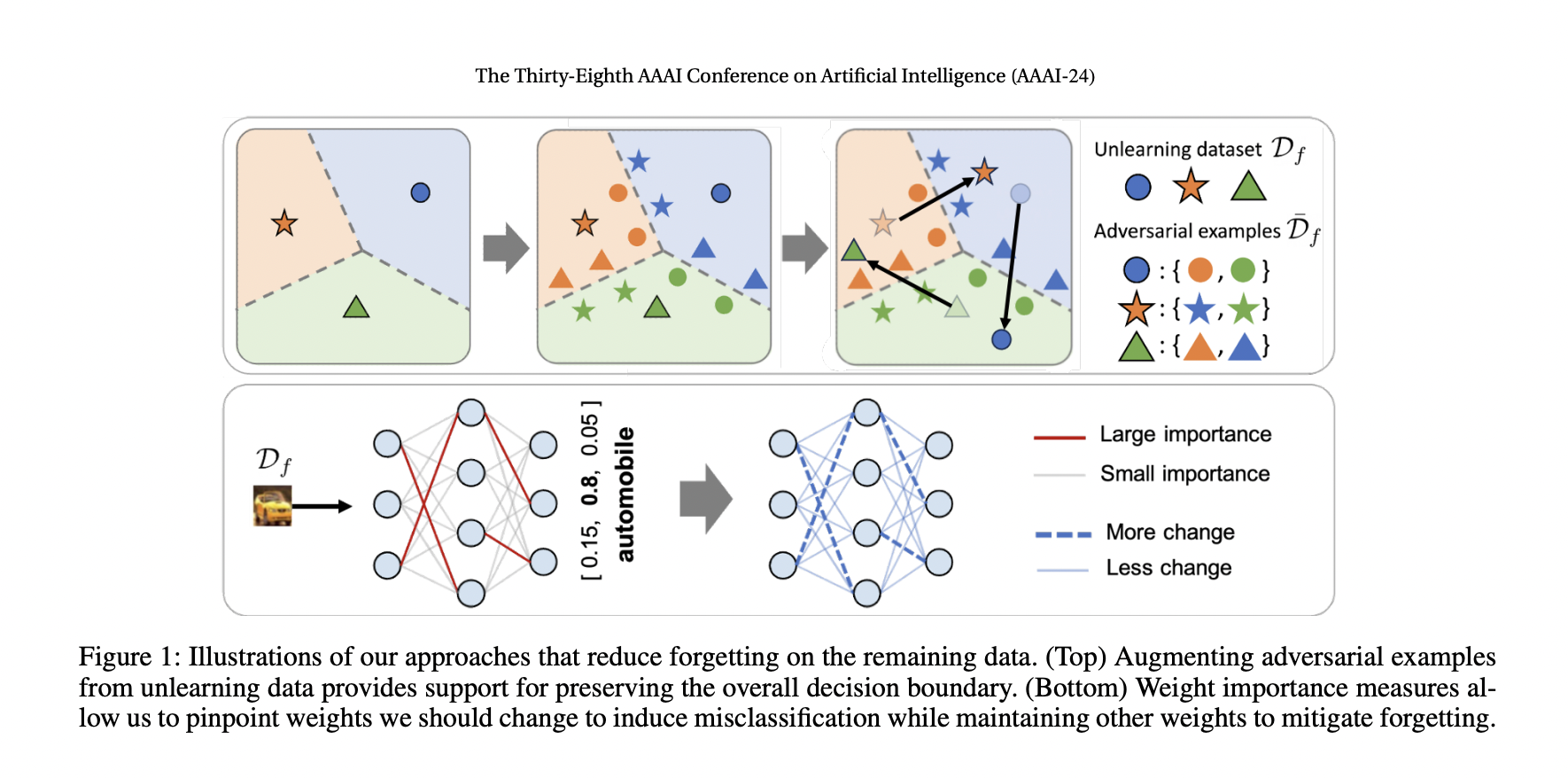
Machine Unlearning: Enhancing Resilience Against Risks and Vulnerabilities
Introduction
The increasing use of machine learning models in critical applications has raised concerns about their susceptibility to manipulation and exploitation. Techniques are urgently needed to allow models to unlearn specific data subsets, reducing the risk of unauthorized access or exploitation.
Challenges Addressed
Machine unlearning aims to modify pre-trained models to forget specific data subsets, enhancing their resilience against potential risks and vulnerabilities. Prior methods have proven ineffective against data leakage due to deep networks’ interpolation abilities.
Novel Approach
A recent publication introduced a novel approach to overcome limitations in existing methods. The proposed method introduces instance-wise unlearning and pursues a more robust objective of preventing information leakage by ensuring that all requested data for deletion are misclassified.
Proposed Framework
The proposed framework employs adversarial examples and weight’s importance measures for regularization. Adversarial examples help retain class-specific knowledge and decision boundaries, while weight importance prevents forgetting by prioritizing crucial parameters.
Experimental Validation
Experiments on various datasets demonstrated superior performance in preserving accuracy on remaining data and test data across different scenarios. The new method outperformed other techniques, showing the robustness and effectiveness of the new unlearning technique.
Practical AI Solutions
If you want to evolve your company with AI, staying competitive, consider strategies for instance-wise unlearning without retraining. Identify automation opportunities, define KPIs, select an AI solution, and implement gradually. Connect with us for AI KPI management advice and practical AI solutions.
Spotlight on a Practical AI Solution
Consider the AI Sales Bot designed to automate customer engagement 24/7 and manage interactions across all customer journey stages.