MIT engineers have found that deep generative models (DGMs) used in AI can mimic existing designs but struggle to generate innovative solutions to engineering problems. The study showed that when DGMs were designed with engineering objectives in mind, they produced more innovative and higher-performing designs. The researchers concluded that AI models need to go beyond statistical similarity and be trained explicitly on design tasks to achieve better results. The findings have implications for various engineering fields, as AI could help engineers create more innovative products if trained appropriately.
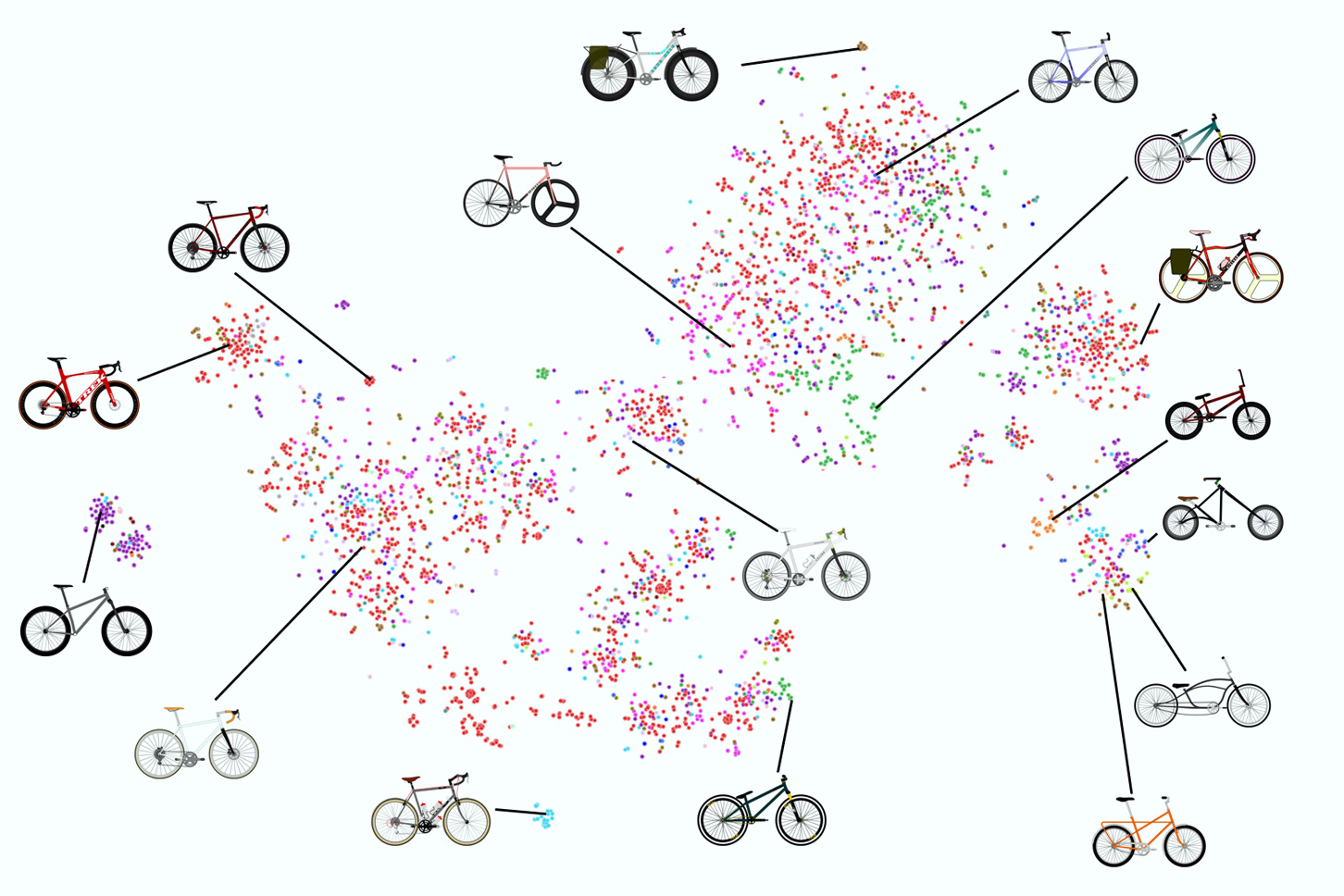
AI Solutions for Middle Managers: Redefining Engineering Design with Generative AI
Deep generative models (DGMs), like ChatGPT, have proven to be powerful tools for generating content that resembles existing works. However, when it comes to engineering tasks, similarity alone is not enough for true innovation. MIT engineers have highlighted the flaws of DGMs in their study, emphasizing the need to go beyond statistical similarity to generate novel ideas and designs.
The Pitfalls of Similarity-Focused AI Models
In their study, the researchers focused on engineering design problems, specifically bicycle frame design. They found that DGMs trained solely on statistical similarity ended up generating frames that mimicked existing designs but lacked in engineering performance and requirements.
However, when the researchers designed DGMs with engineering-focused objectives, the models produced more innovative and higher-performing frames. This demonstrates that AI models can be effective design “co-pilots” when carefully planned with task-appropriate metrics.
Going Beyond Statistical Similarity
The researchers explain that DGMs are powerful learners that can process vast amounts of data. However, they often generate designs similar to the dataset without considering specific design requirements. To illustrate this, the team used the example of bicycle frame design, where even small differences in dimensions can significantly impact performance.
They tested different DGMs on the task of generating new bike frames. A conventional DGM produced designs that resembled existing frames but lacked improvement in performance. However, DGMs specifically designed for engineering tasks generated designs that were not only realistic but also lighter, stronger, and physically feasible.
Practical Solutions for AI Implementation
If you want to evolve your company with AI and stay competitive, consider the following practical solutions:
- Identify Automation Opportunities: Locate key customer interaction points that can benefit from AI.
- Define KPIs: Ensure your AI endeavors have measurable impacts on business outcomes.
- Select an AI Solution: Choose tools that align with your needs and provide customization.
- Implement Gradually: Start with a pilot, gather data, and expand AI usage judiciously.
For AI KPI management advice and continuous insights into leveraging AI, connect with us at hello@itinai.com. Stay tuned on our Telegram channel t.me/itinainews or follow us on Twitter @itinaicom.
Spotlight on a Practical AI Solution: AI Sales Bot
Consider the AI Sales Bot from itinai.com/aisalesbot. This solution is designed to automate customer engagement 24/7 and manage interactions across all customer journey stages. Discover how AI can redefine your sales processes and customer engagement by exploring solutions at itinai.com.