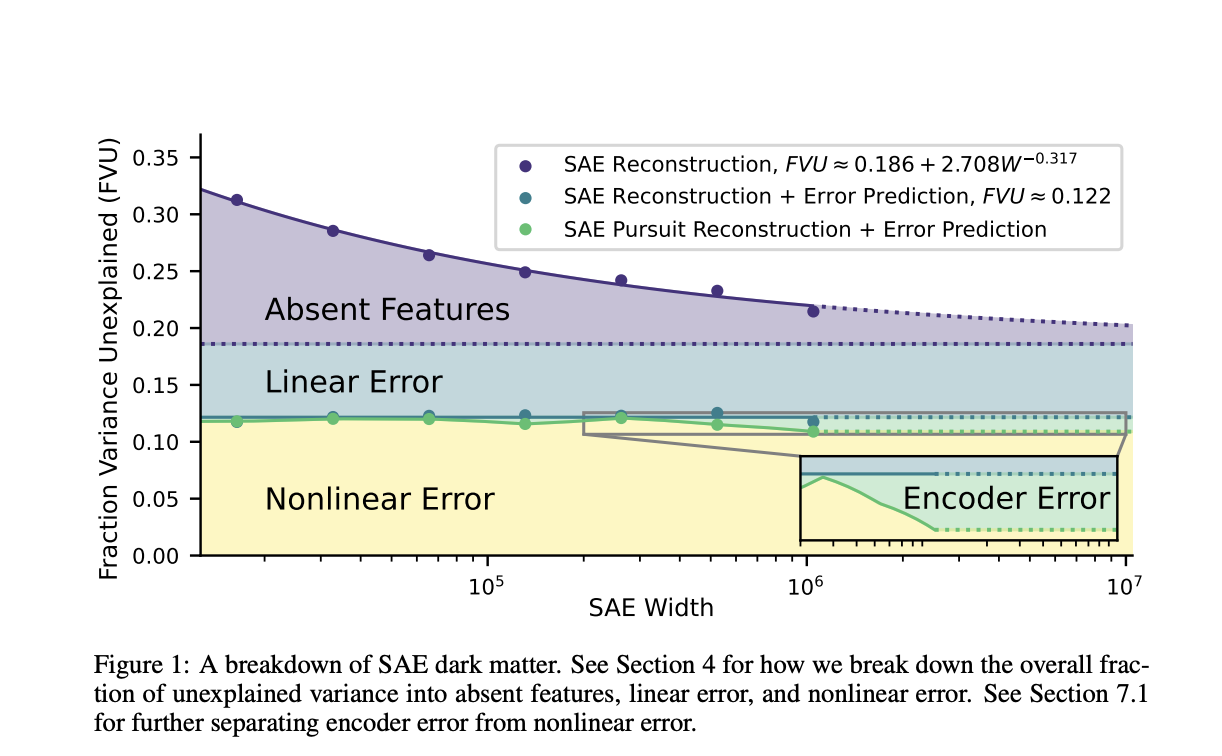
Sparse Autoencoders: Understanding Their Role and Limitations
What Are Sparse Autoencoders (SAEs)?
Sparse Autoencoders (SAEs) help break down language model activations into simpler, understandable features. However, they don’t fully explain all model behaviors, leaving some unexplained data, referred to as “dark matter.”
Goals of Mechanistic Interpretability
The goal is to decode neural networks by mapping their internal features. SAEs learn to represent data sparsely, but their accuracy can falter when faced with complex activation patterns.
Key Findings from Recent Research
- The Linear Representation Hypothesis (LRH) suggests that language model features can be simplified into linear directions. However, newer studies reveal that some models show non-linear behavior.
- Research indicates that SAE errors are often more significant than random changes and that larger SAEs can capture more complex features.
- Over 90% of SAE error can be predicted from initial activation data, but larger SAEs struggle with context reconstruction.
Reducing Nonlinear Errors
The study explored two methods to reduce errors:
- Inference Time Optimization: This method improved overall error reduction by 3-5%.
- Using Earlier Layer Outputs: This method proved more effective in reducing errors.
Predicting SAE Errors
The research focused on how well SAE errors can be predicted. Key insights include:
- Error norms are highly predictable, explaining 86%-95% of variance.
- Nonlinear error prediction remains constant even as SAE size increases.
Challenges and Future Directions
The study found that simply increasing SAE size does not effectively minimize nonlinear errors. Alternative strategies, such as exploring new learning methods, may be needed.
Stay Connected
For updates on this research, follow us on Twitter, join our Telegram Channel, and connect with our LinkedIn Group. If you enjoy our content, subscribe to our newsletter and join our 55k+ ML SubReddit community.
Upcoming Webinar
Join us on October 29, 2024, to learn about the best platform for serving fine-tuned models with the Predibase Inference Engine.
Leverage AI for Your Business
Enhance your business competitiveness with AI:
- Identify Automation Opportunities: Find key customer interaction points that can benefit from AI.
- Define KPIs: Ensure that your AI projects have measurable impacts on your business.
- Select an AI Solution: Choose tools that fit your needs and allow for customization.
- Implement Gradually: Start with a pilot project, gather data, and expand wisely.
For AI KPI management advice, contact us at hello@itinai.com. For ongoing insights, follow us on Telegram or Twitter.
Transform Your Sales and Customer Engagement with AI
Explore innovative AI solutions at itinai.com.