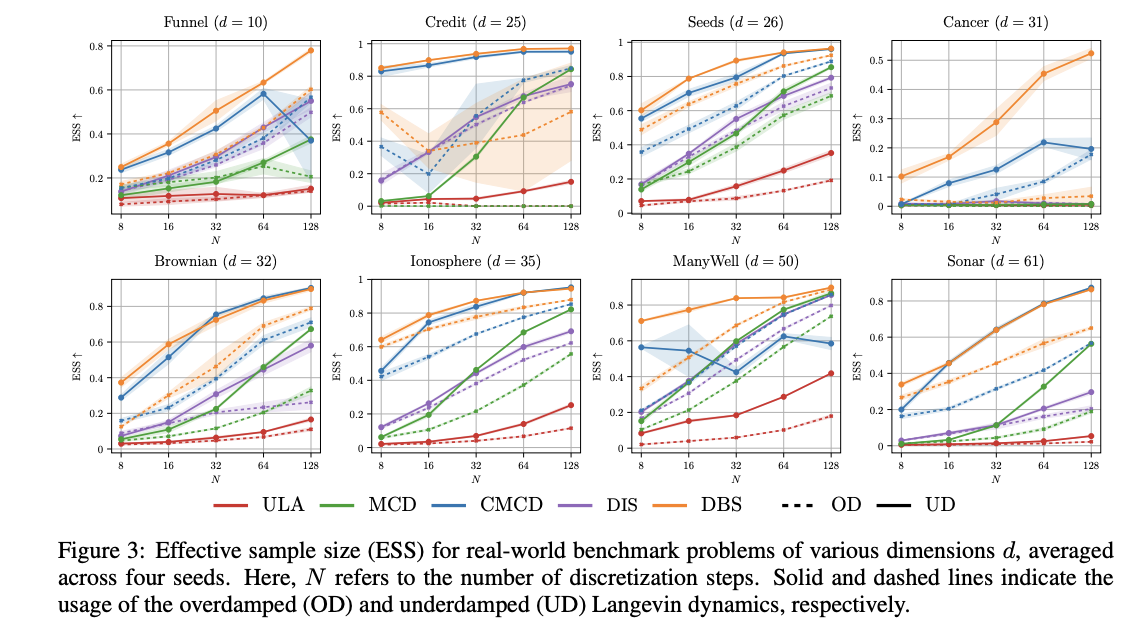
Innovative Sampling Techniques in Artificial Intelligence
Recent research from a collaboration between the Karlsruhe Institute of Technology, NVIDIA, and the Zuse Institute Berlin has unveiled a groundbreaking framework for efficiently sampling from complex distributions. This new method, known as underdamped diffusion sampling, addresses significant challenges faced by traditional sampling methods, especially in multimodal distributions.
Understanding the Challenges
Traditional sampling methods, particularly those based on overdamped Langevin dynamics, often struggle with slow convergence rates when navigating between different modes of a distribution. This inefficiency can hinder timely decision-making in various business applications.
Current Approaches and Limitations
- Annealed Importance Sampling (AIS): Bridges prior and target distributions but can be inefficient.
- Unadjusted Langevin Annealing (ULA): Uses uncorrected overdamped dynamics, which may lead to suboptimal results.
- Monte Carlo Diffusion (MCD): Optimizes targets but may not always reduce variance effectively.
- Controlled Monte Carlo Diffusion (CMCD): Focuses on kernel optimization with resampling strategies.
- Diffusion Bridge Sampler (DBS): Learns both forward and backward kernels but has limitations in real-world applications.
A New Framework for Underdamped Diffusion Sampling
The researchers’ proposed framework offers a comprehensive solution that incorporates existing diffusion models while introducing underdamped versions with degenerate diffusion matrices. This innovative approach allows for smoother sampling paths and tackles the challenge of sampling from unnormalized densities.
Performance Evaluation
The framework was rigorously tested against five key sampling methods: ULA, MCD, CMCD, DIS, and DBS. The results were promising:
- Underdamped Langevin dynamics consistently outperformed overdamped alternatives.
- The underdamped DBS achieved superior results even with minimal discretization steps, leading to significant computational savings.
- Specialized integrators demonstrated marked improvements over classical methods, enhancing overall performance without added computational burden.
Practical Business Solutions
Businesses can leverage these innovative sampling techniques to enhance decision-making processes and operational efficiency. Here are some actionable strategies:
- Automate Processes: Identify areas where AI can streamline operations, particularly in data analysis and customer interactions.
- Monitor Key Performance Indicators (KPIs): Establish metrics to evaluate the effectiveness of AI implementations and ensure positive business impacts.
- Select Appropriate Tools: Choose AI tools that can be customized to fit your specific business needs and objectives.
- Start Small: Initiate AI projects on a smaller scale, gather data on their effectiveness, and then expand their usage progressively.
Conclusion
The introduction of underdamped diffusion sampling marks a significant advancement in the field of artificial intelligence. By providing a robust framework for efficient sampling from complex distributions, businesses can harness the power of AI to improve decision-making and operational efficiency. This research not only sets a new standard for sampling techniques but also paves the way for future innovations in generative modeling and beyond.
For further insights on integrating AI into your business, feel free to reach out to us at hello@itinai.ru or follow us on our social media platforms.