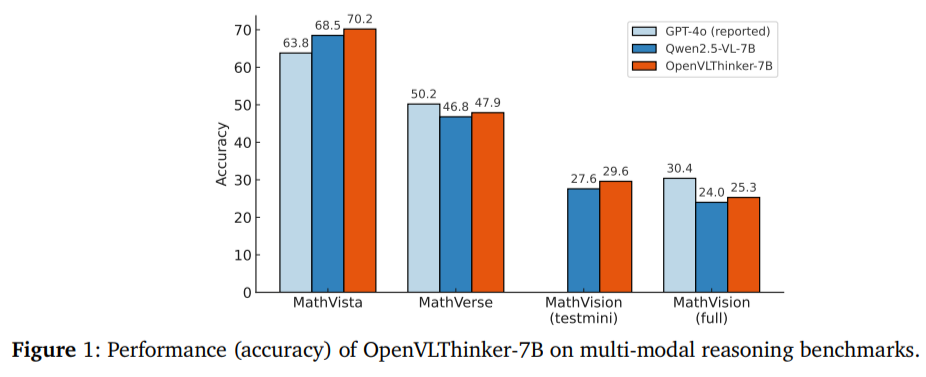
Enhancing Visual Reasoning with OpenVLThinker-7B
The University of California, Los Angeles (UCLA) has developed a groundbreaking model known as OpenVLThinker-7B. This model utilizes reinforcement learning to improve complex visual reasoning and step-by-step problem solving in multimodal systems. Here, we will discuss its significance, methodology, and practical applications in business.
Understanding the Challenge
Large vision-language models (LVLMs) have made significant strides in combining language processing with image interpretation. However, they often struggle with tasks requiring multi-step reasoning, such as understanding charts or solving visual math problems. This limitation stems from their inability to perform complex reasoning involving logical deduction based on visual data.
Innovative Methodology
The researchers at UCLA addressed these challenges by introducing a novel training methodology that combines supervised fine-tuning (SFT) and reinforcement learning (RL). This approach consists of several key steps:
- Initial Caption Generation: The model begins by generating image captions using a base model, Qwen2.5-VL-3B.
- Structured Reasoning Chains: These captions are then processed to create structured reasoning outputs, which serve as training data.
- Iterative Training: The model undergoes multiple training cycles, alternating between SFT and RL to enhance its reasoning capabilities.
Performance Improvements
Quantitative results demonstrate the effectiveness of OpenVLThinker-7B. For instance, on the MathVista benchmark, the model achieved an accuracy of 70.2%, a significant improvement from the base model’s 50.2%. Similar enhancements were observed across other datasets, such as MathVerse and MathVision, highlighting the model’s ability to learn and generalize better to complex tasks.
Practical Applications in Business
OpenVLThinker-7B presents several opportunities for businesses, particularly in the areas of education, visual analytics, and assistive technology. Here are some practical solutions:
- Automated Educational Tools: Develop AI-driven platforms that enhance learning through visual problem-solving capabilities.
- Visual Data Analytics: Utilize the model for interpreting complex data visualizations, providing clearer insights for decision-making.
- Assistive Technologies: Create tools that aid individuals with disabilities by interpreting visual cues and generating helpful responses.
Conclusion
In summary, OpenVLThinker-7B represents a significant advancement in the field of artificial intelligence, particularly in enhancing visual reasoning capabilities. By leveraging a novel training approach that combines supervised fine-tuning with reinforcement learning, this model not only improves accuracy but also addresses the critical need for multi-step reasoning in multimodal tasks. Businesses can harness this technology to automate processes, enhance customer interactions, and ultimately drive growth.