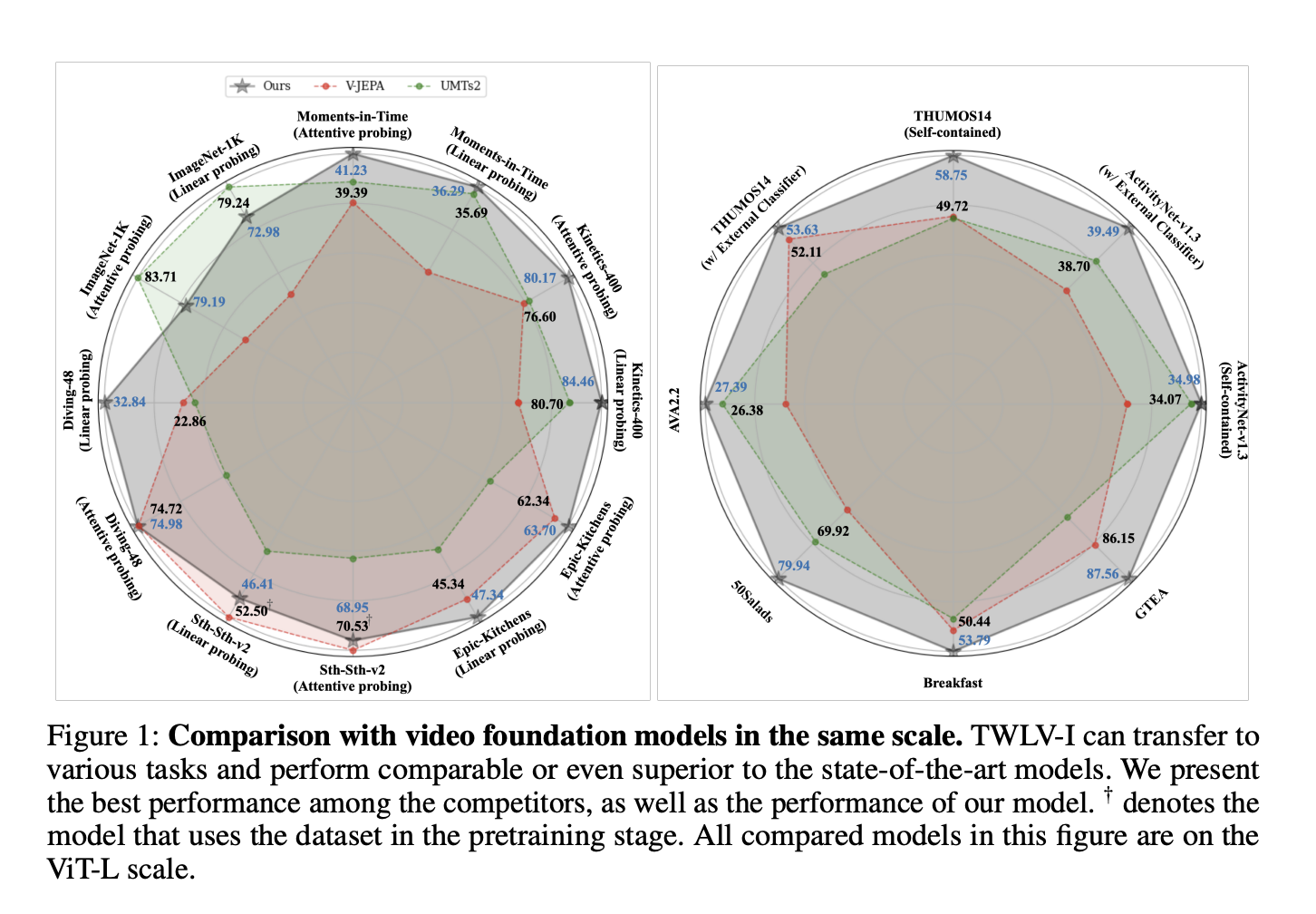
Practical Solutions for Video Analysis
Challenges in Video Analysis
Language Foundation Models (LFMs) and Large Language Models (LLMs) have inspired the development of Image Foundation Models (IFMs) in computer vision. However, applying these techniques to video analysis presents challenges in capturing detailed motion and small changes between frames.
Overcoming Challenges with TWLV-I
A team from Twelve Labs has proposed TWLV-I, a new model designed to provide embedding vectors for videos that capture appearance and motion. TWLV-I shows strong performance on appearance and motion-focused action recognition benchmarks and achieves state-of-the-art performance in video-centric tasks.
Model Architecture and Training
TWLV-I adopts the Vision Transformer architecture and utilizes two frame sampling methods to overcome computational constraints. The model tokenizes input videos into patches, processes them through the transformer, and pools the resulting patch-wise embeddings to obtain the overall video embedding.
Performance and Future Impact
TWLV-I outperforms existing models in action recognition tasks and is expected to be widely used in various applications. The evaluation and analysis methods introduced with TWLV-I are anticipated to guide further research in the video understanding field.
AI Integration and KPI Management
For companies looking to evolve with AI, TWLV-I offers robust visual representations for both motion and appearance-based videos. Identifying automation opportunities, defining KPIs, selecting suitable AI solutions, and implementing AI gradually are key steps in leveraging AI for business growth.
Connect with Us
For AI KPI management advice and continuous insights into leveraging AI, connect with us at hello@itinai.com. Stay tuned on our Telegram t.me/itinainews or Twitter @itinaicom for the latest updates.
Discover AI Solutions
Explore how AI can redefine your sales processes and customer engagement at itinai.com.