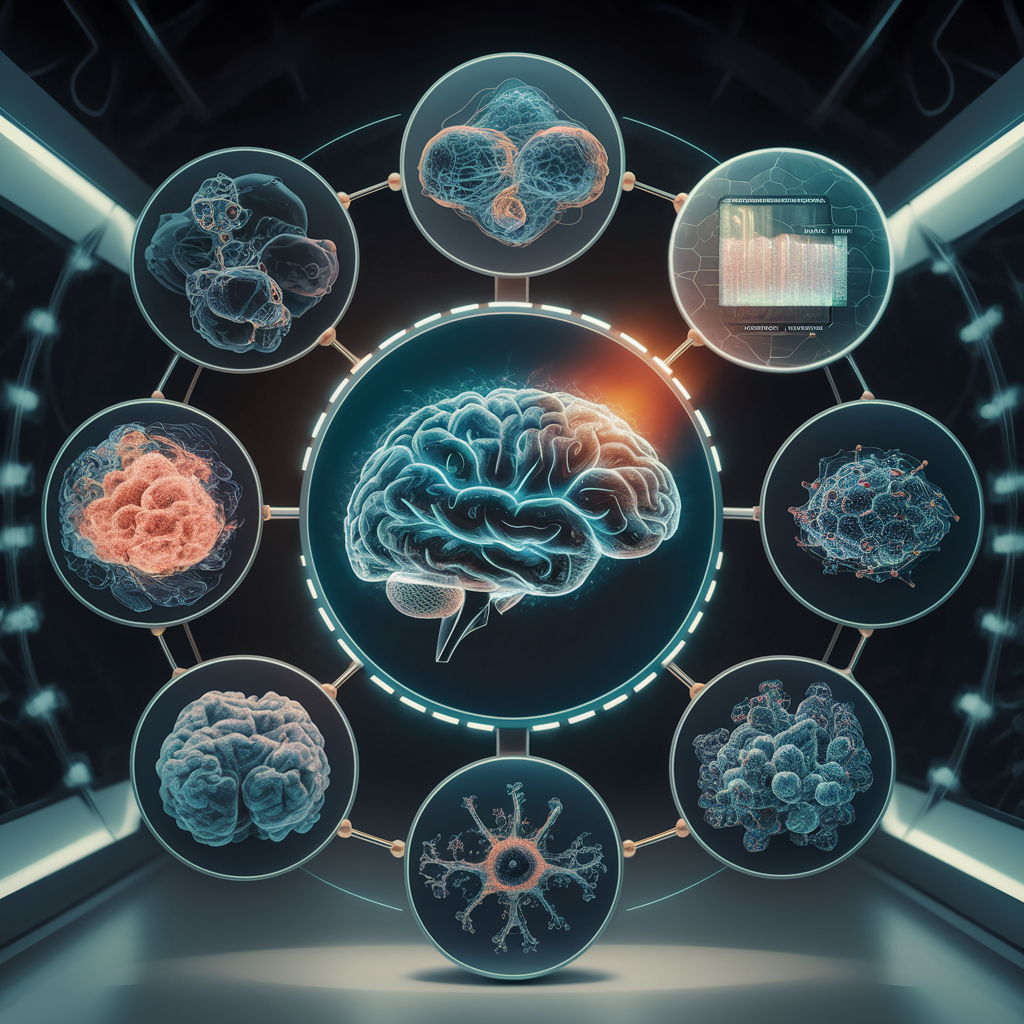
Transformative Applications of Deep Learning in Regulatory Genomics and Biological Imaging
Practical Solutions and Value
Recent technological advancements in genomics and imaging have led to a vast increase in molecular and cellular profiling data. Modern machine learning, particularly deep learning, offers solutions for handling large datasets, uncovering hidden structures, and making accurate predictions.
Machine learning methods automate the critical step of feature extraction, improving the performance of predictive models without requiring predefined assumptions about underlying mechanisms. Deep learning captures complex functions by transforming raw data into abstract feature representations through multiple neural network layers, showing significant advancements in image and computational biology.
Deep Learning Transformations in Regulatory Genomics
Deep neural networks offer advantages in regulatory genomics by learning features directly from sequence data, capturing nonlinear dependencies and interactions across wider genomic contexts. They have been effectively used to predict splicing activity, DNA- and RNA-binding protein specificities, and epigenetic marks, demonstrating their potential in understanding DNA sequence alterations.
Advances in Predicting Mutation Effects and Joint Trait Predictions Using Deep Learning
Deep neural networks applied to raw DNA sequences can predict the effects of mutations in silico, complementing QTL mapping and aiding in identifying rare regulatory SNVs. Advances in CNNs allow predicting multiple traits from larger DNA sequence windows, improving performance and computational efficiency.
Deep Learning in Biological Image Analysis
Deep neural networks, particularly CNNs, have significantly advanced biological image analysis, outperforming traditional methods like Markov random fields. The trend is towards end-to-end analysis pipelines utilizing large bioimage datasets and the powerful symbolic capabilities of CNNs.
Conclusion
Deep learning methods enhance traditional machine learning tools and analysis strategies in computational biology, including regulatory genomics and image analysis. Ongoing improvements in software infrastructure are expected to broaden the application of deep learning to more biological problems.
Spotlight on a Practical AI Solution
Consider the AI Sales Bot from itinai.com/aisalesbot designed to automate customer engagement 24/7 and manage interactions across all customer journey stages.