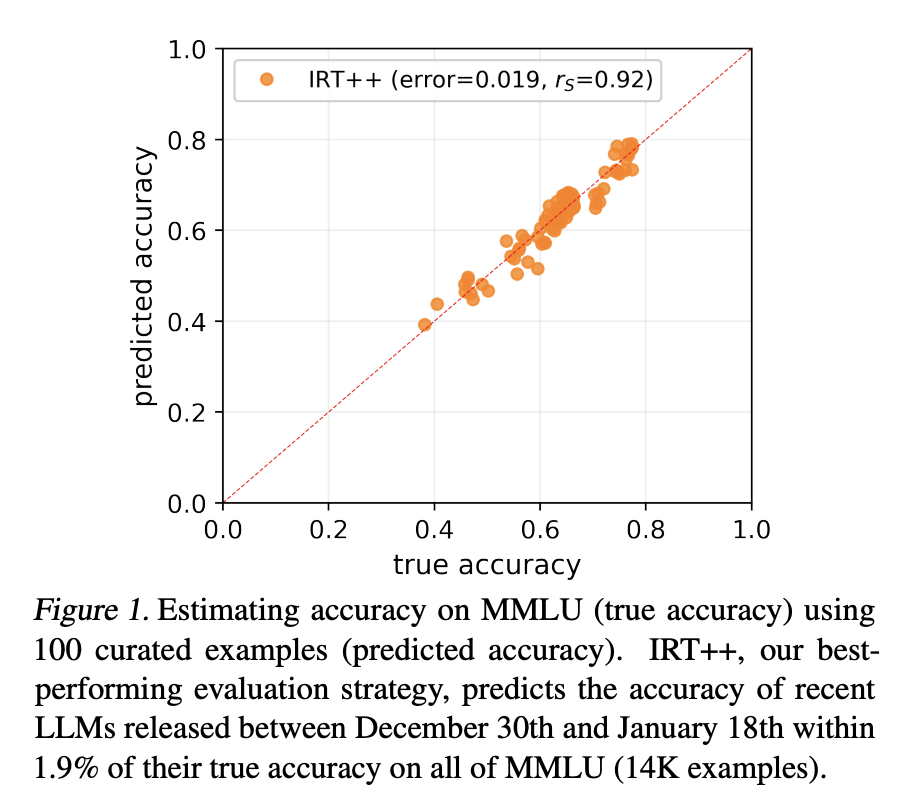
tinyBenchmarks: Revolutionizing LLM Evaluation with 100-Example Curated Sets
Practical Solutions and Value
Large language models (LLMs) are transforming NLP, but evaluating their performance has been costly and resource-intensive. tinyBenchmarks addresses this challenge by reducing the number of examples needed for accurate performance estimation, cutting costs by over 98% while maintaining high accuracy.
Research and Development
The research team from the University of Michigan, the University of Pompeu Fabra, IBM Research, MIT, and the MIT-IBM Watson AI Lab introduced tinyBenchmarks. These smaller versions of popular benchmarks aim to provide reliable performance estimates using fewer examples.
Methodology
The researchers used stratified random sampling and clustering based on model confidence to curate robust evaluation sets. They applied item response theory (IRT) to measure the latent abilities required to respond to benchmark examples, resulting in accurate and resource-efficient evaluation.
Validation and Availability
The performance of tinyBenchmarks was extensively validated and publicly released, demonstrating their reliability and efficiency. Other researchers and practitioners can benefit from these tools and datasets, allowing for continuous improvement in NLP technologies.
Practical Implementation
Companies can utilize tinyBenchmarks to evolve with AI, reducing costs and maintaining high accuracy in LLM evaluation. AI can redefine work processes, identify automation opportunities, and provide measurable impacts on business outcomes.
Further Information
For more details, check out the Paper, GitHub, HF Models, and Colab Notebook. For AI KPI management advice and continuous insights into leveraging AI, connect with us at hello@itinai.com or stay tuned on our Telegram t.me/itinainews or Twitter @itinaicom.
Relevant Resources
Find Upcoming AI Webinars at here. Discover how AI can redefine sales processes and customer engagement at itinai.com.