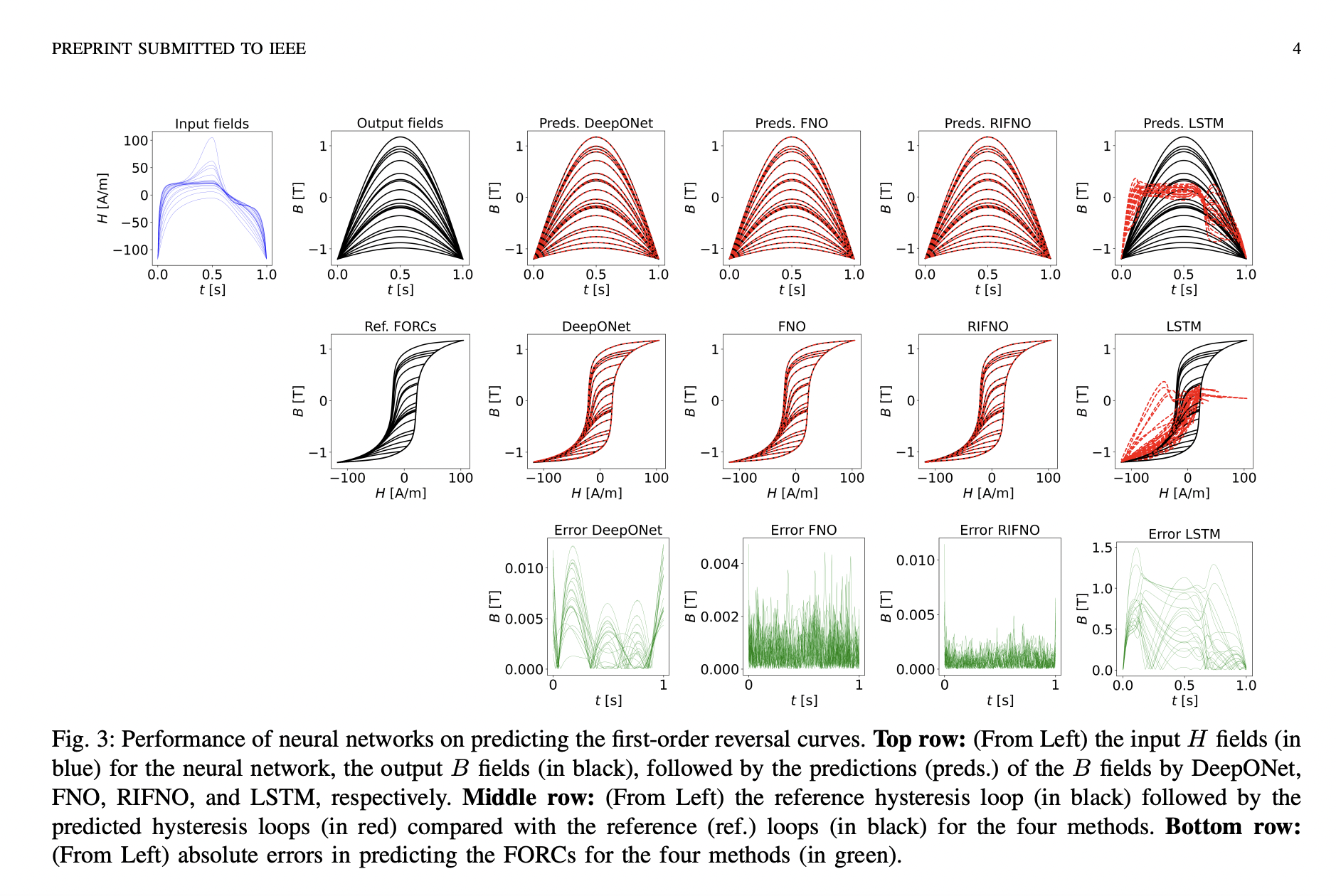
Practical Solutions for Modeling Magnetic Hysteresis
Challenges in AI for Magnetic Devices
Accurately modeling magnetic hysteresis is crucial for optimizing the performance of electric machines and actuators. Traditional methods struggle to generalize to novel magnetic fields, limiting their effectiveness in real-world applications.
Current Methods and Limitations
Traditional neural networks like RNNs, LSTMs, and GRUs struggle to generalize to novel magnetic fields, limiting their accuracy for real-world applications.
Proposed Neural Operators
Neural operators like DeepONet and FNO offer a more efficient and accurate solution compared to existing methods by approximating the underlying operator that maps magnetic fields, allowing for generalization to novel magnetic fields.
Training and Evaluation
The proposed method involves training neural operators on datasets generated using a Preisach-based model of the material NO27-1450H. The performance was evaluated using error metrics, showing superior accuracy and generalization capability compared to traditional recurrent architectures.
Conclusion and Value
The proposed methods, DeepONet and FNO, along with the rate-independent RIFNO, demonstrate superior accuracy and generalization capability, advancing the field of AI by developing efficient and accurate models for magnetic materials.
AI Solutions for Business Transformation
Unlocking AI’s Potential for Business
Identify Automation Opportunities, Define KPIs, Select an AI Solution, and Implement Gradually to evolve your company with AI and stay competitive.
AI KPI Management and Insights
Connect with us at hello@itinai.com for AI KPI management advice and stay tuned on our Telegram t.me/itinainews or Twitter @itinaicom for continuous insights into leveraging AI.
AI for Sales Processes and Customer Engagement
Discover how AI can redefine your sales processes and customer engagement. Explore solutions at itinai.com.