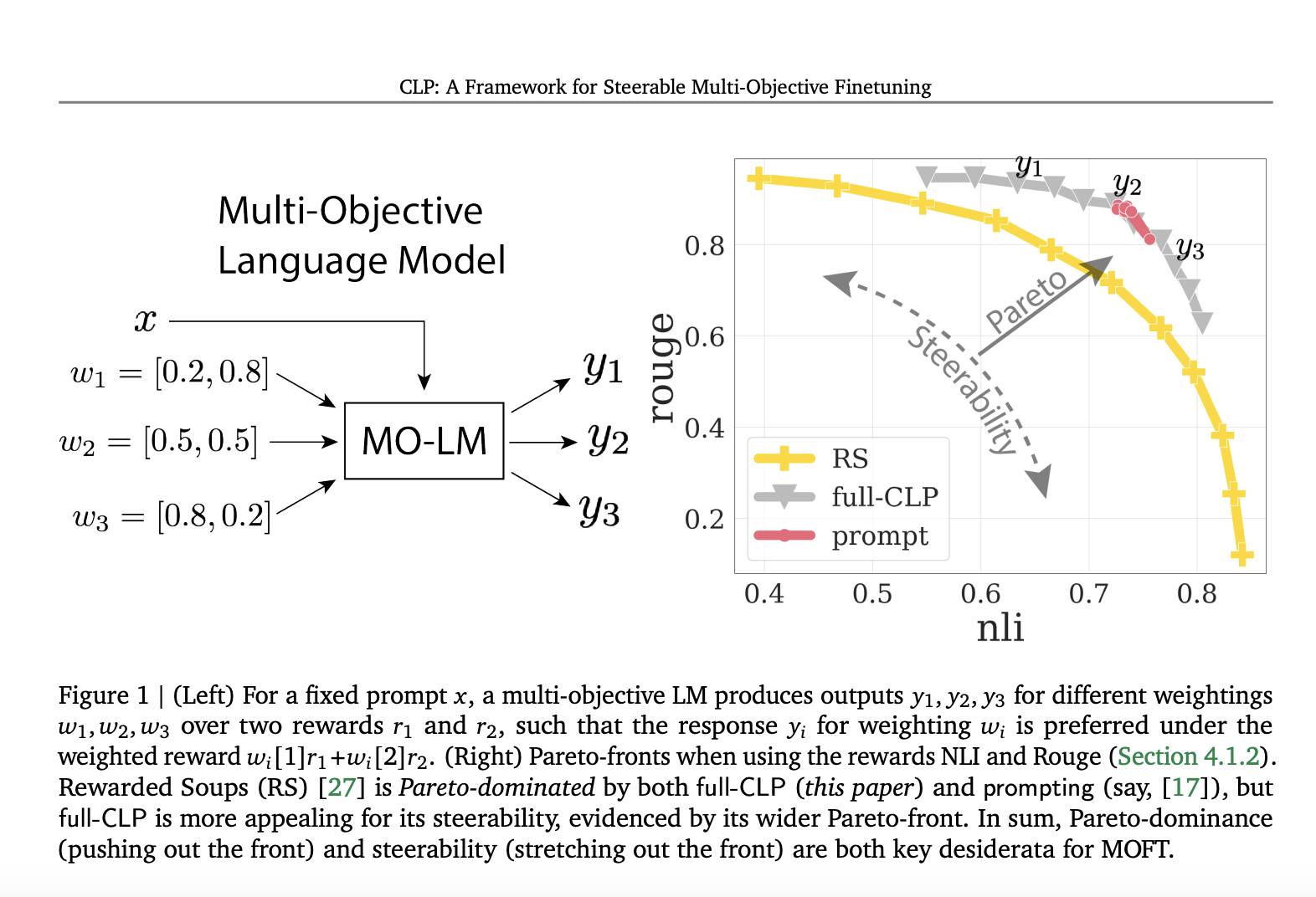
Reinforcement Learning for Language Models
Practical Solutions and Value
Multi-Objective Finetuning (MOFT)
MOFT is crucial for training language models (LMs) to behave in specific ways and follow human etiquette. It addresses the limitations of single-objective finetuning (SOFT) by allowing LMs to adapt to various human preferences and uses.
Approaches to MOFT
Two main techniques for multi-reward alignment are prompt-based and parameter-based conditioning. Prompt-based methods involve custom prompts to personalize LMs based on reward weightings, while parameter-based methods use parameter-space conditioning and multi-task training.
Conditional Language Policy (CLP)
Google’s CLP framework is more adaptable and generates better responses than existing baselines. It offers a flexible approach for finetuning LMs on multiple objectives, creating adaptable models that can balance different individual rewards efficiently.
AI Implementation
Identify Automation Opportunities, Define KPIs, Select an AI Solution, and Implement Gradually.
AI KPI Management Advice
Connect with us at hello@itinai.com for AI KPI management advice.
Continuous Insights
Stay tuned on our Telegram t.me/itinainews or Twitter @itinaicom for continuous insights into leveraging AI.