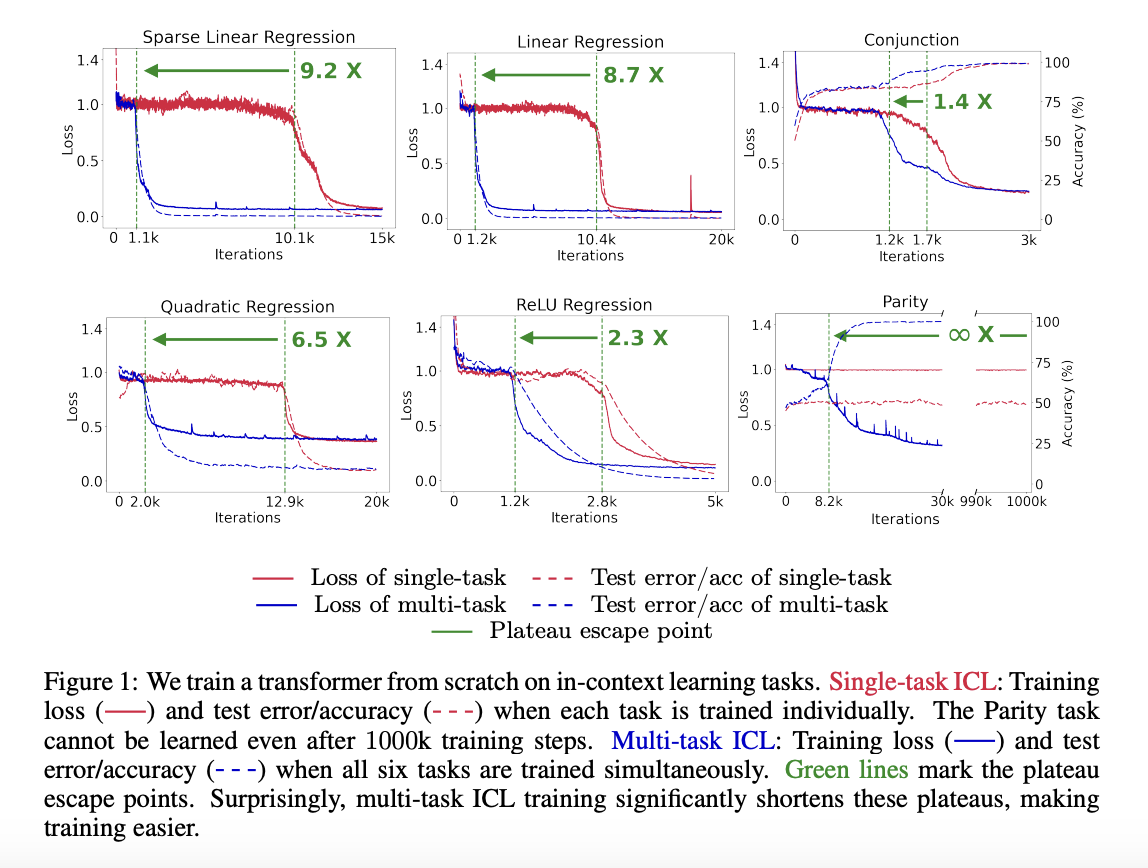
Understanding In-Context Learning (ICL)
In-Context Learning (ICL) is a key feature of advanced language models. It enables these models to answer questions based on examples provided without specific instructions. By showing a few examples, the model learns to apply this knowledge to new queries that follow the same pattern. This ability highlights how well the model can grasp the underlying logic of the data it processes.
Research Insights on ICL
Researchers have simplified models to explore how ICL works. They focus on the essential elements that aid this learning process. During their studies, they discovered a phenomenon called long loss plateaus, where the model shows minimal improvement for a long time, indicating difficulty in understanding the task. However, after this period, the model’s learning can suddenly accelerate, showing a breakthrough in understanding.
Impact of Task Diversity on Learning
Recent findings suggest that training models on multiple ICL tasks simultaneously can reduce the duration of these loss plateaus. This means that models can learn various tasks more effectively when trained together rather than separately. Surprisingly, instead of complicating the learning process, the variety of tasks actually speeds up learning and overall growth.
Implications for Large-Scale Language Models
This research has significant implications for training large language models. It suggests that the diversity of training data is as crucial as the amount of data. By exposing models to varied tasks, they can optimize their learning by recognizing shared patterns across different contexts. This diversity can help models progress through challenging learning stages more quickly and develop a deeper understanding sooner.
Challenging Conventional Wisdom
In conclusion, this study challenges the traditional belief that task complexity slows down learning speed. It shows that, in some cases, greater complexity can actually facilitate mastering each task. This offers a new perspective on why large-scale language models perform exceptionally well when trained on diverse datasets.
Get Involved!
Check out the original research paper for more insights. Follow us on Twitter, join our Telegram Channel, and connect with our LinkedIn Group. If you appreciate our work, subscribe to our newsletter and join our 50k+ ML SubReddit.
Upcoming Live Webinar
Upcoming Live Webinar- Oct 29, 2024: Discover the Best Platform for Serving Fine-Tuned Models: Predibase Inference Engine.
Transform Your Business with AI
If you want to enhance your company with AI and stay competitive, consider the following practical steps:
- Identify Automation Opportunities: Find key customer interaction points that can benefit from AI.
- Define KPIs: Ensure your AI initiatives have measurable impacts on business outcomes.
- Select an AI Solution: Choose tools that fit your needs and allow for customization.
- Implement Gradually: Start with a pilot project, gather data, and expand AI usage wisely.
For advice on AI KPI management, contact us at hello@itinai.com. Stay updated on leveraging AI by following us on Telegram at t.me/itinainews or on Twitter at @itinaicom.
Explore AI Solutions
Discover how AI can transform your sales processes and enhance customer engagement. Explore our solutions at itinai.com.