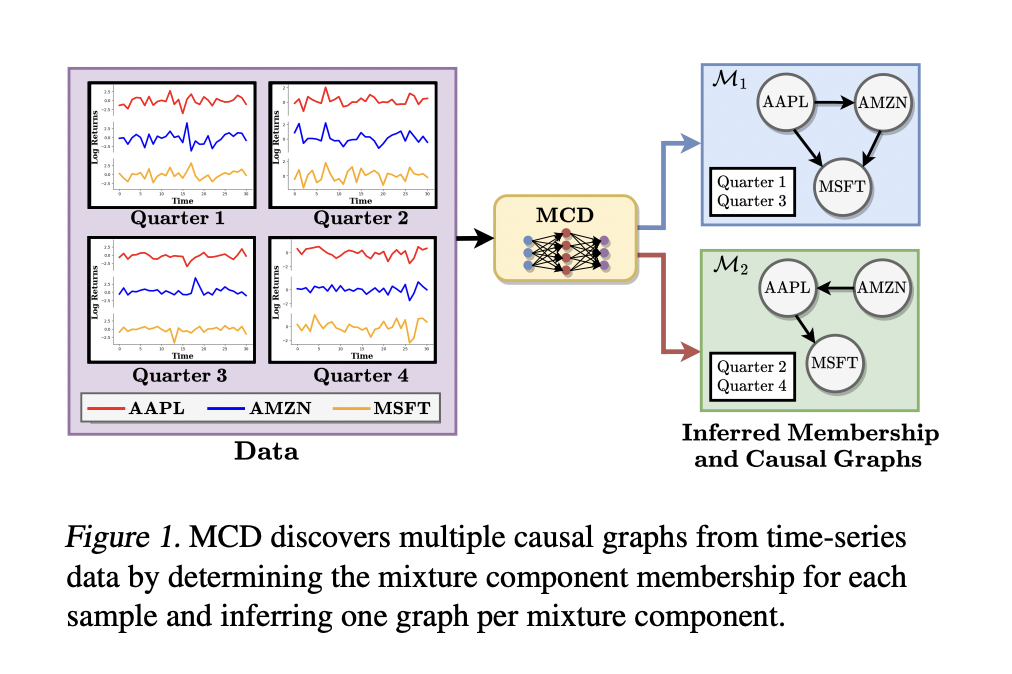
Practical Solutions for Causal Discovery in Heterogeneous Time-Series Data
Challenges in Causal Discovery
Traditional methods for causal discovery in time-series data face limitations when dealing with diverse causal mechanisms. Real-world scenarios, such as gene regulatory networks and stock market interactions, involve complex and heterogeneous data, hindering accurate representation of causal relationships in machine learning applications.
The MCD Approach
Researchers from UCSD propose a robust approach called Mixture Causal Discovery (MCD) to address the challenge of causal discovery in heterogeneous time-series data. MCD assumes that data is generated from a mixture of unknown structural causal models (SCMs) and employs variational inference to approximate the intractable posterior distribution of SCMs.
Value and Practical Applications
MCD offers two variants: MCD-Linear for linear relationships with independent noise, and MCD-Nonlinear for nonlinear relationships with history-dependent noise. This flexible framework demonstrates effectiveness in discovering multiple causal structures in heterogeneous time-series data across various synthetic and real-world scenarios, with applications in climate science, finance, and healthcare.
Performance and Results
MCD performed well on synthetic datasets, outperforming baselines on both linear and nonlinear data. It demonstrated strong clustering accuracy in identifying underlying causal models across various datasets, showcasing its effectiveness in discovering multiple causal structures in heterogeneous time-series data.
Research and Collaboration
For more information on the research and to access the GitHub repository, visit the paper and GitHub links provided. The researchers at UCSD have made significant contributions to this project, addressing the crucial challenge of causal discovery in complex, multimodal data scenarios.
AI Solutions for Business
For businesses looking to evolve with AI, it is essential to identify automation opportunities, define measurable KPIs, select suitable AI solutions, and implement AI usage gradually. For AI KPI management advice and continuous insights into leveraging AI, connect with us at hello@itinai.com and stay tuned on our Telegram and Twitter channels.
AI for Sales Processes and Customer Engagement
Discover how AI can redefine sales processes and customer engagement by exploring solutions at itinai.com.