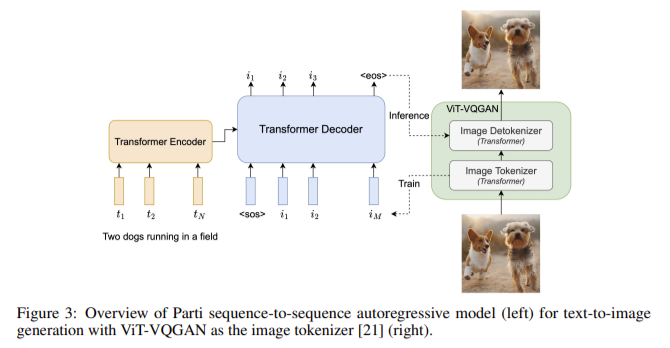
Transforming AI with Transfusion Architecture
Introduction to GPT-4o and Transfusion Architecture
OpenAI’s GPT-4o represents a significant advancement in multimodal artificial intelligence, combining fluent text and high-quality image generation in a single output. Unlike earlier models, which required external tools for image creation, GPT-4o utilizes a novel Transfusion architecture. This architecture integrates Transformer models for language processing with Diffusion models for image synthesis, enabling seamless text and image generation.
Understanding the Transfusion Architecture
How Transfusion Works
The Transfusion architecture employs a single Transformer model that can output both text and images. It incorporates special tokens that denote the beginning and end of image content, allowing the model to generate images and text in a cohesive manner. This internal integration leads to better contextual understanding and more relevant image generation.
Comparative Analysis of Previous Approaches
- Tool-Based Methods: Prior to GPT-4o, models like ChatGPT relied on external image generators, which limited the integration of language and image generation.
- Token-Based Fusion: Earlier efforts, such as DALL-E and Chameleon, treated images as sequences of discrete tokens, which often resulted in loss of detail and slower generation speeds.
Key Features of Transfusion Architecture
Unified Sequence Generation
Transfusion allows for the concatenation of text and image data into a single sequence, enhancing the model’s ability to produce coherent outputs. The use of Begin-of-Image (BOI) and End-of-Image (EOI) markers facilitates clear boundaries between text and image content.
Continuous Image Representation
Rather than using fixed tokens, Transfusion represents images as continuous vectors, which significantly improves the quality of generated images. This method eliminates the bottleneck associated with discretization, allowing for richer and more detailed output.
Efficient Training and Scalability
With the ability to compress images into fewer latent patches, Transfusion is more efficient than previous models. For example, a 7 billion parameter Transfusion model can represent an image with only 16-20 patches, compared to hundreds required by older models, leading to faster generation times and reduced computational costs.
Case Studies and Performance Metrics
Benchmarking Against Previous Models
In benchmark tests, a 7.3 billion parameter Transfusion model achieved a Fréchet Inception Distance (FID) score of 6.78 on the MS-COCO dataset, significantly outperforming a similar-sized Chameleon model, which scored 26.7. This demonstrates the superior image quality and fidelity achievable with the Transfusion architecture.
Limitations and Future Directions
While the Transfusion model is a leap forward, it still faces challenges, such as slower image output due to the iterative nature of diffusion processes. However, ongoing research aims to refine this architecture further, making it even more efficient and capable.
Practical Business Solutions
Adopting AI in Your Business
- Identify Automation Opportunities: Look for processes where AI can streamline operations.
- Measure Impact: Establish key performance indicators (KPIs) to evaluate the effectiveness of AI implementations.
- Select Suitable Tools: Choose AI tools that align with your business objectives and allow customization.
- Start Small: Implement AI in small projects, gather data, and scale gradually based on effectiveness.
Conclusion
The Transfusion architecture demonstrates that integrating text and image generation within a single model is not only possible but also highly effective. GPT-4o excels in producing high-quality, coherent outputs that combine text and imagery. As businesses look to harness the power of AI, understanding and implementing such advanced architectures can lead to significant operational improvements and innovative capabilities.