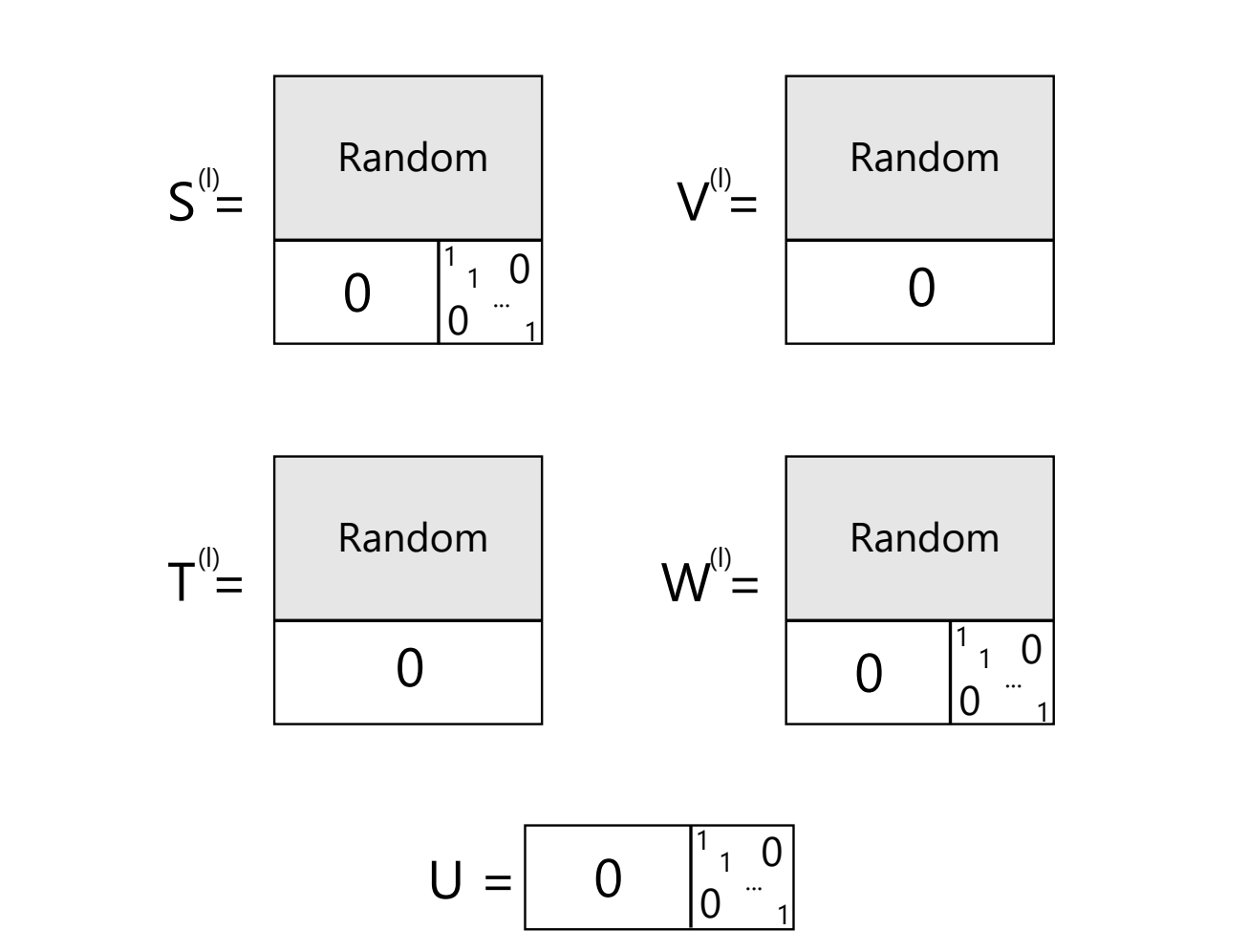
Practical Solutions and Value of Training-Free Graph Neural Networks (TFGNNs) with Labels as Features (LaF)
Graph Neural Networks (GNNs) Applications
Advanced Machine Learning models, especially Graph Neural Networks (GNNs), are instrumental in applications such as recommender systems, question-answering, and chemical modeling. GNNs are effective in transductive node classification for tasks like social network analysis, e-commerce, and document classification.
Challenges and Varieties of GNNs
The high computational cost of GNNs, especially when dealing with large graphs like social networks or the World Wide Web, has been a significant challenge. Graph Convolutional Networks (GCNs) and Graph Attention Networks (GATs) are varieties of GNNs that have demonstrated exceptional effectiveness in transductive node classification.
Introduction of Training-Free Graph Neural Networks (TFGNNs)
TFGNNs have been introduced as a solution to the computational cost issue. By using the concept of “labels as features” (LaF), TFGNNs can produce informative node embeddings without extensive training, making them efficient and versatile for rapid deployment and low computational resource scenarios.
Experimental Findings and Superiority of TFGNNs
Experimental studies have consistently shown that TFGNNs outperform traditional GNNs in a training-free environment. TFGNNs converge faster, requiring fewer iterations to achieve optimal performance when optional training is used. These findings confirm the efficiency and superiority of TFGNNs compared to conventional models.
Recommendations for AI Adoption
For companies looking to evolve with AI, the recommendation is to leverage Training-Free Graph Neural Networks (TFGNNs) with Labels as Features (LaF) for Superior Transductive Learning. The practical steps include identifying automation opportunities, defining KPIs, selecting appropriate AI solutions, and implementing AI gradually.
Contact Information and Resources
For AI KPI management advice and continuous insights into leveraging AI, the company can be reached at hello@itinai.com. Additional resources and AI solutions can be explored on their Telegram channel t.me/itinainews or Twitter @itinaicom.